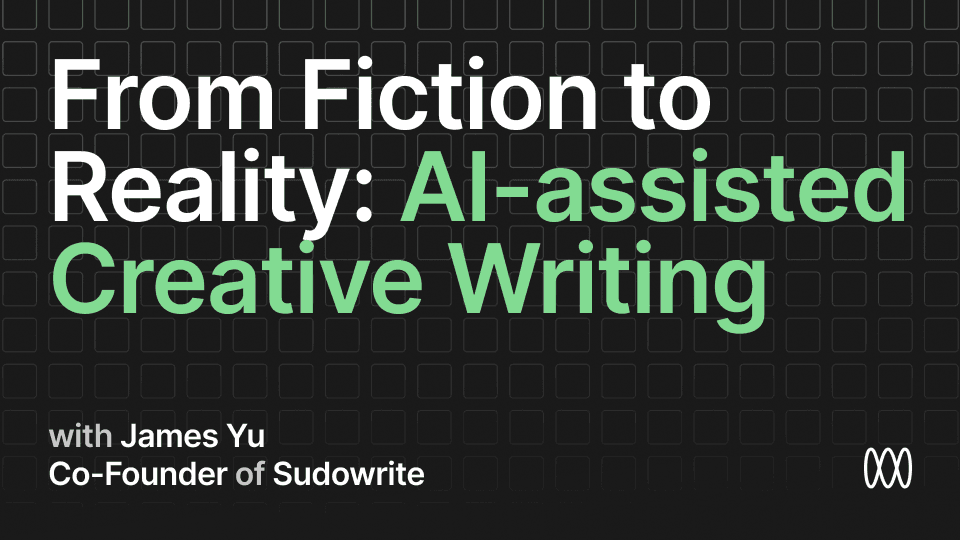
From Fiction to Reality: AI-assisted Creative Writing
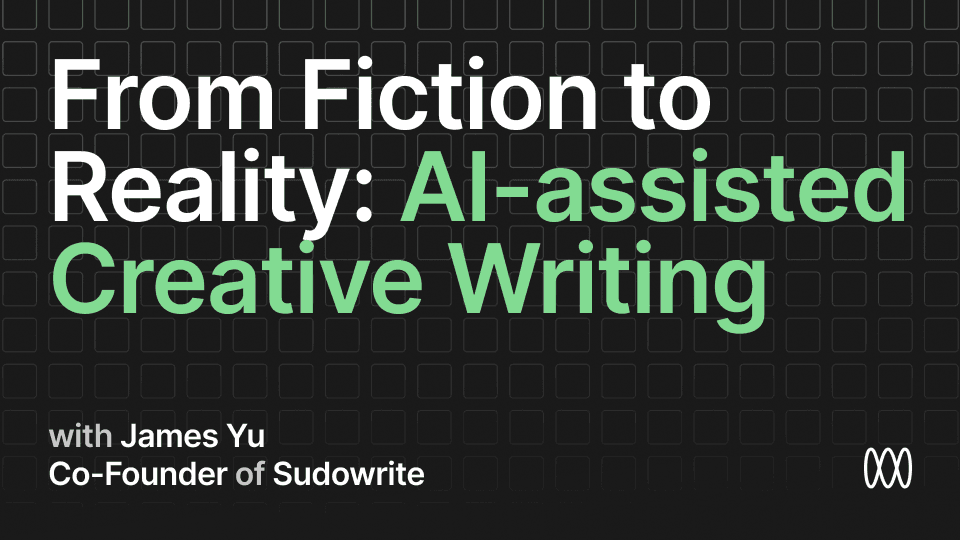
In this episode, we dive deep into the world of AI-assisted creative writing with James Yu, founder of Sudowrite. James shares the journey of building an AI assistant for novelists, helping writers develop ideas, manage complex storylines, and avoid clichés. James gets into the backlash the company faced when they first released Story Engine and how they're working to build a community of users.
Subscribe to Humanloop’s new podcast, High Agency, on YouTube, Spotify, or Apple Podcasts
Takeaways from the episode
- Domain expertise is crucial for AI product development
James mentions that half of Sudowrite's engineers have English majors or are avid readers and writers. "I think domain expertise is what is necessary to bring something from a cool Twitter demo into something relevant."
- Embrace the "wormwhole" concept for creative AI
Framing AI suggestions as "alternate universe" ideas helps users accept and use them creatively.
- Integrate AI into familiar workflows
Sudowrite incorporated AI features into interfaces like Microsoft Word writers were already comfortable with.
- Human remains in the driver's seat
"Even today, we still operate a lot on this card methodology, where we are showing you an array of options, and it's all about control—giving the author control of how they want to use that content."
Transcript
Chapters
00:00 - Introduction and Background of Sudowrite
02:26 - The Early Days: Concept, Skepticism, and User Adoption
05:20 - Sudowrite's Interface, Features, and User Base
10:23 - Developing and Iterating Features in Sudowrite
17:29 - The Evolution of Story Bible and Writing Assistance
24:27 - Challenges in Maintaining Coherence and AI-Assisted Writing
29:12 - Evaluating AI Features and the Role of Prompt Engineering
33:35 - Handling Tropes, Clichés, and Fine-Tuning for Author Voice
40:43 - The Controversy and Future of AI in Creative Work
51:37 - Predictions for AI in the Next Five Years
49:54 - Closing Thoughts
Podcast:
[00:00:00] James Yu: I think that even though Da Vinci could go off the rails, we leaned into that as a strong suit because hallucinations in that world, in fiction, can be good. We actually couched it as the wormhole feature because I think domain expertise is what is necessary to bring something from a cool Twitter demo into something relevant. So, I think it is a big shift, and I understand the kind of fear that is around there, like, "Is it going to take my job? Is it going to be trained on my works?" I do believe that authors should get compensation.
