What is beyond OpenAI?
Logan led developer relations at OpenAI before leading product on the Google AI Studio. He's been closer than anyone to developers building with LLMs and has seen behind the curtain at two frontier labs. In this week’s episode of the High Agency Podcast, Logan and I discuss the future trajectory of AI and share our insights on what AI builders can anticipate in the future. We cover a range of topics from joining OpenAI the day ChatGPT hit 1 million users, what you might expect from GPT5, Google’s latest innovations and how you can stay ahead and achieve real ROI.
Subscribe to Humanloop’s new podcast, High Agency, on YouTube, Spotify, or Apple Podcasts
Here are some key takeaways for AI builders from our discussion.
1. Bet big on AI for Massive ROI
Identify core areas in your product where AI can have the biggest impact and allocate significant resources to develop and integrate AI solutions. Don’t be afraid to make bold investments.
"There is an exponential opportunity for people who are building with AI at the core of their product, vs. people who are just building AI features." - Logan Kilpatrick, Sr. Product Manager, Google AI Studio
2. AI Systems are more important than models
Success lies in building systems around AI models that make use of tools, context and agents. Devin is a good example of a step change in performance without a new model.
"The way that we will end up seeing a step change [in AI scale and adoption] is with people building systems," Kilpatrick said. "A good example is the Devin demo... There was nothing special happening from a modeling perspective, but [Devin] was a thoughtfully designed system presented in a way that solved a bunch of friction points that software engineers have."
3. Google's Gemini Suite
Innovations like the 2 million token context length and caching are redefining AI capabilities. Logan thinks the battle with OpenAI is just getting started and that Google’s infrastructure will allow them to compete aggressively on cost.
"What actually matters to developers is the utility function per token cost,” Kilpatrick said. “It feels like [Google's] 1 .5 Flash is a winner of utility-to-token costs, given that it's cheaper than GPT-4o, natively multimodal, and has all those same frontier capabilities that 1 .5 Pro has with caching, code execution, etc."
4. Future of AI
Logan shared his predictions for how fast the models will improve and what new capabilities we can expect when.
"We're giving builders the freedom to build and taking away the cost constraints," Kilpatrick said. "This is one of the unique advantages Google has from a scale perspective."
This conversation was rich with insights. I hope you enjoy it.
Chapters
00:00 - Introduction
01:50 - OpenAI and the Release of ChatGPT
07:43 - Characteristics of Successful AI Products and Teams
10:00 - The Rate of Change in AI
12:22 - The Future of AI and the Role of Systems
13:47 - ROI in AI and Challenges with Cost
18:07 - Advice for Builders and the Potential of Fine-Tuning
20:52 - The Role of Prompt Engineering in AI Development
25:27 - The Current State of Gemini
34:07 - Future Form Factors of AI
39:34 - Challenges and Opportunities in Building AI Startups\
Highlights
"I think for people who had been at open AI for some period of time before that, they sort of knew this world outside the chaos."
"People who are betting big on AI being like a central or a core part of the product are ultimately the people who are likely going to be the beneficiaries of this wave of technology."
"You wouldn't expect more from a human, but we are expecting some sort of miraculous thing to happen from the model."
Podcast:
Raza (00:01) I'm joined today by Logan Kilpatrick, who's leading developer relations on Google's Gemini API, as well as the Google Studio, and was previously head of DevRel at OpenAI, is on the board of NumFocus, and has contributed massively to open source. So I'm really excited to chat to him. Logan, it's a pleasure to have you.
Logan Kilpatrick (00:18) Yeah, thank you. And my, my correction to the intro is I now lead product for AI studio. So I've transitioned out of like a formal dev rel role, which is what I was doing at open AI into a like pure product focus role. And I still get to do all the dev rel stuff that I love. And yeah.
Raza (00:33) fantastic. Yeah, I see you doing so much DevRel that I hadn't realized that you were doing product as well. It's a huge workload to have taken on.
Logan Kilpatrick (00:40) I think there's a bunch of dirty secrets for perhaps another podcast about like, you know, I think it feels like more and more, especially with developer products, especially in this age, like it feels like it would be hard to be a great product manager without also being like deep in dev rel and spending a lot of time with the community and understanding what people are building. So it's this perfect symbiosis between all these things. I love it.
Raza (01:03) That makes a lot of sense. I wanted to start by going back a little bit. So you joined OpenAI a little bit before ChatGPT came out. And so I was wondering if you could tell us a little bit about what was it like to be an OpenAI at that time before I think they were at the center of the eye of the storm. How did it change? Like what was the moment of ChatGPT coming out like within the company? What's the story there? And then finally, like how have you felt the company kind of grew after that? Would love to hear that story.
Logan Kilpatrick (01:33) Yeah. So I joined, I started interviewing at open AI back in June of 2022 for like a purely developer advocate type position. at the time. GPT3 was out, there was an API, they had some other of those building blocks in place, but the real challenge was essentially the only people who were using the API were the folks in the copywriting ecosystem. So this was the copy AI's the Jasper AI's at the world and they open AI sort of. At that time, GPT4 had actually already finished training and they sort of knew what was coming around the corner, but hadn't yet figured out like, what's the right way to externalize this technology. So, started that interview process ultimately, ultimately, actually they wanted someone in San Francisco and for some folks know this, some folks don't, I don't actually live in San Francisco and back and forth between Chicago and SF. so decided not to continue the interview process because I just moved back to Chicago to be here with my girlfriend.
