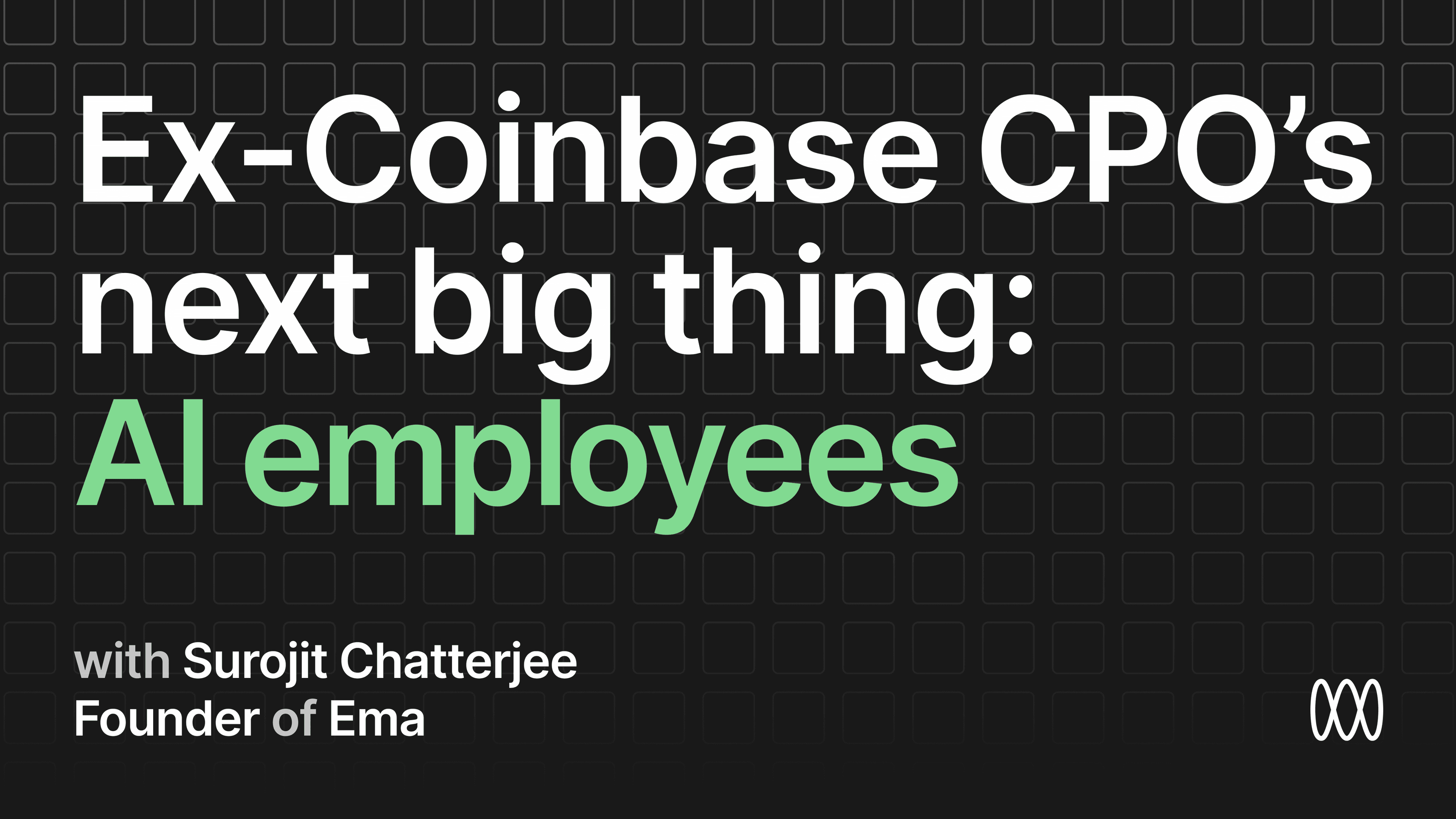
Building AI Employees with Coinbase's Ex-CPO
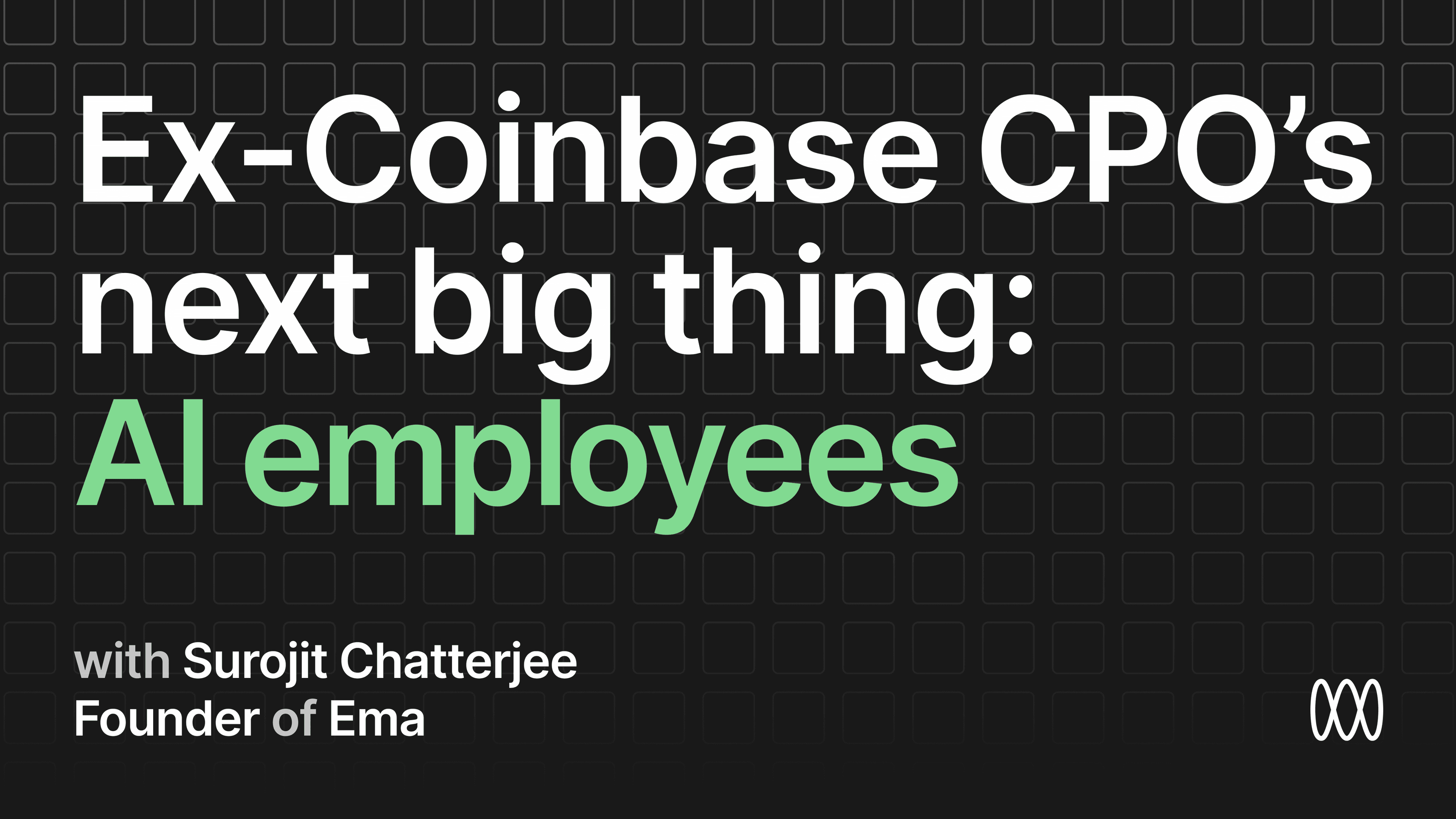
In this episode of High Agency, we're joined by Surojit Chatterjee, former CPO of Coinbase and now CEO of Ema. Surojit unveils his audacious plan to create universal AI employees and revolutionize Fortune 1000 workforce. Drawing from his career at tech giants like Google and Coinbase, he shares how these experiences fueled his vision for Ema. Surojit dives into the challenges of building AI agents, explores the concept of artificial humans, and predicts how this technology could transform the future of SaaS
Subscribe to Humanloop’s new podcast, High Agency, on YouTube, Spotify, or Apple Podcasts
Chapters
(00:00:00) Introduction and Surojit’s background
(00:03:00) Founding story of Ema (Universal AI Employee)
(00:04:53) How the Universal AI Employee works
(00:08:39) Ema’s data integration and security
(00:12:57) AI employee use cases in enterprises
(00:15:02) Challenges with building AI agents
(00:16:45) Evaluations, hallucinations, customizing models
(00:19:52) Artificial human metaphor
(00:25:42) AI employee vs humans
(00:31:25) Advice for AI builders
(00:37:14) Is AI overhyped or underhyped?
(00:39:28) How the business model of SaaS will change
Podcast:
