How to Create AI Strategy in Enterprises
In this episode of High Agency podcast, Peter Gostev shares his experiences implementing LLMs at NatWest and Moonpig. He discusses creating an AI strategy, talks about the challenges of deploying LLMs in large organizations, and shares thoughts on underappreciated AI developments.
Subscribe to Humanloop’s new podcast, High Agency, on YouTube, Spotify, or Apple Podcasts
Chapters
00:00 - Introduction
00:44 - OpenAI dev day reactions
03:47 - Using AI to automate customer service
10:43 - Impact of AI products
13:41 - Who are the users of LLMs
14:47 - Challenges building with AI in enterprises
21:22 - AI use cases at Moonpig
24:34 - How to create an AI strategy
28:10 - Underappreciated AI developments
Podcast:
[00:00:44] Raza Habib: Today I'm joined by Peter Gostev, who's the head of AI at Moonpig, an e-commerce company specializing in gifting. Before that, he led AI strategy at NatWest, one of the largest banks in the UK. So, Peter, thanks for coming on the show.
[00:01:05] Peter Gostev: Brilliant, thanks so much.
[00:01:06] Raza Habib: So, Peter, I want to dive into all the work you've been doing at Moonpig and NatWest. You're an avid writer about AI products and services and deeply involved, but before we dive into that, today is the day after the OpenAI Dev Day. I'd love to get your first reactions on some of the announcements and how they might affect your work at Moonpig, if at all, and what it means for others in the ecosystem.
[00:01:28] Peter Gostev: Yeah, I think it's a really interesting release. Every time new products and features come out, I challenge myself to think about what else I can do with them. It's easy to see a release and think, "Oh, that doesn't matter much," and move on.
[00:01:47] Raza Habib: Right, they announced real-time voice, some evals and fine-tuning support, prompt caching, a 50% reduction in cost, and the ability to opt into having them train on your data and get free inference. Anything else that excited you?
[00:02:11] Peter Gostev: They also announced fine-tuning for vision models, which was interesting for us. At Moonpig, we have about 50,000 greeting cards, and we used visual models to analyze them and create extra tags for search. It cost us maybe £300 or $500, and even if the benefit is small, it's worth doing. With fine-tuning, we might have done it better.
[00:03:02] Raza Habib: Were you excited about the announcements overall, or underwhelmed?
[00:03:09] Peter Gostev: I'm always excited because something might end up being a big deal. But it's hard to predict what will matter. For example, with the previous dev day, I thought Custom GPTs sounded stupid and that I'd use the Assistant API a lot. But it turned out the opposite --- half of our organization is using ChatGPT licenses and over 100 Custom GPTs, while we barely use the Assistant API.
[00:03:47] Raza Habib: Yeah, it's always tricky to predict how these tools will be adopted.
[00:03:51] Peter Gostev: Exactly, and now with the real-time voice API, I really want to try it. Automating call centers is boring, but the cost is quite high --- 200 per million tokens out. It's still expensive compared to salaries in countries like the Philippines or India.
[00:05:04] Raza Habib: True, but the cost will probably decrease over time as the APIs improve. Plus, you can scale your AI team up or down with seasonality, which is harder to do with people.
[00:05:48] Peter Gostev: Yeah, and we've done some projects at Moonpig for customer service. We automated a small process and augmented a larger one with an app that helps agents write better and fill in gaps in templates. We could have automated more, but the input documentation wasn't suitable, so we focused on something simpler and maintainable.
[00:07:45] Raza Habib: Did you try using the vision APIs to deal with that documentation?
[00:07:50] Peter Gostev: It wasn't about the vision part. The models just needed far more context to understand how to use the documentation, which was written for humans.
[00:08:38] Raza Habib: That makes sense. But it's still impressive that you managed to create value with a few developers over a few weeks.
[00:10:38] Peter Gostev: Yeah, we saw about a 15-20% improvement in speed for some agents, but I don't want to overstate that. It's hard to measure since agents don't always have tickets to respond to.
[00:11:45] Raza Habib: One of the reasons I wanted to chat with you is because you're an avid personal user of these tools. What have you found genuinely useful in your day-to-day life?
[00:12:01] Peter Gostev: I think productivity is a slightly boring way of looking at it. I don't feel more productive in terms of doing the same tasks faster, but I'm doing completely different things I would have never attempted before. For example, I built a prototype in one evening using Replit, LLMs, and synthetic data that I demonstrated the next day --- something I would've never done before.