They reached out some months later and like, hey, we're willing to hire someone remotely, would you still be interested? Finished the interview process by the time this actually happened. My first day at open AI was, early December of 2022. Chatgyp had actually already come out to my first day was like the news of chat GPT, hitting a million users. So a lot of my initial, that was day one day one was me was all of that craziness.
Raza (02:47) Wow.
That was day one.
Wow.
Logan Kilpatrick (03:00) I think for people who had been at open AI for some period of time before that, they sort of knew this world outside the chaos. And like for me, my world was always that chaos at open AI. It was like it from day one, it was like complete madness, complete, all the things that, that you could imagine. I think thinking back to the interview process, like the, the interesting thing was it was people knew what GPT -4 was capable of.
But I don't think to the degree that it ended up being like, everyone was like, like we can't show you this thing yet, but like it's, it's, you know, if you're impressed by what we have right now, this is like orders of magnitude better. And it was like hard to, at least it was for me, it was hard to extrapolate. Like, you know, I played with text of NG3 a bunch. How does, you know, what is Forex better than text of NG3 actually look like? And, so I don't think that I had a good mental model of this. I think obviously some folks who were using the technology more did.
But even once I joined, it wasn't super clear. We had all these clunky internal demos where you could try GPT -4. And I still think at that point, it wasn't super clear to me until...
Raza (04:12) It's funny you say this, because I remember very distinctly the first time I got to play with GPT -4. And it was a little bit before ChatGPT came out. I'd come on a trip to San Francisco and visit the OpenAI offices and kind of got one of these like sneak previews. And I think by that stage, I'd been using the models for long enough that I had this kind of like backlog of test cases that I'd built up. So every time someone gave me access to something, I would kind of like get out my favorite test cases and run them through.
I remember just like going through one by one, like each of them and being like, okay, well, it's beaten all of those now. I now need to kind of like think of something new. And so, so for me, at least it felt stark, like day and night, the difference from DevTex DaVinci 3 to GPT 4. And I guess very soon after you started that became apparent well when you guys launched GPT.
Logan Kilpatrick (04:59) Yeah. I think perhaps the missing link for me was like, I hadn't, you know, developed that mental model at that point for like, what, what sort of the limitations of the models were, what, what was going to be like, again, I had started using the models actually, like as part of my interview process, like I wasn't super, like I didn't try GPT two or GPT three before that. So I had a little bit less context. And I think this ultimately for me, the thing that ended up being super cool was ultimately how this technology has been impactful to the world is you put it in the hands of developers and they go and figure out all the problems that people have and they go and solve those problems in really interesting and novel ways. And I think once you sort of start to see what people are building, it's sort of this, at least for me, it was this combinatorial explosion of like different use cases. And I was like, okay, now I actually see this once you sort of start to see people building with the technology, which was super cool. And I think that ethos within OpenAI of know, developers ultimately being the mechanism to achieve the company's mission, I think was something that that resonated with me in the early days strongly.
Raza (06:04) And you said you can imagine what it would have been like, but I actually think it's hard for me to imagine what it would have been like in OpenAI in those days of ChatDBT. Help paint a picture for me, literally what was going on. Like you're all over the news, is the API going down? Like how are you keeping up with the load? Like what's going on? Like literally day to day. How big is the team for ChatDBT? I got the impression at the time it was very small. Like how is the company organizing around this moment?
Logan Kilpatrick (06:29) Yeah, I think the, so at the time there was the applied team and then there was the research team. So I think they still sort of have this breakdown, but it's like a little bit less pronounced now, but I think the research team was actually much larger than the, than the applied team. I remember we had an offsite probably within the first few months that I joined, open AI. And it was like, you know, there was 30 of us in a room. And it was like, these are all of the people focused on externalizing this technology from support to sales, to solutions engineers, to, you know, the API people. So it was a really, really small group of folks. I think that again, it's all, I think the thing that I look back on and, I would never have to doubt as to whether or not this thing was actually going to happen to like the highest degree that it was possible. And that's such a special thing.
It seems nearly impossible to be able to recreate an environment like that. So it's, yeah.
Raza (07:54) It sounds like it must have been an extraordinary special, special place to be. And I guess since then, ecosystems grown, you've now worked probably with hundreds of companies, both across Google and OpenAI, and you're deeply embedded within the developer community. And so I wanted to pick your brains on what distinguishes the companies that really build successful AI products and the AI teams that are getting the most ROI from the ones that don't.
I have some specific questions, but maybe the general one first. Like in your mind, what are the characteristics or the skills or how to think about things that really help builders succeed in this space?
Logan Kilpatrick (08:32) Yeah, this is a this is a tough one, because I think it really depends on where you are as a company. I think I'll tell you that I my instinct and I think there's a you know, I can I won't share all of the data and sort of what drives this instinct. But people who are betting big on AI being like a central or a core part of the product are ultimately the people who are likely going to be the beneficiaries of this wave of technology. I think like there's a lot of instinct and this is like the traditional company slash human instinct of like, okay, let's just sort of find a small little use case for this thing and then maybe expand it, et cetera, et cetera. The people who are going to win are going to be the people who are betting the entire ship and the boat on this technology like actually dramatically changing the entire outcome and the trajectory of their company. And I think you see a really stark difference and there's a whole class of companies and I see this now like independent of my builder hat, but just like as a consumer of these of these products, but like, you know, the whole class of people who like super humans, a good example, like superhuman is, is betting the boat on AI plus email being this thing that is going to like fundamentally change the arc of how people interact with email. And you look at that versus people who are perhaps taking a less, a more conservative approach. And I think it's like very clear to me, at least from a consumer experience perspective, like what is providing more value today? And there's like, across every other vertical, you see very, very similar use cases of people who are like building AI features versus betting everything on AI. And like my...my instinct from seeing all the trends and the trajectories of these companies is I would be betting on the people who are betting everything on AI. Because this is just such an exponential that it's not clear that people who aren't seeing it as that order of magnitude of opportunity, who are just building features, are going to actually benefit from the exponential.