Raza Habib (00:00:00): I'm really excited today to be joined by Surojit Chatterjee. Surojit has a very impressive career, starting from one of the prestigious IITs in India. He was an engineer and researcher at Oracle, had eight years at Google where he ran mobile search ads and grew it to over $50 billion of revenue, was a product leader at Flipkart, the CPO of Coinbase, and now he's the CEO and founder of Ema, a company that's trying to build a universal AI employee. So Surojit, my first question is you had a very impressive career. Two-part question. One is, what should people know about you that maybe they wouldn't find out about from reading your LinkedIn profile or doing a Google search? And given the career you had up till now, what made you want to take on the pain of going back to being a founder?
Surojit Chatterjee (00:00:45): Great question. First of all, thanks so much for inviting me to the show. I'm excited to talk to you. What do they know about me? That's not on my LinkedIn. I always get asked this question and it kind of stumps me. Maybe one thing is almost everything in life is serendipitous. I never planned anything. I never built a resume after my very first job, like maybe 20 years back. And it sort of happens to you a lot of things in life. If you just keep focusing on what you like to do, and that's what I have tried to do. I love building products. New products in new areas. So, you know, I was building products in mobile. And mobile was just starting off. I was building products in crypto and blockchain in early days. And now I'm building products in AI. So that's what keeps me going and that's exciting.