[00:00:33] Raza Habib: This is High Agency, the podcast for AI builders. I'm Raza Habib, and I’m really excited today to be chatting with James Yu, who's the founder of Sudowrite and also a serial entrepreneur, having previously founded and sold Pulse and been an engineer. I've actually been looking forward to chatting with James for a while now because I think Sudowrite is a pretty unusual AI company. They’ve built a writing assistant for creative writing and for fiction authors. And also, because I think James is unusually thoughtful in how to build with LLMs, and as I think, like, discovered a lot of the best practices for himself. James, it’s a pleasure to have you on the show.
[00:01:17] James Yu: Yeah, happy to be here and talk with you, Raza.
[00:01:19] Raza Habib: Fantastic, James. To start with, for our audience, who are mostly people who are themselves trying to build AI products, can you just describe a little bit of what Sudowrite is and the current stage of the company?
[00:01:23] James Yu: Yeah, so Sudowrite is an AI assistant for novelists, and long-form storytellers is our sweet spot. How I usually describe this to people is that most writers, 99.99% of writers, do not have a writer's room like in Hollywood. That is a very rare thing, and so you could kind of think about Sudowrite as that writer's room for you as a creative writer. So, we're very focused on using AI as a point of inspiration to help get you unblocked when you're writing a story, specifically.
[00:01:58] Raza Habib: So, I remember when I first heard about this idea, like, the first time you and I interacted, which I guess must have been, like, 2022 now, so some time ago, and I was, like, immediately skeptical that this is something that writers would want. It was, like, a really counterintuitive product to me. Like, who are the people who are using this and what has their response been? You know, like, why was my intuition wrong? Like, I thought that people who are creative writers would kind of balk at the idea of an AI writing for them or helping them.
[00:02:26] James Yu: Yeah, it's been interesting. Well, I mean, I think one aspect is that Sudowrite was really born out of our own needs. So, my co-founder, Amit, and I, we were actually part of a writing group called Sudowriters. So, that was the origin of the name, and we named it that because we were sort of tech-adjacent folks who were trying to break into fiction, really critiquing each other’s stories, getting different ideas. So, when GPT-3 came out, I started playing with it. It really helped unblock me specifically. So, it really came from that lived experience, seeing how an AI could reflect back on my story. And you know, as a story writer, you're going to get blocked. You're going to hit these roadblocks where you don't know how to finish a story. You need some inspiration. You go for a walk, talk to your friends, and I found, I personally found, that AI was another tool in that toolset to be able to help you get unblocked. So, I sort of saw it as a pair with the craft of fiction, which you still need. The craft of fiction, it's not, you know, it's not magic. You still have to put in the work, but it is another voice that you can consult with. And yeah, how this sort of spread was really word of mouth, right? In the beginning years, we didn't really see this as a sort of high-growth startup. In that way, we really saw it as a toy for ourselves and just talked to other writers' groups who were receptive to the idea of including AI, and it sort of just grew from there. And even in the beginning, we didn’t charge for it for a while, or maybe charged like five bucks a month. But what we really found is that there are certain classes of writers who are interested in using language models in that process. That being said, it's pretty nascent. I think that it is maybe more common to see AI being employed in emails or other enterprise kinds of applications. I think it's still relatively new to the literary world and screenwriting world and sort of creative writing. I do slowly see that changing in the same way that people are using, you know, things like Grammarly, or things like grammar check, spell check, and other kinds of tools. So, I do see this as a long-term journey for us.
[00:04:53] Raza Habib: It’s part of why I wanted to speak to you because I think so many of the initial applications of AI have been very useful but practical, mundane, focused on automating mundane tasks. And this is one of the few examples I’ve seen of a product that really is enhancing people's, like, normal creative abilities. You know, it’s something that I didn’t even think was possible. I saw you guys do it. Do you mind sharing a little bit about the numbers? Like, how many people are using this now? Like, what’s the scale of Sudowrite today?
[00:05:20] James Yu: Yeah, sure thing. So, we have over 15,000 paying members. I would say the vast majority of them are novelists. And these kinds of novelists range—some of them are self-published, some of them have more of a traditional path with agents, some of them are writing multiple books a year, and some of them are writing, you know, maybe one book every four years. Yeah, it's been kind of a journey, really word of mouth over the past four years. So, we’ve been around since 2020 from that seed, but it's definitely now to a point where it is a sustainable business for us so that we can continue to focus on growing to more novelists as well. Screenwriters are sort of a secondary audience that we do see a lot of growth in as well.
[00:06:06] Raza Habib: You know, we're an audio podcast. Can you describe the interface of Sudowrite for someone who hasn’t seen it? I actually think it’s pretty innovative how you guys have set it up. So, you know, if I open up the Sudowrite editor, like, what am I seeing on my screen?