Raza (10:39) You're closer to it than most, and you're seeing behind the curtain in a couple of top frontier labs. People outside of the space who are less close to it, I think maybe don't have as good an intuition for how fast the rate of change both has been in the past and might be in the future. Especially because at least from the outside, these training runs are very long, there's a lot of noise constantly, and so it's easy for it to feel like...maybe there hasn't been a huge improvement for a long period of time, or maybe things are plateauing. You know, someone who is skeptical of what's coming, what might you tell them based on what you're allowed to share that, you know, would maybe change their mind?
Logan Kilpatrick (11:19) Yeah. One, I have a lot of empathy for that because like, I feel this as well. Like I feel the, the like anxiousness. I'm like, wait, we've had, you know, GPT four class models for, you know, coming up on almost two years now, probably like pretty close to the anniversary of two years from when that model finished training. And like, doesn't feel like the world has sort of taken a major step function from that original GPT four model yet. I do think in a lot of ways it's you know, it's that saying of like, slowly than then suddenly. And I think that that I find myself saying that so too much now. So I need to find a different a different phrase. I think that the perspective that I think a lot of folks are missing is like the initial hype and excitement around this technology, I think sort of glanced over too much how many like practical limitations there were on the technology at the time, whether that was, you know, if you were an open AI customer, like the rate limits that were being offered or the context windows or whatever it was. And I think you now see these like massive order of magnitude improvements along those axes, which like aren't actually correlated to the model capabilities, but are correlated to the impact that this technology can have. And I think there's like some amount of disconnect from, you know, you're seeing the hype on Twitter versus like you are actually someone who's practically building with this technology. And I think the people who are practically building with the technology even a year ago today have seen this order of magnitude increase in like what's actually possible along all of those axes. And you can throw in modalities in there. You can throw in the cost of running these services.
Raza (12:54) That, yeah. That definitely resonates, especially things like cost and modality. And also, I mean, I mean, obviously I believe this because that humanly we're building infrastructure around the models because we do think that without that infrastructure, we're not going to see the full potential of them. but I still wonder like, should people be expecting another step change? Like, like in addition to everything around the models, unlocking, you know, the unhobbling is as some people have called it recently. Okay. We've unhobbled a lot and that's given us a huge improvement.
Is your intuition that there'll also be another GPD four to five leap that's similar in magnitude as it was from three to four? Or does it feel like we need more new ideas and we're about to enter a period where it's more research dominated rather than just scale dominated?
Logan Kilpatrick (13:43) I think ultimately the way that we're going to see that leap is people building systems. Like it's not like the, the models are going to get, continue to sort of ramp up across, you know, many of the evals are already saturated, but they'll continue to make new evals. They'll sort of hill climb on those evals. Like that, that process will continue as part of like the normal research process. But I think really the way that we all end up seeing that step change is with people building systems. And I think a good example of this was the Devin demo. Like I think the Devin demo seemed like a step change in capabilities, but really it was a system like that, that model. There was nothing special happening from a modeling perspective, but it was a thoughtfully designed system presented in a way that sort of solved a bunch of the friction points that in that case, like software engineers had. And I think there'll be a lot more of those, like take the model, which is going to continue to get better, but also build the right system around it in order to like continue to see those like step changes happen.
Raza (14:44) Of the companies that are betting the ship, the ones that are gonna do extremely well, have you seen any particularly high ROI use cases or if I'm a product leader trying to think about multiple options and I'm trying to make a bet, like where have you seen people be really successful in not just like making the product good, but actually like realizing a genuine return on investment, getting actual money out of it.
Logan Kilpatrick (15:07) I think the ROI piece is still definitely challenging. I think like cost, the cost associated with running these models in production at scale. I tweeted something a few months ago, probably now, but I think there's this, there's this such strong tension for product builders and for folks and for product leaders as well who are grappling with, you know, end users have benefit so significantly. From an increase in the amount of tokens that you sort of spend on any given task to help them. But in the context of the company, there's this inherent tension of they need to minimize cost in order to keep their margins high and make money and have a business, which is very real. It's a very real constraint. And therefore, they're disincentivized from actually building AI into the product. And like not just know, a lot of people build AI into the product, but it's like the minimal amount of AI possible when really if they were to sort of three exit or four X the amount of AI that was being built in behind the scenes, like that actually could meaningfully change the product. So I think this, this tension point ultimately, and I think this is actually a sad outcome for the space. Like what's ended up happening is people who have a bunch of money and are willing to throw away a bunch of money on compute costs are able to build better products because they aren't bounded by the cost of running these things versus like your traditional indie hacker profile or just a regular developer off the street. Like they have these very real compute constraints to work within. And therefore like can't build the same product that somebody who has billions of dollars of VC money to burn. And I think there's a lot of things that the ecosystem needs to do to sort of offset those. Cause like ultimately like we're just getting less good products because of this, which I don't think is the best thing for the world. And I actually don't think it's the best thing for like ecosystem providers like y 'all and like others who sort of benefit from there being more extremely compelling products that people are building.