Raza Habib (00:01:41): And what about the second part of that question? So you will have had many options, CPO of Coinbase. You could have gone on to many other things. You've chosen to go back to be a founder again and start from scratch. What is the founding story of Ema? What motivated you to go do that?
Surojit Chatterjee (00:02:00): Look, I grew very large teams from small to very large at Google and started with two-member team to build mobile ads. And as you mentioned, we hit like 50 billion. And now, I think mobile ads is $100 billion plus business for Google. It grew to be very large. Same with Coinbase and Flipkart. And in all these places, we hired like really good talent, very good talent. But what ends up happening is you have this great talent, and then half of their time or more than half of their time is spent in just KTLO - keeping the lights on. This repetitive tedious task that has sort of soul crushing part of your job that you don't know what to do but you have to do it to keep things moving and that's something that bugged me for a long time. We went through some rough times say at Flipkart right we had to Let go of 20% of our employees at one point, right? Same at Coinbase. Then it was like, okay, how do we do more with less people, right? Because that work doesn't decrease by 20%. And one thing that are my engineering counterpart or the company operations counterparts who tell me most of the people are just maintaining what's already there. They're not involved in building new things, creating new stuff. So that's the genesis. How can you free up employees to focus more of their time and energy and building new things, creating value, and how do you automate all of these tedious tasks using AI?
Raza Habib (00:03:38): And can you describe what Ema is for audience members who will not know? So from the website, it purports to be a universal AI employee that could manifest in lots of different ways. So I'd also love to hear like literally when I open it up as a customer, what do I get to see?
Surojit Chatterjee (00:03:53): So two years back when we started working on it, we called it a universal AI employee. We still call it, universal AI employee, because that kind of grounds us into how we build the product. And essentially, what we started building two years back and no one was talking about is Agentic AI. So every AI employee is a collection of agents, is an agentic mesh or swarm of agents that's orchestrated automatically by our no-code platform. So every employee basically is we have a small model that translates a customer problem into kind of a workflow. Then that model will recruit multiple agents to perform that workflow and also generate the orchestration code for managing that workflow. That's kind of very simple way to think about it. So what it does is this AI employee, which is like an Agentic mesh, it mimics a human role. So it could be a role in, say, customer center automation, sales and marketing, could be legal, could be HR, could be anything. And it can do tasks similar to what humans will do. A big thing is it can collaborate with you almost seamlessly. And so what do you see when you go to our product? There are a B2B product, enterprise only product. Sell to largest enterprises on the planet, like Fortune 1000 type customer. What you see is basically like a mesh of agents where you can configure them. So you just connect data sources to them. And we have already connected with hundreds of enterprise apps. So it's like two click. You'll say, okay, connect to my Salesforce or Workday or my SharePoint or whatever your data is structured or unstructured data. You'll give some natural language instruction and say, do this or do that. It learns from your existing documents, your existing SOPs, existing workflows, wherever it is written. You can also give additional instruction, just like what you will do to a new employee. You will onboard the employee. I'll give that employee some instructions. You will tell the employee what to do or not to do.
Raza Habib (00:06:04): And what's the action space of that agent? So once it's connected up to this data source and I give it some instructions, what is it capable of going and actually doing?
Surojit Chatterjee (00:06:13): So our agents have a very wide set of capabilities. They can take actions, use tools in the enterprise. So they can, for example, write into any of these applications that are connected. Let's take an example, the contact center automation. So a lot of our customers are using us. to resolve L2/L3 tickets, like more complex tickets where you always need a human today. So usually what happens is a ticket will come or the end user, your consumer will hit a chatbot. The chatbot will try to resolve the ticket. And if it cannot, then it's filed as a real issue or a ticket and humans will try to resolve them. So what Ema will do is you connect Ema to your ticketing system. It could be anything, Zendesk, FreshDesk, ServiceNow we are connected to 30 plus ticketing systems today. You connect Ema to your SOPs, your policy documents, and other databases, other applications. And it will look at past tickets, automatically learn how to resolve tickets. We'll tell you this is how I think of your tickets. This is how I'll categorize. This is how I'll resolve. You can give some instructions. You can fine-tune a little bit just using natural language. You can click a button and say, OK, go resolve the tickets. You can tell Ema to do it in a way so it doesn't actually answer the end customers because you want to still validate. You can do that in internal only mode. Or if you feel confident, you can say, okay, just answer to the end customer. It will behave just like a human agent. So it will be just like another employee, Ema, sitting on your Zendesk or FreshDesk or any of this app, answering the tickets or sending emails back to humans.
Raza Habib (00:07:57): And for those integrations to things like the ticketing agents or whatever, is it operating at the level of the UI? Is it seeing the pixels and clicking buttons? Or is it directly via the API integration?
Surojit Chatterjee (00:08:09): Yeah. So underneath, we have done a lot of API integrations. For the end user, you are just doing a few clicks deploying. So that's another thing we have done, which is it's like fastest to deploy, fastest to value. and also all legitimate applications today.