[00:13:14] Raza Habib: Yeah, as the models get better, people who aren't full software engineers can get involved in development more easily.
[00:13:54] Peter Gostev: Exactly. At Moonpig, the people who have been really successful with LLMs aren't all engineers. It's more about curiosity and sticking to it, rather than technical skills.
[00:14:47] Raza Habib: That makes a lot of sense. Moving on, you led AI strategy at NatWest, a large, regulated bank. What was that like? What was the reception to AI technologies?
[00:15:14] Peter Gostev: It was interesting. The innovation team I was part of grew from about 10 to almost 100 people. We had strong senior leadership pushing for it, which made a huge difference. But it still took us six months just to get access to OpenAI.
[00:16:33] Raza Habib: What projects did you work on, and did you see barriers to production?
[00:17:06] Peter Gostev: We did several prototypes, like using RAG for HR policies, but getting anything into production was tough. Every step took months --- from getting stakeholder time to deploying anything.
[00:18:57] Raza Habib: Why was deployment so hard?
[00:19:00] Peter Gostev: We didn't have a clear pattern for deployment. It wasn't just red tape, but coordination. The teams needed to deploy were often busy for months.
[00:21:22] Raza Habib: Now you're at Moonpig, where things move faster. Have any of your AI projects had a meaningful revenue impact?
[00:21:34] Peter Gostev: It's easier to get things done here. We've got half the company using ChatGPT and have deployed small projects like greeting card tagging. Some cost savings, but we're not quite at meaningful revenue impact yet.
[00:24:34] Raza Habib: Based on your experience at both large enterprises and smaller startups, what advice would you give other AI leaders about developing their own strategies?
[00:24:59] Peter Gostev: First, I think it's important to just play with the models. Many people don't spend enough time understanding what works and what doesn't. You need that intuition to spot opportunities. Second, I think it's important to have a portfolio of projects --- some quick wins that show value and some bigger, more experimental ones.
[00:28:10] Raza Habib: That makes a lot of sense. Final question: Is there anything you've seen recently in AI that's exciting but underappreciated?
[00:29:03] Peter Gostev: I think OR1 models are interesting, though I'm still trying to get a better feel for them. The idea that you can spend more compute to get better answers is exciting, but it's still early.
[00:32:21] Raza Habib: Yeah, and I think people didn't update their expectations for the rate of change post-ChatGPT. OR1 and test-time compute are promising new avenues for progress.
[00:34:16] Peter Gostev: Agreed. I think we've trained ourselves to spoon-feed these models, but with OR1, you can give it more context and higher-level reasoning.
[00:36:38] Raza Habib: Exactly
[00:36:40] Peter Gostev: Right. And I think that's where we'll see some really interesting applications. As these models get better at handling more complex, nuanced tasks, we'll be able to offload more high-level thinking to them.
[00:36:55] Raza Habib: Absolutely. It's an exciting time to be in this field. Peter, as we wrap up, do you have any final thoughts or advice for our listeners who are working on AI projects or thinking about getting started?
[00:37:08] Peter Gostev: I'd say the most important thing is to just dive in and start experimenting. Don't wait for the perfect project or the perfect model. Start small, learn from your experiences, and iterate. And don't be afraid to share what you're learning with others. The field is moving so fast that we all benefit from sharing our insights and challenges.
[00:37:30] Raza Habib: That's great advice. Peter, thank you so much for sharing your insights and experiences with us today. It's been a fascinating conversation.
[00:37:38] Peter Gostev: Thank you, Raza. It's been a pleasure.
[00:37:41] Raza Habib: And that's it for this episode of High Agency. I'm Raza Habib, and we've been talking with Peter Gostev, head of AI at Moonpig. If you enjoyed this episode, please subscribe to the podcast and leave us a review. Until next time, keep building and stay curious.