Raza (17:12) But it sounds like one of the takeaways from your experience has been that in order to realize the ROI, you have to be willing to take a big bet. So if you're nibbling and you're keeping your costs low and you're being careful, actually you don't see the ROI. So in some sense, what I'm hearing from you is like you have to be brave almost if you want to realize the value.
Logan Kilpatrick (17:33) Yeah. And I think it's like, there's, there's multiple paths to get there. It doesn't have to be that like, Hey, let's take our existing products and throw everything out the door and build something from scratch. But you should have that conversation and you should do that exploration. And maybe the outcome is like, we're going to build a different product. And I think a great example of this is the folks at Cora. Like Cora didn't take Poe and build Poe into the base.
the base Cora experience, like they went and built a different product because they were like, this is a huge opportunity. We have the engineering knowledge. We have the data advantage we have, et cetera, et cetera, whatever the rationale was. and that's a great example of like they've been successful. They monetize that product. They had many hundreds of thousands or millions of people using it on an act on an active basis. and I don't know if there's more, like I don't have any other top of mind examples other than them in that case, but
Raza (18:21) I can give a couple, I think. So we interviewed the CTO of Ironclad on this and he actually gave advice that was extremely similar to you. They really like bet the shop on it in terms of like going all in and embedding it very fully into their products. And he was saying that for some of their larger customers now, 50 % of their contracts are auto negotiated by AI. So, you know, seeing like very significant ROI and his advice was actually exactly the same as yours. He said, if you're not, you know, if you don't take a big swing now.
Then the risk of not doing it is probably bigger in his mind than the risk of doing it. I think Zapier are very similar. They've gone all in on this. A lot of founder led companies, you know, you mentioned Quora. I think it's easier if you are a founder led company to take those risks, which I guess makes some sense. Whilst we're sticking.
Logan Kilpatrick (19:08) I think Ironclad, Zapier, Cora, we're all at this also like unique intersection where, you know, I give them a lot of credit for being like taking the risk, but also like it's quite obvious in the case of like automation workflows that like AI has this incredible opportunity. So like it, like I wonder if there's some amount of like a company, fit to this, like I want to it being obvious that you should bet the boat on AI versus I have empathy for people where like, perhaps it's less clear right now because of whether it's the capabilities of the model or the cost of the use case or whatever it is of like, not feeling as compelled to be like, we should just go all in on AI at this moment.
Raza (19:50) Whilst we're still talking about advice you give people, is there any advice you find yourself repeating particularly often? You're speaking to developers, you're speaking to companies. Like what do you find yourself repeating a lot?
Logan Kilpatrick (20:00) Good question.
I think on the builder side of things, I still think a lot of folks are under appreciating the opportunity of fine tuning and actually going, the world that sort of has been promised by AI is like people having these personalized models. Which are able to like really understand them and, you know, sort of have empathy for their use cases and be there to try to help support them, et cetera, et cetera. And I think part of the only way of realizing that vision is to do fine tuning. I think there's some amount of Delta between like the tools and the understanding and the complexity of actually making fine tuning work from a use case perspective, which is why a lot of people don't do it. There's also like a, there's a huge cost burden. but I think that.
Raza (20:50) The other reason I commonly hear from people, because we also give the same advice that people should fine tune. And another objection that I hear, and I'd be curious to get your take on it, is just they don't want to be unable to switch as much. So they're still seeing a very fast rate of change in models and upgrading very frequently. And so it feels like fine tuning is slower to update, to changes, and kind of feels like you're investing more in something that's going to be static versus if you're prompt engineering lead. That feels easier to update quickly.
Logan Kilpatrick (21:21) Yeah. And this is, and, I'm hopeful that this isn't a shameless promotion of, of Gemini, but I think like part of this is like the, the reason that the rate of change is low is because the cost associated with, with refined tuning a model is significant. I actually think like, and it's a similar trajectory, like as the cost of tokens go down to zero, as the cost of like refined tuning goes down to zero, I think this changes like Gemini. We don't intentionally because of this, like we don't charge people money to retune the models. The inference costs are the same, whether it's a regular model or a fine tuned model. And I'm hopeful of that in some capacity, like, you know, puts pressure on other people or plants the seed for other people to be like, Hey, this is actually what is going to enable this technology to be more useful for people. And there's still a whole lot of economic upside for us as the providers of these models in that world where we do that. So I think that will that will help change this narrative. Cause I agree. I think that's like a very valid concern that people have. Like it's, it's a lot of work if you want to also refine tune the model as you switch providers or whatever the circumstances.
Raza (22:31) Yeah, and it's something that we try to help people with through the platform, but then they have to redo all their evaluation again. And so there's always a bunch of things that come with doing the fine tuning that I still think they're worth it. I think the advice we always give to people is like push the limits of prompt engineering to validate the use case and to really make sure it works. And then view fine tuning as an optimization step. You get more personalization, you can bring down cost, you can get tone of voice.
But it's still a subset, like it resonates what you're saying. It's a smaller subset of people that are reaping the benefits of fine tuning right now. And maybe whilst we're discussing this, you know, you run a podcast called Around the Prompt. You're telling people they should be fine tuning more. You know, what is your view on prompt engineering? Do you think it's going to stick around? Like how's it going to change as models get better?