Raza Habib (00:08:26): So I guess a couple of questions. I've spoken to a bunch of enterprise leaders who are building AI products about the build versus buy distinction and in certain cases why they chose to build internally. And something I hear really commonly is that the data integrations that are required to make the AI useful, are very extensive, right? Like GPT-4 is very smart, but the things that are needed for it to be really useful is it has to have access to all the customer data, it has to have access to the CRM data, to the previous customer support, those integrations you just mentioned. And people are often nervous about giving third party access to that information. So how does the data integration work and how do you guys get the permissions to make that work successfully?
Surojit Chatterjee (00:09:05): Couple of things. One, we have done a lot of these pre-integrations. So we have integrated with API access to almost all known or mostly used SaaS applications out there, all the cloud storage, different types of databases and so on. What that means is as a user, you have to just authorize, you don't have to integrate two clicks, three clicks. You can just authorize Ema to use your data, right? And you have control over what part of data Ema should access. You don't need to give access to everything. I can say only access this part and so on.
Raza Habib (00:09:39): Did you have to build an auth layer yourself for that or are you able to leverage some kind of existing off platform?
Surojit Chatterjee (00:09:45): We have built a lot of it like ourselves because what we saw is what exists out there sometimes doesn't meet our needs. So we have a lot of engineers with deep expertise in data, like large scale, and I spend a lot of time at Google. So you have a few Googlers in the company, people from other companies like Informatica, Databricks, and so forth. So people with that kind of experience. The other thing is, like you said, companies, enterprises are legitimately so, very wary of what happens to their data. Will it leak to LLMs? First, our approach is we offer a regular SaaS configuration, multi-tenant SaaS, like any other application. We also do single tenant SaaS. We can just make sure that it's only you as a tenant. And we do on your cloud instance. So then it's like completely shielded off.
Raza Habib (00:10:42): Presume you still have to call out to the models, right?
Surojit Chatterjee (00:10:45): Yeah, so for largest enterprises, basically all of our software now can live in their cloud, like their Azure Cloud, their GCP Cloud. We are cloud agnostic, we have containerized everything. And large enterprises, what happens is they often have a private endpoint to, say, OpenAI or to Gemini and so on. So they have done that negotiation with Microsoft or Google.
Raza Habib (00:11:13): So you're leveraging that existing. Okay, so integration is one half of it. The other half is what I mentioned before, the agents being able to take actions. I would imagine if you only have API access to these applications, some of them, the things the agent might be able to do might be quite limited. So I wonder whether you do go do anything to go beyond that or do you plan to do something to go beyond that in the future where the agent might be able to, you know, we have vision models now. You can see the elements on the screen. You could imagine interacting directly with the UI.
Surojit Chatterjee (00:11:40): So far we have found the API is being quite robust and meets the needs. But you are right, they don't give access to every piece. So in the future, we may do other things, but there is a lot of ground to cover just with API access. There's so much automation we can do just with API access. Also, the API access makes the customer feel, frankly, a little more safe because it's very clear what data you have access to and what you don't have access to. Through a UI, there's kind of security challenge, et cetera, that comes safe.
Raza Habib (00:12:16): And how are people using Ema today? So what are some of the most common use cases? I want to get to the point where people can really be visualizing what this looks like in practice.
Surojit Chatterjee (00:12:25): Yeah, so we are a horizontal platform. We can basically build this AI employees for any kind of function, any role. Where we see a lot of demand today, two areas. One is customer center automation, which I'm sure a lot of people are talking about it. What's the unique about us is we deliberately attack the most complex part of that customer center automation piece, which is resolving L2 L3 tickets. We can do all the other things too, but resolving tickets, not just answering, like looking at some knowledge based on answering. This requires checking actions, changing data and state applications. We also see a lot of demand in sales marketing automation, so things like generating business proposals. Some of these are very large like 300 page documents, can you generate them? automatically, generating leads, sales leads, business intelligence, a variety of use cases in the sales and marketing automation side. And then we have customers using us for a bunch of other very interesting use cases, like HR being one, like internal employee experience, right? Employees have questions about policies or they own file tickets or expense report or whatnot. They can do all that. But also legal teams looking at do these contracts comply with my policies. We have healthcare use cases, healthcare companies using us for accelerating, say, prior authorization of treatments and so on. And often what happens is our customers will start with kind of a known use case or regular use case and then extend to other use cases. So every one of our customers have used us for at least two or maybe more use cases to And you obviously built a lot of different types of frontier technology products.
Raza Habib (00:14:18): You mentioned being mobile first, then you're involved in crypto, now AI. What's different about building AI products from other types of product categories? What challenges have you faced? What have you had to overcome to do that?