[00:06:16] James Yu: So, basically, you see a text editing program, just like Google Docs. So, that is really the primal interface. We are not a chat; it's not like a chat program, like ChatGPT. You are working with your manuscript, but then at the top bar, you have various AI functions. Some could help you ideate how you would continue the story. Others could help you try to describe things in your story with more lush language or rewrite it. So that's the principal way that people interact with this. So, you’re writing, and you occasionally use some of these functions to help you when you get stuck. But we also have something called Story Bible, and this is something we introduced last year, where it is really a database, essentially, for a story writer to keep track of all the things that are important to your novel in a way that can inform our AI about the various elements, like characters or elements in your world-building, like cities, towns. So, these can be kept up to date by the author, or it can also be analyzed as well when you import your work-in-progress novel. So, we see this as a very crucial piece of the puzzle because it's sort of the liaison between the unstructured prose of your novel, which could be 100,000 words, and the structured ideas and elements that are in your story. It’s best to communicate the AI through this interface we call Story Bible.
[00:07:44] Raza Habib: There are almost like two different paradigms for interacting with the AI then in Sudowrite. So, one is this kind of AI, for want of a better word, co-pilot, which has become kind of the name for these things now, that's kind of sitting over your shoulder. It's watching as you write, and you can ask it to add color, to rewrite things, to extend stuff, to kind of help you as a critic. And then separately, there's this, like, separate, bigger piece, Story Bible, that is allowing me to build up a coherent long-term context of the characters and the background and the city. Is that a fair summary?
[00:08:14] James Yu: Yeah, that's a pretty fair summary. We do see Story Bible as the backbone for a lot of these functions. So, even when you're using, like, a small rewrite of this particular paragraph, we may be bringing in salient elements of StoryBible into the inference as well. So in that way, it is similar to RAG in that way. But, you know, Story Bible is kind of like RAG. We are doing also RAG across kind of the prose, but we also see Story Bible as part of that system. So underneath the hood, we developed something called a saliency engine, which allows us to figure out which parts are salient or not. That being said, we do tell the authors to meet us in the middle, because even with today's advanced language models, writing a novel is still quite an undertaking and a very, very intense process that humans should definitely be in the middle of. So, for example, an affordance that we have in Story Bible is the ability to say, always show this element or hide this element from the AI for now. This is useful, for example, if you have secrets in your story that shouldn't show up until later. We would love to have people do those kinds of things automatically, but we're not quite there yet, and we know that. So, that's something that we inform our community about, like how to tweak these knobs and things like that. So in that way, I almost think of Story Bible paired with the inference in our system like a music sequencer. Maybe you need to mute these drums now, or maybe you need to enhance, you know, the wind section over here. Why not be able to also think about that when you're writing a story or a novel that progresses through time? Because that is the other crucial element. There’s a very big time aspect to writing a story. For now, like, yeah, the user, the writer, is meeting us in the middle. I suspect, you know, as time goes on, and these language models get even better, they won't necessarily have to meet us in the middle. But, you know, one thing is our writers do enjoy writing, and they are using this as an instrument that is highly adaptive to their style. That's why a lot of people choose Sudowrite today.
[00:10:23] Raza Habib: Hopefully, we've given the listeners now a fair picture of what Sudowrite is, how many people are using it, and the fact that it's a really powerful creative tool. So, what I'd love to turn our attention to for a little bit is actually, like, how you build it, because I think you guys have a particularly interesting take to this, and I want to dig in deep on, like, how you guys do prompt engineering and how you figure things out. But also, given, you know, the length of a novel and the coherence that needs to be maintained, and the Story Bible, like, it's a complicated thing to figure out: how do I actually use LLMs to help someone write a novel? So, can we maybe start off with where you started? Like, what were the earliest features that you built into Sudowrite? And then work our way up to how you get the full complexity of Story Bible to work?
[00:11:11] James Yu: Yeah, so when we started in 2020, this was literally the first edition of GPT-3. It was the Da Vinci model, which I still have soft spots for, because I think that even though Da Vinci could go off the rails, we leaned into that as a strong suit for us because hallucinations in that world—in fiction—can be good. What we developed early on was literally just giving authors this kind of completion technology, right? And we actually couched it as the wormhole feature. So, how wormhole, how we communicated it to the author is that, “Hey, when you hit this wormhole button and it gives you suggestions for what might come next in the paragraph, this is other copies of you in other universes that are making different choices in the multiverse. Take it or leave it. Maybe this suggestion from multiverse 2763 is a great option. Maybe it sucks, right?” So, it was very crucial for us to set that expectation because even though GPT-3 was a big jump in quality versus GPT-2, you still had to take things with a grain of salt. But even at that very nascent state, we found that it was a killer feature. Right when you were stuck, you needed different voices and different kinds of ideas on the table. So that was really the first version of Sudowrite—our very baby step.
[00:12:37] Raza Habib: That version, if I imagine how it's working, is really just passing the LLM the previous paragraph of text and saying, "What comes next?" Basically, yes? So, MVP Sudowrite is kind of nicely wrapped GPT-3, and then what came next?
[00:12:53] James Yu: Yeah, so we, you know, as the language models became more powerful and had longer context windows, and became more coherent, we added a lot of features that we felt could be delivered with quality, right? That the expectation is met. So, for example, the next feature we had was: "Oh, highlight a word, let’s say in the scene, like highlight a character, and say 'describe them,' you know, and we describe them using the five senses—taste, smell." So, a lot of these things are about trying to get a very evocative scene, which is one of the pillars of writing great prose. We found even Da Vinci was pretty good at doing that, giving you suggestions for different world-building elements like characters. And once again, all of these were in the very, like, card-like interface, which gives you many different options, right? Because at that time, it still goes back to hallucinations and going off the rails. Maybe, let’s say, two out of the five cards would go off the rails.