Logan Kilpatrick (23:19) I think it's, it's surprised me how much alpha there still is in fine tuning. Like I think if you had asked, like my intuition has always been that fine tuning should just, or prompt engineering should just be replaced by an AI system. Like there is no reason to prompt engineer. Humans are so lazy. and, and again, it's been surprising that like so few products have done that. And like, it's actually like broadly picked up mainstream momentum.
The people who are training large language models are oftentimes they're not actually exposing to you with, I think, a few notable exceptions to this, how those models are being trained. Like you don't know what the input output data sets look like for these large language models. And ultimately that information, if the model hasn't been trained to see something the way that you're putting the prompt in, then like, you're not going to get the output that you expect, or it's not going to be as good as it could be. And given that the LLM providers like know what data is in the training set, it's, it's still confusing to me that there isn't like that you're not seeing. And I guess like part of this is because if you make all the prompts look like the training set, then it becomes a little bit more obvious what the training set was. So there's some amount of, disincentive to do that, but I think somebody's going to do this. And I think it's going to end up like, how Dali 3, for example, automatically rewrites your prompts to get it into a format that is sort of optimized for the way that the model is trained and takes the burden off. Because there's this, the natural tension is you need to give the model context. Humans are by nature lazy, and I don't want to give the model the context that it needs. And then I'm surprised when I don't get the output that it receives. But really, it's like the same outcome you would get from a human. If I say, Hey, go write me a blog post about LLMs. Like you're just going to take whatever knowledge you have about LLMs and like take a shot at it. And like it's, I think people sort of forget that you, you wouldn't expect more from a human and the, but we are expecting some sort of miraculous thing to happen from the model.
Raza (25:36) Can I run my take on this by you and see what you think? So my rough pitch with prompt engineering is I actually think it's gonna stick around and become more and more important over time. But I think that you can split prompt engineering into two types of things. There's like hacky tricks that people are doing to conform to the idiosyncrasies of the model and like the training data itself. And I feel like that stuff should obviously go away and fine tuning and more exposure to the training sets and just the model is getting better at following instructions, I think will obviate the need for that. But then there's a second part of it, which is what you were just talking about, which is, you know, there's no matter how smart the person is, there's no way to get a person to do what you want unless you give them clear instructions and articulate what it is that you're trying to get the model to do. And in so far as prompt engineering is going to be like clear instructions and defining tasks and essentially writing the spec. Like it seems to me that that should go up. Especially because the barriers to entry are going down. So the people who can be involved in this product managers and domain experts are able to come in and customize an AI product for a very new use case using the knowledge they have just by writing clearly and providing the relevant context. And that's very powerful and exposes, you know, when the, when the cost of something goes down, typically people consume more of it. And so my, my pitch is actually, I suspect that prompt engineering of the, of the second kind of like just clearly communicating what you want. Will rise in volume and importance over time. Curious if I'm missing something.
Logan Kilpatrick (27:05) I think that resonates. I think my, my sort of tangential comment to that is my, like, as these systems get more context on, on you and your life and your habits and et cetera, et cetera. I think my hope is like the first pass or the template or whatever it is, is going to be like embedded in that prompt so that I'm not going to have to like by default.
Raza (27:27) Yeah.
Logan Kilpatrick (27:30) Write out my six paragraph essay with like all this hitting on all the points. Like the tools will actually, or the model itself will actually like guide me through it getting just like, again, if I were to go to a smart teammate that I have and say, Hey, I need you to do this task. Like they're not just going to go and be like, okay, done. And then just go in front of it. If I give them two sentences of context, like they're actually going to reason and then come back to me and get the information that, that, that they need to be successful in the task.
Raza (27:56) Yeah, I think we're almost thinking about two slightly different audiences. Because I think for consumers, like the end consumer should never be writing prompts. And I completely agree with that. I guess I was thinking more about maybe the builder or the product developer who might need to bake that in for them. But yeah, I see that.
Logan Kilpatrick (28:12) Yeah. And I think like ultimately it'll probably be like tools like human loop that like help the builder persona sort of do that consumer flow as well, where they're, you know, building a better prompt along the way. And I'm sure that you're helping folks do that, which is, yeah, which is helpful.
Raza (28:29) So changing gears slightly, it felt like for a while Google was the sleeping giant in the AI race. Built the transformer, pushed the boundaries of deep burning, authors of TensorFlow, authors of Jax, but they weren't releasing a lot. And then post -Jax GPT, it feels like they got into the race in a big way, and this has become a focus for leadership. So it's almost hard to keep up with the amount of stuff that's being released right now. Can you give me a little overview of what's the current state of Gemini?
What's the stuff that you've released recently? And for people who have kind of defaulted towards OpenAI or Anthropic, what should they know about Gemini that they might not have considered?
Logan Kilpatrick (29:09) Yeah, this is, I think that this narrative is spot on to a certain degree. I think like Google was always making large order of magnitude bets on AI. I think they were just like, not the, you know, like Transformers had been a part of Google search for like, you know, since the transformer paper came out, it was just less of this like specific use case that I think has caught the world's attention. So I know there's a whole group of people inside of Google who have like been...productionizing transformers and this technology and they're all sitting in the back and be like, what do you mean we didn't ship?
Raza (29:41) Yeah, so, okay, that's definitely true, but I worked at Google AI in 2019 and I loved it, but there wasn't a sense of urgency to productize the research. It didn't feel like we were running towards getting stuff into the hands of customers. Like, and it feels like that has maybe changed.