Surojit Chatterjee (00:14:30): AI is a fundamentally different type of beast and different type of software. I've built a lot of products in my career. And starting AMI was like, okay, how hard could it be? And it seems like a lot of this is highly technical kind of engineering work, right? Changing a model, this, that. Turns out there is actually a very large amount of product work involved in building products for AI. And to give some examples, these products are unique in the sense that the same product, same AI employee can behave very differently for different customers based on what feedback they give to this employee. It's very similar to a human employee. You can hire similarly capable employees. And then if you give them different feedback, different type of jobs, they kind of evolve differently. They will perform very differently maybe in a few years. Human feedback can fundamentally influence behavior. And if you give good feedback, it improves. Second thing is, that's why we call it an AI employee LLMs are stochastic processes, right? So they are not 100% accurate. We got a great length to eliminate hallucination. But in some cases, it will hallucinate.
Raza Habib (00:15:52): Can you tell me a little bit about those lengths you go to? I mean, just so you have the context, right? At Human Loop, what we're focused on is evaluation and helping people measure performance. So it's something that I spend a lot of my time thinking about. And I'm always curious how other people approach the problem. So how do you guys do the work of evaluation, of reducing hallucinations, of customizing the models as well?
Surojit Chatterjee (00:16:14): Let me talk about evaluation first. So every one of our AI employees come with a Turing test embedded which means they will show you their work and they will show you equivalent kind of human work side by side and ask you to give feedback. And that's super helpful because we humans can understand, okay, is AI, at bar with humans, better than humans, worse than humans, and make the judgment.
Raza Habib (00:16:43): How does that work? Where do the initial test cases come from? So I hire Ema, I install Ema, as it were. I'm now going to get these test cases. What is the set of test cases that is running on initially?
Surojit Chatterjee (00:16:54): Yeah, so let's take the example of resolving tickets, right? So I'm going to look at past tickets, we'll learn from them, but then we'll keep a portion of the past tickets just to test, right? It'll start answering those tickets based on the learning and also show you side by side or kind of an interesting gamified file where we'll show human answers sometimes. And you as an internal expert will rate, oh, is this a good way to resolve the ticket? Is this the right resolution? How would you rate Ema? So that's how it would do it. So any role we are getting into, there is some precedence, right? Some humans have done that role before. So we are always looking at, okay, past work by humans and saying, let's attempt that and see how close we get to humans. You also ask a question about what we do in terms of eliminating hallucination, right, or reducing hallucination. We have a very unique approach there. We have a smaller model called Ema Fusion. It's a mixture of experts model. It actually integrates many public LLMs and many small models that we create in a very unique way. Typically, a mixture of experts will be like we can take open source and models and march the top layers of the model. But we are integrating both open source as well as closed source models in an interesting way. What we often do is figuring out what's the best combination of models to talk to. So we'll cross-reference the same question with multiple models, for example. That has the interesting impact of eliminating bias or reducing bias, as well as improving the answer because we are now combining answers of multiple experts, right? Now this is in doing this in real time will be very expensive, right? We are not like going and asking 100 models. How do you answer this? So we trained a model that learns this offline with generating multiple incorporate multiple experts. And then in real time, it will figure out, okay, it memorizes it has embedded in its model how to execute this particular query, this particular scale in real time.
Raza Habib (00:19:13): And you guys have chosen to take an anthropomorphic metaphor in terms of representing this. So you represent it as an AI employee. And that metaphor obviously will affect how people think about the model's capabilities and also as a statement of intent almost in what you want the product to be able to do. You didn't have to choose that particular metaphor. So why did you sort of want to present this as an artificial human versus a new toolkit or a chat bot? There's a lot of different ways you could talk about the product. How come you went for that one?
Surojit Chatterjee (00:19:42): Yeah. So first thing is we are like Ema can chat, but it's not a chat bot in the tradition of chatbot, right? Basically, it's taking actions, it's automating complex workflows. Many of our example is multi-step workflow, but generally walkflow. These are not RPA as like fixed if-then are statements, where every step, it's making some independent decision. based on some reasoning it's doing on the data, if you think about that. So that's why it's not a chatbot. Every time, you know, I got a pushback over this employee seems like difficult to understand.
Raza Habib (00:20:21): The reason I asked this question just to clarify, or like the reason I was thinking about it is To knowing what your product does, it feels like a reasonable metal. In fact, it's a complex agent that's automating workflows. But there has been a history of snake oil salesmen in the recent past who have been promising something like this and then not delivering. And so the concern that I would have is... both that you get tarred by the unfair brush of what's come before, and also that people's mental models, the kind of metaphor that they're approaching the model with might be slightly wrong in that the models are human-like in many ways, but they fail in ways that humans wouldn't fail, and they're superhuman in certain ways. I wondered, like, how you approach that challenge of the user expectations that are set by this choice.