[00:13:49] Raza Habib: When you say card-like interface, if I recall correctly, the way this works is like, I click "rewrite" or I click "describe," and then there’s a kind of sidebar where I get multiple generations, and I can choose to add them into the editor and they’re kind of appearing on the side?
[00:14:03] James Yu: Yep, so it would not auto-insert. That was actually one of the pillars of early Sudowrite as well. We didn’t feel like the language models had the confidence for people to just be like, “Okay, this is going to be great prose, and it’s going to just insert into your manuscript.” But we also saw, as part of the artistic process, that you are looking at this as a pastiche on the right bar—different ideas and options—and then also felt like lower stakes because it’s not being inserted directly. It’s like, “Take it or leave it” again, or ignore those cards very easily. And if some of the options were bad, well, you know, a lot of people see bad options and say, “Oh, you know what? That makes me think of an even better option,” and they never even use the content in those cards, right? So, this kind of negative space aspect to language models as well, because bad ideas are sometimes good in a way that makes your own brain think of a better idea. And so we leaned into that for a while. Even today, we still operate a lot on this card methodology, where we are showing you an array of options, and it’s all about control—giving the author control of how they want to use that content.
[00:15:13] Raza Habib: And something that I recall from when you showed this when you were first building it is that you were very deliberate and considered in figuring out how to make those features good. Do you mind explaining a little bit of your process for how you guys develop the prompts and how you sort of take the 1.0 version of the feature and actually get it to the point where 15,000 writers are wanting to use it?
[00:15:33] James Yu: Yeah, so that’s evolved a lot over the past four years. I would say in the very beginning, it was really human evaluation from me, my co-founder, our team, and also our community. And, you know, that got us actually pretty far in doing that in the early days. But then we also added this aspect of looking at positive actions taken after a card was produced for the user. So, for example, if they decide to insert that card or they decide to copy and paste some content from that card, those are positive intents by the user, and we would take that into account when, let’s say, we’re improving the wormhole button or the describe button. Maybe we improve the prompt somehow. Maybe we inject other, you know, context stuffing, or some other technique. We tend to use that as more like downside protection. I would say, like, “It’s not colossally bad; like, this didn’t reduce the quality a lot.” In terms of the upside, we definitely do quite a mix of qualitative and quantitative measurements. Today, with 15,000 members, we lean also heavily on the community for this. We beta test a lot of our features and measure things like, "Oh, are they using it more often?" So, retentive kinds of measurements—are they using it as a replacement for the older version of the function more often? Sometimes we will show them side by side. So, various different techniques to really get at that positive intent from users, to try to get a sense of whether people are actually using this feature. One thing I’ve discovered is that something that goes viral on Twitter in terms of some AI demo doesn’t necessarilymean that people are going to use it day in and day out for their workflow. So we really want to see that kind of retentive use of our function.
[00:17:29] Raza Habib: So is your workflow quite experimental? Are you putting out a lot more features than stick around? Like, what's the process?
[00:17:35] James Yu: So it varies. For example, Story Bible really was an experiment. I built that as a prototype of what could be possible with GPT-4, essentially. And it was really that step function where we saw that, wow, it’s much more coherent now. It is able to remember things better, take into account the context. So that was a huge unlock. But even that took many months of experimenting and tinkering, and a long beta process where we even built that as a separate surface on our app, separate from the main editor. So I would say that was a very intensive experimental phase that lasted months.
[00:18:15] Raza Habib: And during that experimental phase, what are you iterating on? What are the variables or the levers you're pulling to try and make it better?
[00:18:22] James Yu: Yeah, so a lot of that was tweaking the prompts, tweaking the temperature, the various parameters. But that’s also in concert with looking at how users actually use the system, right? To give a bit more context, the Story Bible workflow is very different than, for example, just "describe." "Describe" is like you highlight a piece of text, and then it gives you some options. Story Bible, meanwhile, starts with a brain dump of what your story could be, and it helps you flesh out a synopsis from that. And from a synopsis, it helps you flesh out an outline. You can edit that, you can rewrite it, and from there, you can start fleshing out each chapter of your book. So this was not just one single prompt, but I would say it’s a prompt system, and there’s also almost like a model system as well, because some of these were multi-chained prompts that involved different models. I think at the time, mostly GPT-3, but today we also use lots of open-source and Anthropic models as well. So the complexity was 10x, right? So in that world, really, it was both an educational problem for the community, like how do we educate people to use the system in an effective way, while also measuring, like, “Oh, are people thumbs-upping the output? Are people keeping the output and being like, 'Okay, I’m going to copy-paste this into my manuscript now because it was really good?’” Only one in, you know, one. What is the quality bar for that? Well, some of that was quantitative. It was like, "Okay, is the thumbs-up rate good or not?" But a lot of that was also just a gut feel, seeing the excitement in Discord, seeing how people were now telling their friends about it, like, “You should get into this beta program for Story Bible." “Oh, it’s a limited beta. I wish you guys could expand it even more.” Right? So we also do a big community temperature check on a lot of the features that we’re building. If there’s no excitement around it, usually it's kind of a negative signal. And if people are not using it, that's also a negative signal as well. So we take a lot of these kinds of signals coming in. Story Bible is the most involved. For our smaller functions that we iterate on, maybe it is... we just take some quantitative signals from an A/B test, and we use a tool called GrowthBook for doing some of the testing there. Of course, we also use HumanLoop as well for the evals and also for iterating prompts. So, we're not very wedded to one kind of technique, because I think each and every function is used differently, and different judgment calls are needed for each of them.