Logan Kilpatrick (29:58) Yeah, and this, I think this is actually probably the most fundamental Delta is I think historically and this, you know, I'm not a part of these research teams. So take, take my perspective with a, with a grain of salt. And obviously I wasn't around for this, but I think like there was a bunch of orgs at Google that were doing like a bunch of like fundamental AI research and putting out a bunch of papers and stuff like that. But there was a much smaller group that was focused on like, how do we actually productionize this technology? And I think the combination of Google DeepMind becoming Google DeepMind and not just DeepMind and then Brain and Research all coming together has been this, at least for me as the beneficiary of this reorg now happening and then me getting to come into Google after it's already happened. It's actually been really incredible because to me, I see Google DeepMind as the thought partners for how we can productionize this technology. And everyone who I talk to, their goal is how do we put this hands in the technology of developers?
We want to see people build with this technology. We want to see it impactful, impact the world, which is, it makes my life easy. Cause I'm like, we got a whole bunch of incredibly smart people doing really interesting things who want to make those interesting things available to developers. And that's ultimately what I care about as well. so I think there's been a ton of, a ton of really differentiated innovation, in the last, that we've actually released in the last probably month, I think. So we just, last week rolled out 2 million contacts on 1 .5 Pro, which is just literally blows my mind every time I
Raza (31:31) Give people some intuition for what 2 million tokens is. Like how much data is that?
Logan Kilpatrick (31:35) It's to the point where you start to have a hard time in like a consumer use case, like finding enough tokens to put in. You need like a, it's like a two hour video, but where the model is not actually just looking at the transcript of the video, it's looking at the audio, it's looking at images from the video. It has all of that and the transcript and like literally two hours of like raw video. It equates roughly to like 2 million tokens.
Raza (32:08) And I haven't had a chance to push the limits of that context lent myself yet. What's happening to latencies and costs there? If I'm, if I'm filling that 2 million and a half token context lens, like, is it still usable? Is it still affordable?
Logan Kilpatrick (32:22) Yeah, it's, I mean, it's definitely more expensive. so you need to be thoughtful about the use case here. And this actually segues and I'll come on and I'll comment on this briefly in a second, to another innovation, which is context caching, which we can talk more about, but, latency does tend to go up. So I think on an order of like, you know, I was doing a couple of like 1 .2 million token requests with 1 .5 pro earlier this week. And I was seeing like a 52nd round trip.
Time, time to, I think it was like time to first token. Maybe it was the whole request. I forgot it in the exact example, but so it is like, you need to have a use case where you're latency tolerant. I think that actually starts, the narrative starts to change with context caching. So with context caching, we actually make it so that you can say, Hey, I have these tokens. I.
Normally would be paying every time, like you could take a long system message or a video. If you had like a chat with your docs app or a chat with your video doc. and normally the, the base flow for every developer using this technology today is. Stake a bunch of tokens in the context window. And every single time I send a request to the model, I need to pass those tokens back and I need to pay for those tokens every single time I pass them to the model. Context caching allows you to essentially do that input cost once and then pay a fixed storage cost. And it's on the order of like a few cents every hour to keep that essentially like hot filled in the system and waiting for you to sort of send additional tokens after that. And the model, it performs exactly the same. So if you were to have paid the whatever amount of dollars or cents to pass in those tokens, pass in the cash tokens plus the new tokens, you can still do all the same thing. So it really, I think this narrative of like giving builders freedom to build and taking away the cost constraints, I think is like one of the unique advantages Google has from a scale perspective, from a building large distributed infrastructure problems perspective, which gets me excited because I think like, again, there's always been an incentive for developers to be like, okay, how can I get rid of as many tokens as humanly possible, keep this as minimal as possible?
And for what it's worth, I don't always think that like there's some use cases where that makes sense, but that doesn't make sense all the time. so it's, it's exciting to see that people are now able to sort of start pushing those things.
Raza (34:51) Yeah, it's interesting. I feel like there's almost two different mindsets depending on the use case. Like I was speaking to the Brian Bischoff who leads AI at Hex and he was talking, you know, I was like very impressed by the latency of their agents. You interact with Hex and the product is super fast. And so I was like, are you guys fine tuning? Like you're using open source models. Like how are you achieving that low latency? And his answer was surprisingly simple, which is they're just brutal about stripping tokens from the context.
So they do everything they can to just make the context as short as possible. And that's how they achieve those really short latencies. So I can see opportunities where, hey, yeah, I want to analyze a two -hour video. And then having a massive context is amazing. And then I can also see places where you've got a complicated agent or something, and you're going to be trying to push it all the way down. Before we move on, anything else about the Gemini Suite that people should really think about or be aware of?
Logan Kilpatrick (35:41) Yeah. One other quick comment on caching, which is by default right now, we're not sort of shipping a significant latency improvement. That's something that's like very near term in the roadmap. Something that's that we're thinking about, like ultimately it should have a dramatic effect on the latency and go down from like multiple seconds to hopefully, you know, less than a couple of seconds, which is super exciting. And again, like removing the barriers to using large context. The other piece of this that I think has, has really resonated with developers as flash. I think this, you know, people are trying to find the right models that have the right capability, intelligence costs, latency, et cetera, trade -off. And it feels like from the traction that we're seeing, like flash has really struck that chord. Like I think, you know, GPT -4 .0 has a bunch of. You know, cutting edge state of the art capabilities. I think for a lot of use cases where.