[00:37:58] [Outro music fades in and out]
About the author
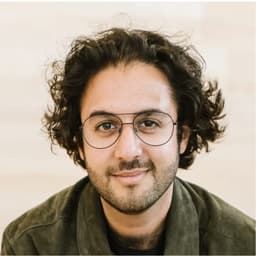
- 𝕏@RazRazcle
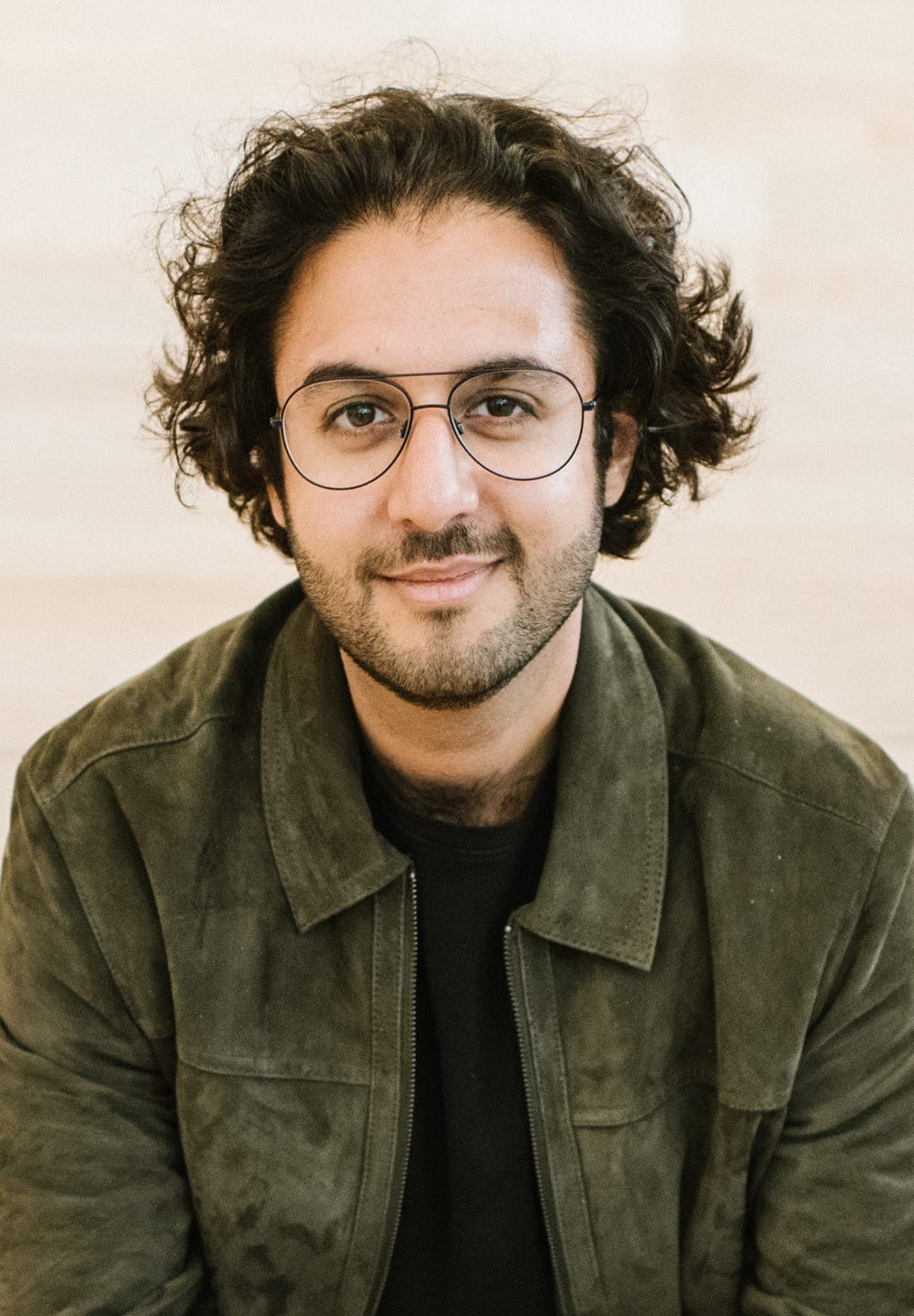
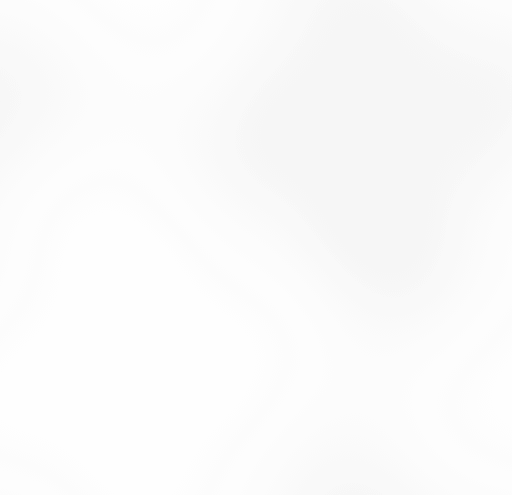
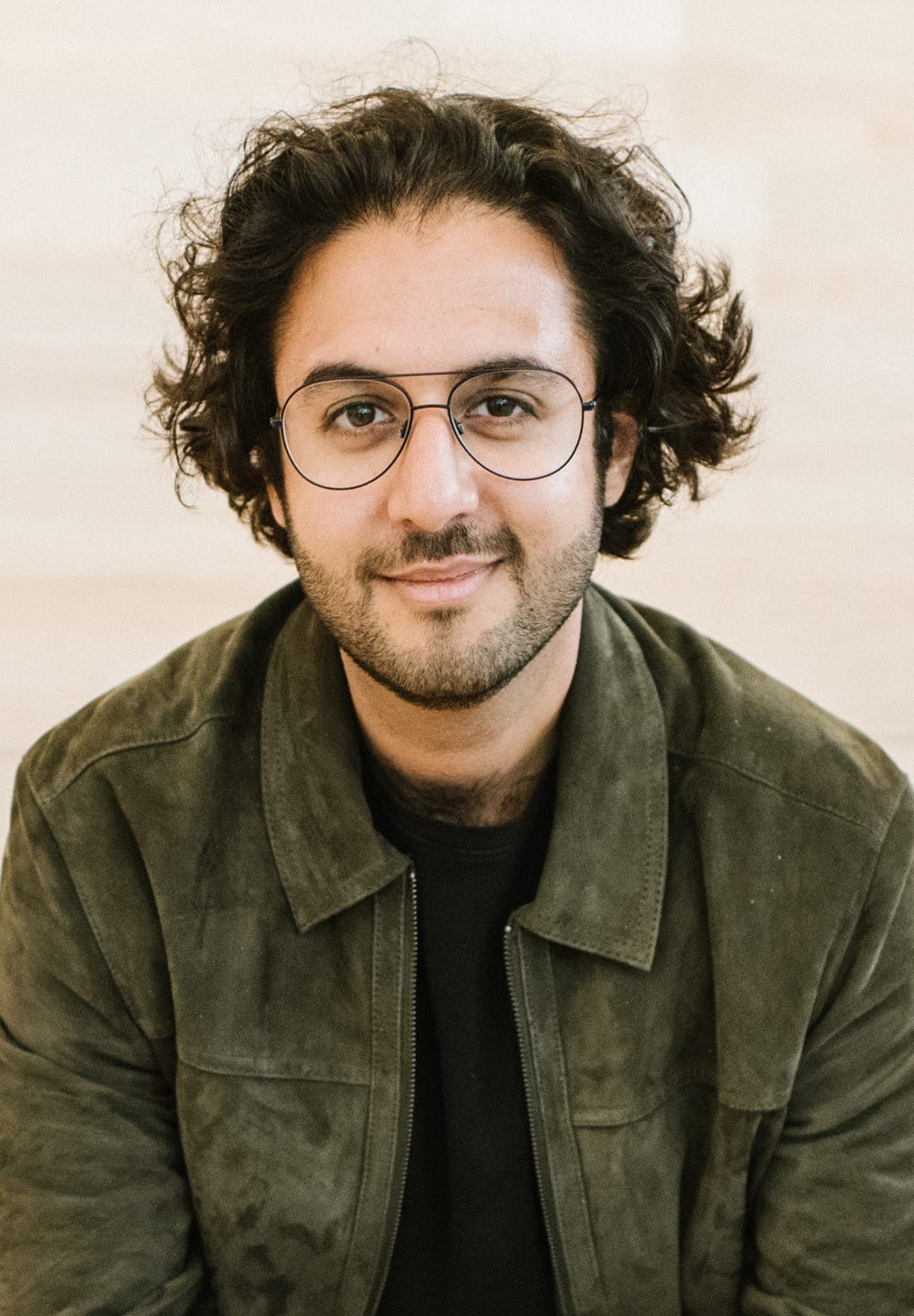