[00:20:57] Raza Habib: Can you tell us how Story Bible works? You know, the original features like rewrite, describe, etc., I kind of intuitively understand how one might build that. But how do you even begin to go about building something like Story Bible that needs to help me write an entire novel? And also, when you launched it, the context windows were a lot shorter. So, to what extent are you using one context? Like, what techniques are you using? How do I make it work?
[00:21:17] James Yu: For Story Bible, I sort of sat down and thought about how I think about writing a long-form story. There are many ways into that, but one way into that is something called the snowflake method, which is, you start with broad strokes of the entire story that could be encapsulated in a very small amount of synopsis. Say it’s just a few paragraphs or so. From there, you expand each paragraph into, you know, a larger one-pager. From there, you expand each one-pager into a chapter. And if you keep doing this fractally, essentially, you would get a coherent story and a whole novel as well. So, this was a methodology, I believe, created by a software engineer who turned writer. He espoused the snowflake method. Now, Story Bible wasn’t precisely this method, but it was in the same thrust where I sat down and thought about, “Wait, what is the problem with the context window?” Because at the time, yeah, the context windows were small, and how could you possibly generate 100,000 words for a novel from that if your aim was to write a zero draft, for example, using a language model? Well, this sort of expansion technique with the human in the loop would allow the language model to be able to go much farther there. So, I basically built a prototype, which was one box, I think it was like six boxes next to each other, and it started out from the very high level, all the way down to each chapter. And then essentially, the user is working left to right and imbuing more and more detail and more and more length along the way. And so this was the crux. This allowed the language model to be able to work in sizable chunks that it could handle. And one crucial thing we ran into along the way was, going from a scene—a description of a scene, let’s say, for a chapter—and going from a paragraph, how do you have a language model write 4,000 words based on that small description? A junior writer, for example, a human junior writer, could probably do that pretty well with good literary knowledge. So, in cases like that, we added a step in the middle. For example, you can't just go from the scene description to the full chapter. You have to write scene beats. So, basically, an ordered list of step-by-step actions that happen in that scene. This was not actually intuitive to literary writers in the beginning, because now they think it’s just like, “Well, yeah, you just write the whole scene, right from top to bottom.” But we found that it was very effective to have the writer break down, “Okay, what are the beats of this scene? Give me 12 bullet points.” Then, we would have this chunking technique that would allow the AI to then be like, “Oh yeah, okay, I’m working on these two or three elements within this scene. I have the context of some of the previous ones, but I could just focus.” Right? So it’s really about having the human break down all the constituent parts of the novel-writing process into chunks that the AI could be able to handle.
[00:24:27] Raza Habib: And how do you ensure coherence across the book? Right? Like, presumably, a character gets mentioned in chapter one and they're doing something, and then in chapter 11, everything still needs to be consistent. That feels like it would be quite hard to do, maybe easier now with long context, but still non-trivial. How do you guys overcome that?
[00:24:45] James Yu: This is something we’re still trying to overcome. Okay, it is a tough problem. You know, as I said before, right now, we are really having the author meet us in the middle. So they are giving the context in Story Bible. Something that we’re actively working on right now is adding a timeline element to Story Bible. How I think about this problem is world modeling, essentially. If you were to do this full force, you would have a timeline going from the beginning of the novel all the way to the end, and then state variables for every single element, and obviously every character, but also like, where they are, scene settings, and once you have that model, you can imagine a system that would be able to provide whatever’s salient to that scene to be able to write it. So, J.K. Rowling actually is very famous for being this type of writer, in that she keeps a very detailed spreadsheet of all the characters in Harry Potter for every scene. What are the characters doing in that scene? But what are the characters that are off-scene doing as well? So, her mind kind of works in this way. So, I see Story Bible in this sort of what we’re trying to reach for, to be able to get to that state. Now, I think that to ask a human to write all of that is very onerous, and no one’s going to do that, except for maybe J.K. Rowling. So we’re currently employing things to be able to fill that out, right, to be able to essentially simulate this novel world for the purposes of writing this particular chapter or scene, or to make this description more evocative. We're not there yet. I mean, I think we're in the middle of that journey of essentially making this model that will help the AI understand the story better. Now, you also mentioned long context windows. Obviously, you could just put the entire novel into, you know, the long-context Gemini or the longer-context Anthropic stuff. Obviously, there are some cost concerns there, like, do you really want to pay seven bucks to help with this one paragraph? Maybe, maybe it’s a very crucial paragraph, but I think that might be the wrong tool. I think that might need to be paired with a system that understands logically what’s happening in this thing.
[00:27:33] Raza Habib: When you talk to the writers about their interaction with the system, when you're talking to your kind of users and customers, how do they describe their interaction with AI? Do they describe it as, like, being an assistant? Do they view it as a co-creator? Do they still view it as a tool? Like, what is the metaphor that they are using when they’re thinking about what Sudowrite is to them?