Intelligence matters a lot. it's a great model to do that. Same thing with GPT, same, same thing with, Gemini 1 .5 pro, but I think there's something about, there's something special in the, in, in sort of that mix of trade -offs. And I was chatting with the LM SIS folks and commenting on the fact that I think it's like still hard for developers and builders to sort of articulate they feel about or like what their use case necessitates from all of those different dimensions in the trade off. And like the leaderboards are great because they're showing sort of frontier capabilities, but like what actually matters to developers is like the utility function per token cost. And it feels like 1 .5 flash is like the far in a way winner of the utility to token costs, given that it's like 10 X cheaper than GPT -4 -0 natively multimodal has all those same frontier capabilities that 1 .5 Pro has with caching, with code execution, et cetera. It's been really interesting to see that. And I think the, my intuition actually wouldn't have been that that was the case. So it's a nice, it's been a nice surprise that this, that it really does have those, those right trade -offs for developers.
Raza (37:57) Yeah, the, this kind of, I guess two things that stuck out to me. One is there's a huge difference I found between like leaderboard performance and application specific performance, because people are caring about, well, one just on a specific use case, those leaderboard things do shuffle around a little bit, depending on what it is, but also personality and tone of voice and these very subjective elements that are hard to pin down on a, if you're just evaluating the model, but become very clear when you're doing a specific use case seem to matter to people.
So we definitely see the like inversions occasionally of like which models people prefer depending on what they're applying it towards. At the top of your Twitter right now, there's a tweet that asks what the final form factor of AI will be. And you follow it up with a spoiler alert. It's not a chat bot, which is essentially screaming to have the question be asked, well, what do you think the final form factor will be? Or what do you think is wrong with chat bots? What do you think is going to be coming next, what should people be thinking about?
Logan Kilpatrick (38:57) Yeah. I think the, the, I think chat has, has actually like from a ROI for the world, been such an incredible, like there's a lot of use cases that are better suited by chat. And I think there's like a whole host of like, you know, data visualization things where you were going through some clunky UI where like, really you could just ask the question, let the model execute code, get the answer to your question. and, and, you know, the ideal UI sort of manifestation of that actually is chat. I think the thing, and this was prompted by, I was sitting back over the last couple of weeks thinking about like how the world has changed from new technological revolutions and different things that have happened. And I think there's been a lot of changes in previous iterations of these like technological innovations that have sort of manifested in the physical world in the sense that you can almost like see sort of the, the, the technology, like, you know, it's in a lot of cases, maybe like abstracted away from your like actual visual view, but like, really it's like hidden behind something. And it feels like to a certain extent that hasn't happened with AI yet. I think maybe like the mobile phones and you know, AI going on device, you could sort of make that argument, but really like, all of the AI as far as how it's manifested in the physical world is in a data center somewhere that you've never seen before that you'll never see because it's actually locked down and hidden from the rest of the world.
So yeah, and yeah, this was more so me just thinking out loud. I don't know if I know the right answer, but I have a feeling that I would be excited to see some sort of positive, like the, ultimately the hardest thing in the world is, is moving physical, physical atoms in, in the real world. Like moving bits around is, is actually an easy problem. Moving atoms is incredibly difficult. And I'm, I'm hopeful that there's, and whether it's, you know,
I don't think this being the final form of AI is humanoid robots necessarily, but I think like that use case, has an incredibly high ROI for the world because it actually helps with the moving of atoms. And I think that's just like, we're, we're the, and a separate tweet of I put out a few, a few weeks or months ago is, you know, we could have AGI right now, like AGI right now actually doesn't have the order of magnitude of impact on the world that people think.
Like all, you know, if I have AGI right now, like we're still rate limited by the progress of the world. Like there's a bunch of like software challenges that you can solve, which is great, but like that doesn't, that doesn't move bits in the air. That doesn't move atoms in the real world that moves that move, that move bits on my computer. And I think there's this.
Raza (41:45) Where do you think the rate limiting step would be? So you get AGI, what now becomes the rate limiting step?
Logan Kilpatrick (41:53) I think it's like, what do you do next? Like, okay, I have AGI. How's that going to help me build more houses? Like, it's not really super clear to me what, what that, you know, how that fixes it. Like how's AGI going to help me build a skyscraper? Like not really super clear to me that it's going to like help me accelerate the time to build a hundred story skyscraper from, you know, 10 years to one year, like maybe in helping with permitting applications or something, it'd be helpful. But like, I really think like,
The problem is still, there's a bunch of highly skilled humans who know how to physically move atoms around in a way that ends up resulting in a building.
Raza (42:32) I've heard similar arguments for science as well. And you kind of see the arguments a little bit both ways. So some people make the argument, hey, we get AGI or we get something that represents ASI. And suddenly we're going to get this like huge flourishing of breakthroughs in science and big things in physics and chemistry, et cetera. And I can kind of see the argument a little bit, right? We've seen from DeepMind a bunch of stuff around AlphaFold and other breakthroughs. I've got some friends who run a company that are doing materials you know, research and they're using generative models to guide that and drug discovery. But I also have seen a lot of people point out, hey, the rate lending step is often the experiments, right? Like actually you got to go build the super collider or you got to go, you know, pipette some stuff and wait for the experiment to happen. And some things maybe we can just do in simulation, right? Like the models are, have solved protein stuff in simulation, but I can also definitely see an argument that the rate lending step moves to doing things in the physical world, which is interesting.