Surojit Chatterjee (00:21:07): I think calling it an AI employee actually helped me build a product in the right way. Because a lot of what we do with MI is very similar to what you will do for a new employee who is onboarded. For example, if you hire a very smart new employee, that employee, even though very smart, may not be very effective on first day at your company, because they don't have any context. They have not seen any of your data, don't know your processes, and so on. So you have to train this new employee, giving them your onboarding document, giving them access to systems and so on. It's very similar. You will have to do the same thing. The other thing here is, this is kind of another learning. We have worked with many customers. Unlike the regular software, generally AI, software can degrade in performance, can upgrade in performance. So it's not a static. If you buy any regular SaaS app, it just works the same way unless somebody pushes new code on the back end. But that's not the case here. The models are changing all the time. And based on what the model is learning with your data, it can change behavior. So you have to periodically give feedback to this employee, just like Even if your best employees, you give feedback every three months or six months, right? You have to help them grow and help them get better. A very similar paradigm. How will give instructions to this employee? Because this AI employees also need instructions just like human employees need. And they have long-term memories. So we have every one of our AI employees have like long-term memories. They will remember it. You can ask it to change its memory or revise its memory to do that. So that paradigm really helped us build the product the right way, actually. We are very focused on creating ROI. So you said, there have been many instances, almost over promise and underdeliver, fully aware. And what we did is for first year and half of our existence, we are completely under the radar. We didn't even have a website. And we were not advertising, publishing anything. We just wanted to make it perfect. And even now, when we go to the customer, we take this high burden on ourselves to prove ROI first. So our pricing model is very different. It's not based on a number of seats, which will also make no sense because the reason you are hiring this AI employee is to augment your human employees. But we are We price based on outcome or based on number of tasks, it's performing and so on, based on usage and outcome. So we take that on us upfront to prove ROI to create value. And without creating value, there is no business.
Raza Habib (00:24:11): So maybe just to summarize my understanding, the reason why actually having this metaphor of an AI employee is powerful is in some ways it might misset expectations, which is my concern. But actually, in some ways, actually it does it really nicely in that your expectations of an employee upfront are that there's a ramp-ups phase where the employee needs to gain context. And you naturally have an expectation of an employee that its abilities will change over time and that its capabilities might increase, that the tasks you delegate to it might change. which you don't have of typical software. And so actually this metaphor, in some ways, is actually shifting people in a positive direction where they're thinking about, okay, this won't work for me on day one. I have to get the context day and I have to train it. So there's an expectation of a ramp and also there's an expectation of continued improvement that maybe they wouldn't have from typical software.
Surojit Chatterjee (00:25:04): Absolutely. I'll give you a real example. This is one of our clients. We have case studies on them published. We worked with them first couple of weeks, and they are one of the largest immigration attorney companies. So they help other large companies, their employees with visa filing, H-1B filing, green card filing, processing, etc. So very complex use cases. These employees will have very difficult questions for these attorneys, often very personal question. Every case is different. So they have to give a personalized answer, not just a generic answer. and looking at their case history and so forth. So we'll give them kind of initial responses to past tickets, the way I described, we always validate. And their expert attorney would be like, no, this is not there. So we went back. Second week, oh, it's better, but not there still. Third week, they're like, yeah, it's getting better, but not there. Then in the fourth week, I'm like, okay, I sent you some more answers and tell us how it did it. And they're like, oh, actually, it kind of did worse. What happened? It was getting better. I was like, actually, the last time I sent you, what if humans did for the same tickets? And that was the kind of the realization that, oh, actually our humans are also not perfect. So the best agents are doing like what their expert lawyers will say like 90% accurate, not 100% in terms of comprehensiveness of the answer and so forth. And AI is surpassing humans, but the definition of 100% accuracy is also vague or not there, right, in many of these cases. That's one example where this AI employee paradigm helps the customer also understand, okay, now I have something that is better than my best agents, but one hundredth of the cost. It seems like a good value. The other piece of it is in that process, like three, four weeks when we were improving it, every week we'll find out, oh, we forgot our human employees actually look at this database. or look at this particular website or this particular forum or this other data, private data that we forgot to give you. So there's this data discovery phase that happens. Because most customers, their data is not very well organized. It's a lot of tribal knowledge, right? The people in the role know what to find the data. They don't necessarily write it down. They just tell others, other people just know. So that also helps them comprehend, okay, imagine this is a new employee. And you have not told this employee where the data is, how do you know? It cannot go on its own hunt the data because that will also be a secure bridge.
Raza Habib (00:28:01): How long does that process typically take? Like how long till you feel like you're at steady state in terms of ramping up the system?
Surojit Chatterjee (00:28:08): Yeah. So now we have lot more learnings with working with customers. We do a lot of the discovery early on. We know what to ask and so on. So it's much faster. Within two to three weeks, we can deploy very quickly. First of all, it's like a few clicks to deploy. We can integrate really quickly. Unless they have like really bespoke database or application, then we have APIs, we implement that we take one or two weeks. And then within a couple of weeks, three, four weeks, max, we can get to a place where we are showing outcomes similar to their human employees, as long as we have similar information. Sometimes some information is not accessible easily. Sometimes there are delays in security approach. And in normal cases, that's the timeline.
Raza Habib (00:28:54): And what happens next? Do they just start cutting staff? What is the impact on these companies? Are these directly substituting for employees, as the metaphor suggests? Or in practice, is there something that's freeing people up to work on other things? There's a lot of speculation about this. You probably are one of few people with actual first-hand experience. What happens in practice?