[00:27:33] James Yu: Yeah, that varies a lot. And the reason it varies is that it's still very nascent, and people are still trying to figure out how they’re interacting with these systems. So, I would say it’s all of the above, but there are definitely people who are like, “Oh yeah, I use it strategically for these kinds of places where I need help in my manuscript,” all the way up to people who are like, “Oh yeah, it's a co-creator. I have a particular template I use for my romance novel. My readers know what they want, and they actually know that AI is involved in helping craft this story. I have a particular outline template, and I use this to write my zero draft, and then I go in and edit everything.” So it really spans the gamut. There’s also a lot of people who just use it as a reflective tool. In the early days, we heard from users that this made them feel less lonely because, as you might know, novel writing is a pretty lonely affair for most people. Most novelists are lone wolves. Maybe they have a writing group, maybe not. I mean, I think that’s still almost a pretty privileged position to be in. And sometimes their partner won't even read their manuscript if they’re sick of it. So, we definitely heard reports that, like, “Oh, at least Sudowrite will read my stuff and also be positive and be like, ‘Here are some ways to be able to improve it.’” So, we definitely hear that. In some ways, we might also age into a little bit of a wellness kind of—or coach, writing coach, or AI writing editor. I hesitate to fully characterize it as such, because I think that, you know, ultimately, the human really is the editor. But we definitely have shades of that where they are anthropomorphizing the AI as being that co-creator.
[00:29:12] Raza Habib: I took a slight tangent. I want to close the lid on kind of the actual practical nuts and bolts of building this thing. One big question that has been on my mind is just, how do you do evaluation overall? Like, how are you measuring? You’ve mentioned hints of this around the place, but what’s the process for when you launch a new feature? Or, because it feels very subjective, feels like it’s hard to measure. Especially once you’re generating something the length of a novel, then actually even reading the output might be something that takes a lot of time, let alone generating multiple versions and scoring them in some way or trying to check for consistency. So, what is literally the nuts-and-bolts way that you guys measure performance today?
[00:29:49] James Yu: Yeah, so we do use some LLM evaluation, and those are mostly guardrails. For example, we have a coherency metric. So, in Story Bible, let’s say that you have these scene beats, and then you generate 400 words of prose from that. So, we have an evaluator, I think we’re using maybe GPT-4 or OpenAI, or maybe Haiku or something like that. And then we’re using that to basically inspect the output and be like, “Okay, did it actually hit those scene beats? Was that accurate in that sense?” So nothing about creativity; it’s more like guardrails of, yeah, “Is it following the instructions?” So, we have a whole bevy of these—about a dozen different metrics that we use. Another example is, "Are the characters that are mentioned in this prose actually the characters that were in Story Bible? And if so, are they accurately named? Are they doing anything that’s out of character with them?" All the way down to even lower-level things like, “Are there repeating paragraphs in this scene?” Because a lot of times, LLMs tend to repeat themselves internally. We call this the demon of repetition because it happens a lot. So, we have, like, “How do we vanquish repetition?” So we have a metric for that as well, trying not to repeat beats that we had already written for. So, we don't run these evals all the time. We will do them strategically when, for example, we’re developing a new function or we’re doing a vast improvement to an existing function as well. The other thing is just, yeah, human evaluations, and that’s through beta testing and getting their feedback and having them thumbs-up or thumbs-down. We will also look through those thumbs-downs, especially when we’re iterating on a function, and be like, "Okay, was this a colossal problem? Why was this bad?"
[00:31:41] Raza Habib: How often is the problem just the prompt itself? How much of the time can you improve things through prompting versus other interventions?
[00:31:49] James Yu: I would say the vast majority you can improve through prompting and/or deciding, “Hey, we’re not going to release this feature.” Right? We would just hold, where we're like, "This is not at the quality level that we feel would be useful on a daily basis." That being said, we are now also doing a bunch of fine-tuning experiments where we are fine-tuning based on the voice of the novelist, and that reduces the problem of tropiness or things that feel out of character. So, I think those problems of that nature, which are about tone and voice, are more suited to fine-tuning or LoRAs. That’s something we’re doing a bunch of research in.
[00:32:30] Raza Habib: The intuition that I have for this is that if it’s the kind of thing that’s very hard to tell, if it’s like a "show, don’t tell" type of attribute, then it’s probably better done through fine-tuning, right? Like, if you can’t articulate it in natural language, it’s going to be very hard to do it in a prompt. But if you can clearly explain it to another person, then I find prompting is often the way to go.
[00:32:51] James Yu: I think that’s a fairly accurate representation. And so, therefore, a lot of the problems are things that you can describe, right? And also, our users can describe pretty well. I think there’s one exception, which is tropiness in some ways because you can tell there are certain kinds of turns or phrases that are being used. Obviously, you can try to prompt against that, but we found that it’s not quite there.
[00:33:12] Raza Habib: Interesting thought. Do you guys do any analysis across your multiple writers? Like, is there anything that you're learning from different writing styles or improving the models in some way? Take tropiness as an example. Can you kind of do cliche detection? Almost like, "Hey, by the way, you’re writing in a style that’s really similar to everybody else." You could imagine that kind of feature. Do you have anything like that today?
[00:33:35] James Yu: We don’t have a feature for that specifically. We have features that try to coax cliches out of the models. For example, if you go to ChatGPT today and say, “Hey, write a small-town romance,” there’s a 99% chance that town will be set in a place called Willow Creek. We don’t know why it’s always Willow Creek. It’s like 90% of the time, it’s Willow Creek. If it’s a fantasy novel, it’s a town called Eldoria. We don’t know why these strings do appear in literature, but they’re not that notable. There are some novels, I believe, but yeah. So we have prompting—not specifically for Willow Creek—but we found that certain types of prompting will eject it, at least from this particular latent space. There’s still maybe a 30% chance it will still be Willow Creek. That’s why we always say, like, the solution to that kind of tropiness is, "Put in your original content." Please do not start from the standstill of, "Generate the entire novel from this one paragraph." It just doesn’t work very well. If you want to do that, I mean, sure, we’re not stopping you from doing that, but you’re probably going to get stuff that falls into the easy spaces—the easy tropes that GPT will just
[00:33:35] James Yu:
We don't have a feature for that. Specifically, we have features that try to coax clichés out of the models. For example, if you go to ChatGPT today and say, "Hey, write a small-town romance," 99% of the time, that town will be set in a place called Willow Creek. We don't know why it's always Willow Creek. It's like a 90% chance that it's Willow Creek, and if it's a fantasy novel, it's a town called Eldoria. We don't know why these names appear in literature, but they're not that notable. I mean, there are some novels like that, sure.