Surojit Chatterjee (00:29:14): So for our customers, many of them were already understaffed. The economy has not been the best, so they have not hired for a while. Their existing staff were overwhelmed. So it actually released some pressure. It improved their customer experience, right? And their employee experience as well. We have some customers who are very high growth customers, like their revenue growing 200%. Year on year, they can't kind of staff up fast enough to pace that revenue growth. So in many cases, it has helped them pace their growth better.
Raza Habib (00:29:52): And I guess also for something like support or sales, where if you might be in a seasonal industry, you can't just suddenly ramp up a large number of new employees and ramp them down again if it's seasonal, but with an AI system, you actually can.
Surojit Chatterjee (00:30:05): We have a very interesting use case in India. We work with a company called MoneyView. It's one of the largest B2C lending companies in India. They have like 50 million users or maybe more at this point. And they have this seasonality every month. So basically people will take kind of loans, payday type loans, end of the month, and then they will pay back and they get their salary, a short-term loans. So they need a lot of stuff in the last week of every month, and then they can ramp down. And that's very hard to manage. Every month, how do you do this month over month? So it's been very helpful for them to manage that extra volume every month. with AI employees.
Raza Habib (00:30:47): Throughout your career, what advice would you give to a product leader starting off today who's building an AI product maybe for the first time? What lessons would you distill to them?
Surojit Chatterjee (00:30:57): First thing is there is so much noise in this market. Don't believe in the noise. Just actually build it and test it because there is half the research papers I read, we try everything out. We have filed lots of patterns and new technology ourselves as well. But there's a lot of things that are out there that people will claim working, not really working in production at scale. So that's the first thing. Have a good amount of skepticism. If you are basing your product choices based on like Twitter feed, not the best tweet. Test it out yourself. And the other thing is the market, there's enormous amount of confusion amongst customers as well on different kind of technologies. What are the layers of this technology? It's still very early. So testing with customers is the best way. You can build a product on your own or do research. That's not enough. A lot of your assumptions will change. So we always build with customers from day one. We've built with multiple design partners. We do a lot of research, but very applied research. Those are a couple of things I'll say. And the last thing is for any entrepreneurs, there will be setbacks, don't give up, and don't get intimidated. Because it seems like everybody is doing everything.
Raza Habib (00:32:23): On that point, how do you think about competition for AI products more generally and also for yourself, right? In many ways, you're directly competing with the large foundation model providers. You're now competing with, say, Salesforce, Dreamforce. This vision of using AI agents to liberate tasks in the enterprise, is one that a lot of people are not going after. What do you think about that competition?
Surojit Chatterjee (00:32:46): So first, we made a very deliberate choice early on to leverage every model out there, not completed them. See, there was an alternate model to what we are building. This was two years back. The word agentic was not even coined. So when I went and talked about AI employees to many investors, I think 80% of them did not understand, right? Oh, are you building a model? Why don't you build a model? And there are some companies who are building like an action transformer and so on. I think that's an alternate approach.
Raza Habib (00:33:21): I don't know how it's working. It's not worked out for some of them, right? We know that Adept was acquired. And to be honest, the model layer seems to be increasingly commoditized, even amongst the top providers. OpenAI currently has a slight edge with 01, but everyone's fighting over the top spots in these leaderboards.
Surojit Chatterjee (00:33:36): So this was my hypothesis about the future two years back. I am not going to build another foundational action transformer or another foundation model to teach LLMs how to take actions. That's the core of the problem you're solving, right? Teach LLMs how to take not just action, but sequence of actions, like create workflows and so on. We did that with kind of an agentic architecture and a very sophisticated agentic architecture because we use swarm of agents and there are many design patterns. of those agents, like how we organize those agents and so on. That's where we have spent our energy. The interesting part is as the underlying models get better, our product gets better. We are not in conflict with them. Neither are we trying to batch up things that don't work today. So that's another thing. When we started working on this, the models were more very expensive, high latency, low accuracy. Okay, we are not going to solve the problems that we think will automatically get solved. over time. Solve the problems that we think will not be solved, which is the last mile problem, which is in the enterprise, how do you make this work? Because every task in enterprise in some way is slightly bespoke. Now, that's why you are using services. See, another thing, another choice we made is we are not competing with any, like you mentioned, Salesforce and everybody else has agents. I think that's great. Everybody should have agents, everybody should have a commerce social interface. Where what is interesting is, we actually like the heterogeneity of the enterprise. So we are able to work with multiple databases, multiple applications, tools, everything, right? Because that's why you need humans today, right? Because all the data is siloed, you need humans to take data from somewhere, make some judgment, recent decision. do something somewhere else, right? Can you do that with AI? That's the problem we are solving. So in some way, yes, there is a lot of competition and there's a lot of confusion, frankly, but we are looking at the horizontal kind of the heterogeneous enterprise environment, which I think will continue to be heterogeneous, and looking at using the foundational models as kind of a code layer, as you said, it's getting commoditized. So what we have done is our fusion model actually leverages multiple models. So we connect to GPT-4, GPT-4 all versions, Gemini, Claude, Mistral, everything that is out there, Llama, right? And any model that gets better, we benefit automatically because our model constantly figures out how to integrate the best models. all the time to get the highest accuracy at the lowest cost for every enterprise action. So that's the core IP, one of the core IPs we have developed that has served as well.
Raza Habib (00:36:31): So two final questions I'd like to ask everyone to get your perspective on. One is in the current market, do you think AI is overhyped or underhyped? And why?
Surojit Chatterjee (00:36:42): I think like every technology, it's overhyped and underhyped at the same time. Let me explain, right? everybody expects tomorrow there will be no humans in the workplace anymore and everything will be AI. That's not the case. What will happen is there will be a transition and humans will learn how to work with AI, just like we learned how to use computers to do our job better. So every human employee will become a super employee in a few years because they can just do a lot more. Every human employee will probably be managing scores of AI employees and working with AI colleagues. So that's the first thing. But it's not that this is going to happen overnight. Underhyped in the sense that we think, okay, this is not happening. That's the nature of technology. It seems like too slow and it happens all of a sudden. So there are many companies thinking, okay, this is all kind of hype and I just sit on the fence and watch it. I think that's a dangerous strategy as well. It's just like the internet, every company had to transform. People thought it was overhyped. But I think over time we see every kind of the promises of the internet have come to fruition. Yes, there are things that were tried in, say, circa 2000 that didn't work. If you remember, webband didn't work out, but Instagram works great. It's the same basic idea tried in another time when the underlying layers are built out, like payments is built out. The gig economy is known and how it works, it's known. And then if supply chain is figured out, then it works better. But fundamentally similar ideas. You'll see a lot of that, right? There'll be a lot of experimentation next few years. We'll see a few things working really well. And then other things may work a little bit later. It may not work today, but it doesn't mean that they will never work.
Raza Habib (00:38:41): So if you imagine the world five years from now, what things do you think will be the same versus what will have changed?
Surojit Chatterjee (00:38:50): I can talk a little bit about what will change. I think a lot of SaaS software fundamentally will change. I think the writing is on the wall that the business model of SaaS needs to change, right? Because the per seat model of pricing and this massive load of self-ware in every enterprise, right? Every enterprise now has like average, I think 300 or software applications that don't talk to each other and probably half of them are never used. I think that will change. I think this new type of software, agentic software, will make it very easy to move data from one place to another or make decisions based on disparate sources of data, right? And combine them to create more effective intelligence and decision making in the enterprise. So a lot of that will change. You'll see a lot of SaaS software companies evolving their strategy. You can already see how Salesforce and others are trying to evolve their strategy. Some of them may not survive because they may not evolve as fast. Others may grow bigger. It's a massive disruption. What will not change, I think, look, there will still be humans doing important work because this new layer of software will enable humans to have just more time for himself to do more creative work.
Raza Habib (00:40:19): Do you believe that even in a world where the models continue to improve? So there's kind of two possible reasons that may be true, right? It might be true because the models plateau in some way and the capabilities of AI systems plateau. And so there's only so far we can go with automated AI. It may also be the case that no, we get AI systems that are as capable or more capable than humans at most tasks. In that world, what will the relationship between humans and AIs be in your mind in a world where the systems do progress very quickly? Because I think both worlds seem plausible to me. As a machine learning researcher and someone close to the space, I put decent probability on both of these outcomes, right? Neither of them seems impossible or crazy. But I actually find it harder to reason about what happens next. So granted, okay, the AI systems really do accelerate very quickly, Reasoning about the implications for society still seems hard.
Surojit Chatterjee (00:41:13): Yes. I think any exponential solution of technology, it's always hard to predict what the impact will be on society. If you look at Industrial Revolution probably is the closest example of exponential evolution of technology. You know, 90% of humanity were farming before Industrial Revolution. And now probably less than 1% humanity is farming. We are all doing other interesting, useful work that all that free time enables has to do. So I don't think it will be like, okay, humanity has nothing to do because there are a lot more important problems to solve. I'm actually very optimistic about this, what humans can do in general, what we are capable of doing, solving climate change, solving energy crisis, solving other important big problems. it will just free us up to do more of that. Every enterprise, imagine if they could be a lot more productive, a lot more efficient. I think that will give rise to this proliferation of more enterprises, more startups, startups with 100 people making multiple billions of dollars, maybe, tens of billions of dollars. So I think it just opens up this possibility that humans can really tap into their true potential in more ways than before. I think we always underestimate our own potential. If we are not doing this farming job, there's nothing out there. Or if we are not creating one more TPS report, there's nothing else we could do. I don't think that is true. I think there are First, managing this storm of AI employees itself will be a task and humans would be needed to supervise in some ways. I think there will be lots of very interesting next generation of technology work where humans will be needed.
Raza Habib (00:43:18): On that optimistic note, I think that's a great place to stop. Thanks so much for coming on the show.
Surojit Chatterjee (00:43:24): Thank you so much.
About the author
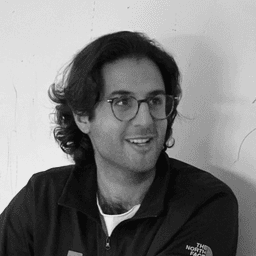