We have prompts, not specifically for Willow Creek, but we found that certain types of prompts will eject it, at least from this particular latent space. More likely, though, maybe 30% of the time, it would still be Willow Creek. That's why we always say the solution to this kind of trope is to put in your original content. Please don't start from scratch and generate the entire novel from one paragraph. It just doesn't work well. If you want to do that, we're not stopping you, but you're probably going to end up with stuff that falls into the easy spaces, the easy tropes, that ChatGPT offers up.
That said, we do try some trope detection to catch things like "Willow Creek." It's funny; our users actually have lists they share with each other of all these tropes that occur over and over. We have something called a plugin system in Superwrite, where they can make their own prompts and share them with our user base. People have made trope detectors and trope "washers" that present a list to the AI and say, "Do not write about this," or, "Please detect when you see this so I know how to rewrite it." These are like band-aids. I think the right solution is probably some kind of fine-tuning because we found that when you fine-tune, Willow Creek goes away, especially when you're doing it on a particular author's corpus of works, which doesn't include Willow Creek.
[00:35:43] Raza Habib:
To get that to work in practice, how much of the author's work do you need for it to be a plausibly good fine-tune?
[00:35:49] James Yu:
Not that much. In our current workflows, we're seeing that even just two or three chapters are enough with the current state-of-the-art models for what I call "unmasking." As I mentioned before, I love DaVinci, and deep inside the reptile brain of GPT-4, DaVinci still lives. I like to say it unmasks all these tropes. As soon as you do even a little fine-tuning, we see much more original content.
I hesitate to use the word "original" because what's original to one person is a cliché to another, but in our tests, our writers say, "Yeah, this sounds more like me." Especially when you do a side-by-side comparison. That being said, more content can be better too, so we're experimenting with people uploading multiple series of novels—like 500,000 words—but I see diminishing returns after about one novel.
[00:36:55] Raza Habib:
Final question on how you build this, and then I want to talk more about the bigger picture—what it means to have AI for creative work. What skills are needed to build something like Sudowrite? If someone's assembling an AI product team today, what's the structure? What kind of people have you brought together? Do you have people with deep machine learning expertise?
[00:37:18] James Yu:
I feel strongly that any AI startup needs domain expertise, which might be the most important thing. If you're making a legal startup or a chef AI tool, you'd better have cooking experience or consult with chefs. Domain expertise is necessary to bring something from a cool Twitter demo to something retentive, like how novelists want to use our tool daily.
Half our engineers have English majors, or they're avid readers or writers, so they understand the domain and the problems writers face. That was the number one thing. We're mostly full-stack engineers because I see the UX as a lot of the value we're delivering. With the newest models, it's easier to have a team that doesn't need PhDs in machine learning to deliver a high-quality product.
That being said, we are up-leveling on machine learning. We have consultants, and one of our engineers is taking the torch as our ML engineer. I would say all of our engineers have deep experience in prompt engineering and use these tools daily. The other big aspect is community. I'd say about 50% of our effort is cultivating a community around the tool, especially for a creative tool like this.
We even employ community members to teach others—like thriller or romance writers teaching beginners. We also have a designer, but many of our engineers are good product engineers too. I'm looking for people who have that full-stack vertical experience and can understand user problems, work with our designer and machine learning expert, and figure out how to deliver solutions.
[00:40:03] Raza Habib:
So you prioritize domain expertise over AI expertise, and then you staff the team with full-stack people who can understand user needs, ideally with prompt engineering experience? In order, you’d prioritize domain expertise, full-stack engineering, then prompt engineering, then ML experience, right?
[00:40:26] James Yu:
Yes, unless you're building models from scratch. But if you're making AI tools for real people, that's the structure.
[00:40:34] Raza Habib:
Some people refer to the "API dividing line." Some build models, and others build products using those models. There's an abstraction barrier now.
[00:40:43] James Yu:
Yes, that’s a good way to put it.
[00:40:43] Raza Habib:
So AI for creative work is something that could be quite controversial. I remember a moment a year and a half ago when "AI" with a big cross through it was trending on ArtStation after all the image models came out. Also, I know when you launched Story Engine, the first version, you had a mixed response on Twitter. Some were very positive, but others almost tried to cancel you. Could you share that experience? Why do you think critics reacted that way, and what do you think they got right or wrong?
[00:41:27] James Yu:
Yeah, when we launched Story Engine, I posted a video about how you could collaborate with the AI to flesh out an entire chapter. This struck a chord because it was during the WGA strike, and lawsuits were in the news every day. My video caught that zeitgeist, so the reaction was very polarized. Some were anti-AI for any creative process—text, images, video, etc. And on the other side were those who saw it as a tool, thinking, "Oh yeah, this could help me with my craft." The nuance got lost.
[00:42:11] Raza Habib:
The nuance definitely got lost. I'll link to the original post in the show notes because it's hard to communicate the visceral emotion both sides felt. You clearly struck a chord, and AI and creativity evoke strong emotional reactions.
[00:42:33] James Yu:
Yes, very strong emotions. It was a tough week.
[00:42:37] Raza Habib:
I can imagine.
[00:42:39] James Yu:
I think it speaks more about how social networks are structured. People dunk on things in line with their tribe, and there’s no nuance.
[00:42:52] Raza Habib:
And it's so easy to criticize something without actually doing anything yourself.
[00:42:57] James Yu:
Exactly. No one watched the video either. The analytics showed that people watched one second of it. It was all retweets and brigading. In retrospect, I struck a chord and sparked interesting discussions about how AI fits into the creative landscape.
It's still a mixed conversation. Just last night, Timbaland posted about using AI to flesh out old songs, and in music, it's more accepted because of more technological innovation. But in the literary world, tools haven’t really penetrated content creation in a meaningful way. Maybe you could say spell check or Grammarly features have, but how we write hasn’t changed much in hundreds of years.
Since 2020 with GPT-3, we’ve had to wrestle with philosophical questions that used to be sci-fi but are now real. We have a tool that can understand your story, give suggestions, and even write parts of it. It’s a big shift, and I understand the fear—is it going to take jobs or be trained on my works? I do believe authors should get compensation for their works being trained on, but how we figure that out as a society is complex. It’s a question the courts are dealing with now.
[00:45:55] Raza Habib:
I think there's a spectrum. To an extent, AI is just another tool, like Photoshop or a camera—it helps us achieve our goals better and faster. But there’s a qualitative difference between a tool that can have its own goals and reason like humans. We're not quite there yet, but we’re on that trajectory, with systems that have goals and subgoals, and so they'll have to start having goals of their own. That's a key difference. Photoshop can't decide to do something on its own, but AI systems can already do that, like recommender systems.
[00:47:01] James Yu:
Yeah, I think there is a gradation. Even in Story Engine or Story Bible, under the hood, we're giving the AI a goal like, "Create an evocative scene." Some people have experimented with having the AI write an entire novel at the push of a button, but that doesn't stop you from writing your own novel. I still believe that people will want to read stories where humans are part of the creative loop.
[00:47:32] Raza Habib:
Something I worry about, and others do too, is what gets referred to as "AI slop"—low-quality, high-volume content that overwhelms the good stuff. Could bad writing flood the market and make it harder to find high-quality content?
[00:48:05] James Yu:
I don’t worry too much about that. There's already a lot of human slop out there, and the good stuff still rises to the top. Even before AI, there were lots of bad books and movies. We have recommendation algorithms, and word of mouth is still king. How do you figure out what book to read next? Most people would say, "My friend recommended it."
I don't think AI slop will edge out the good stuff. The really talented writers will begin adopting AI in their process, and I trust their taste. Sure, there will be people trying to push out spam with AI, but I think that will be temporary. As a society, we'll still prioritize the good stuff.
[00:49:26] Raza Habib:
One last question specific to you, then one we ask everyone. When people read a novel, they often feel a connection to the author. It’s that sense of connection that makes a book valuable. Do you think AI might undermine that?
[00:49:53] James Yu:
I think that goes back to human trust. Throughout art history, artists have employed teams to help create things. They weren’t doing every single part of the process, but the final piece still reflected their taste and their connection to the human experience. I don’t see it being any different with AI. Some people will try to generate everything without reviewing it, but that's not the same as what a thoughtful creator would do with their work.
[00:50:39] Raza Habib:
That resonates with me. We still talk about AI in terms of existing literary forms, but we haven’t touched on new literary experiences AI could create—novels where you interact with characters or fork the story. There’s so much possibility, but let’s wrap up. We ask everyone this: if you imagine the world five years from now, what will be the same, and what will be different because of AI? And is AI under-hyped or over-hyped?
[00:51:37] James Yu:
Great question. In five years, I think things will move slower than expected in some ways. Four years post-GPT-3, we’re seeing uneven productive use of AI in different industries. In some verticals, like coding, AI is a boon—everyone's talking about tools like Cursor. But AI can’t just autonomously create everything, and I think the hype around agents was overblown. I see those timelines being longer, especially for regulated industries.
We’ll see breakthroughs in verticals that surprise us. I think we'll see major breakthroughs in coding—I'm closer to that area. But in the literary world, I think AI adoption will grow, but there will still be debate. In music and audiovisual sectors, adoption may be faster. In the physical world, I use Waymo in San Francisco, and I think autonomous vehicles will be widespread in five years.
In terms of what's under-hyped and over-hyped: I think agents are over-hyped. Cool demos, but not many real-world applications yet. That might change, but I see it further out. Prompt engineering, on the other hand, is under-hyped. We’re only scratching the surface. Even people at OpenAI and Anthropic have barely tapped into what’s possible. These models are like alien black boxes, and we're still figuring them out. I think multilingual prompts are another under-explored area.
[00:55:42] Raza Habib:
On that bombshell, maybe we should end the conversation here. James, I’ve really enjoyed chatting. I think Sudowrite is one of the more interesting AI applications out there, helping people be more creative. It’s had a lot of success in retaining a large user base, which is rare for AI products. Thanks for coming on.
[00:56:04] James Yu:
Thanks, Raza, for having me.
About the author
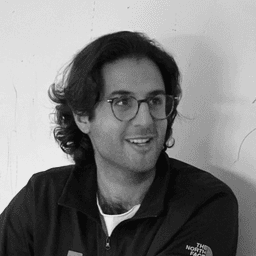