Logan Kilpatrick (43:27) And that's why, yeah, I completely agree with you. And I think like Leah pulled his comment on Darwesh's podcast about like the acceleration of AI research, I think is a good example because like the test bed for AI research is deep learning. And like the deep learning stack is with the exception of the hardware that it's required to run on like purely digital. Like you could parallelize a hundred million if you had the GPU sitting around different deep learning experiments. And even if you just randomly change stuff. Like there's probably some stuff that results in something like relatively novel and that's not rate limited by again, assuming computes not your limit. So I think there's a whole, all of those digital applications.
Raza (44:09) Although if there was one takeaway from that podcast, it was that compute will very quickly become your rate limiting step.
Logan Kilpatrick (44:15) Very true. And like, again, it's not clear, like if we have AGI today, how's that going to help me build more data centers? Like maybe again, it's, you know, efficient, it's like an efficiency gain, but it doesn't seem like it fundamentally changes the construct of, you know, what it means to be human or how quickly we're actually able to solve these problems. Like maybe you can use AGI to 20 % increase the management of construction worker efficiency on a highway project, but like, you know, is that actually going to impact my day to day life? Like, unlikely, no, like it's, it's, it's really not. I'll just have to hopefully sit in a little bit less construction traffic, you know, a month out of the year or something like that.
Raza (44:55) All right, final question before we break. Another tweet that you made maybe a little while ago, I've been following your Twitter, was that you feel a very strong urge to go and make an AI startup right now. And you said that this feeling occurs to you maybe every few months and the pull that is difficult to resist presently. So I guess a two -part question. One, what kinds of startups are attracting you? When you're thinking about this, what problems would you be going and working on?
Why are you so pulled by it right now?
Logan Kilpatrick (45:27) There's many different dimensions to this question. I think one, I have a lot of appreciation for what types of problems Google is able to solve well. I think with the skill set or with the capacity that Google have, comes all of the design constraint problems that just make solving a problem more difficult than you would.
Raza (45:29) Hahaha
Logan Kilpatrick (45:55) More difficult of a situation than you would have oftentimes in a startup capacity. It's like, it's, you know, you choose your pain, you can have your pain and, you know, this design constraint problem, or you can have your pain of, you know, not having resources and, you know, clawing at the ground zero and not having anything. And like, it's a different feeling of pain.
But, but I think that, you know, again, it gives me a renewed appreciation for like how startup founders like really do have this green field, especially right now in this AI space of like, there's so many green fields to go and solve these really interesting problems and get to think about it from a new perspective without any consideration for how somebody has solved the problem before, or what the systems they've used to solve it before. And like that's, that's oftentimes not a luxury that you get at any large company because
There's systems that have been built to help you do your things better, which are oftentimes like things that constrain you as well. so I think that's part of it from, from my thinking, I think the. My, and part of the reason why I didn't start a startup when I left open AI was like the thing that gets me excited about is building things for developers. And, outside of all of my official job capacities, like do a ton of investing in companies. And it's often in companies that are solving these developer problems for people. And.
It became this weird situation where like all of the really interesting, hard problems that I care about in the developer space, like are being solved by people who I've invested in their companies. And then it's like, okay, I can make a company and then go directly compete against people who I had enough conviction to invest money into their company because I thought they could solve this problem. And it becomes this like weird, situation for, for me personally. So, and on the other hand, like, there's a huge application. You know, I was talking to one of the venture, one of the popular venture firms and the comment was like their consumer team had only made a single investment because no AI applications had yet with the exception of the one that they invested in, none of them had hit the like thresholds for what it would mean to have a successful consumer company where they'd be willing to invest their money into that company. So there's huge, I think there's like, we have yet to see most of the value created in the consumer space. But again, like my, you know, it's, there's, you know, people say the like founder, market problem fit, or whatever the whatever the saying is, like, I'm not, you know, I haven't built consumer companies, I don't think about those problems all day, I really think about developer problems all day. So the only thing that
Yeah. A bunch of stuff around fine tuning is, is what I basically came down to. And it was just a question of like, did I want to go down that route? And I think part of the reason I didn't make that decision at the time was the economics of fine tuning. I think we're still pretty limiting. And I think now if I were, have been in the same situation, seeing the economics of, what's possible fine tuning Gemini models and not having to actually pay the training costs or increase inference costs, it actually makes.
Some of the ideas that I was kicking around like much more feasible. So like, this is like a very personal example of like what happens when you continue to accelerate this technology and like take the barriers down. Like my idea went from like not being financially feasible to like actually being financially feasible because I could now run on the order of many hundreds of thousands of like individual fine tune models, which is just like super cool. And I'm
It gives me a lot of motivation staying at Google to like continue to solve those problems where we can continue to pull down the barriers for people who want to solve problems right now. But like the technology is just slightly out of reach for, for whatever that use cases.
Raza (49:38) Yeah, and I've seen from the online reaction when you left OpenAI and then when you joined Google that there's a huge community of people who are very grateful for the work you're doing and clearly pleased about it. So your work has definitely built you a legion of admirers. Logan, I've really enjoyed the conversation. Thank you so much for taking the time to chat with us. And hopefully we can get you on again sometime again soon.
Logan Kilpatrick (50:03) Yeah, this was a ton of fun. Thank you for having me.
Raza (50:05) Thank you.
About the author
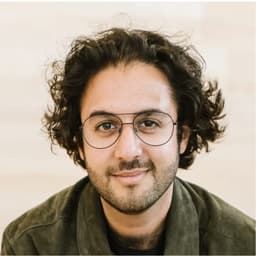
- 𝕏@RazRazcle
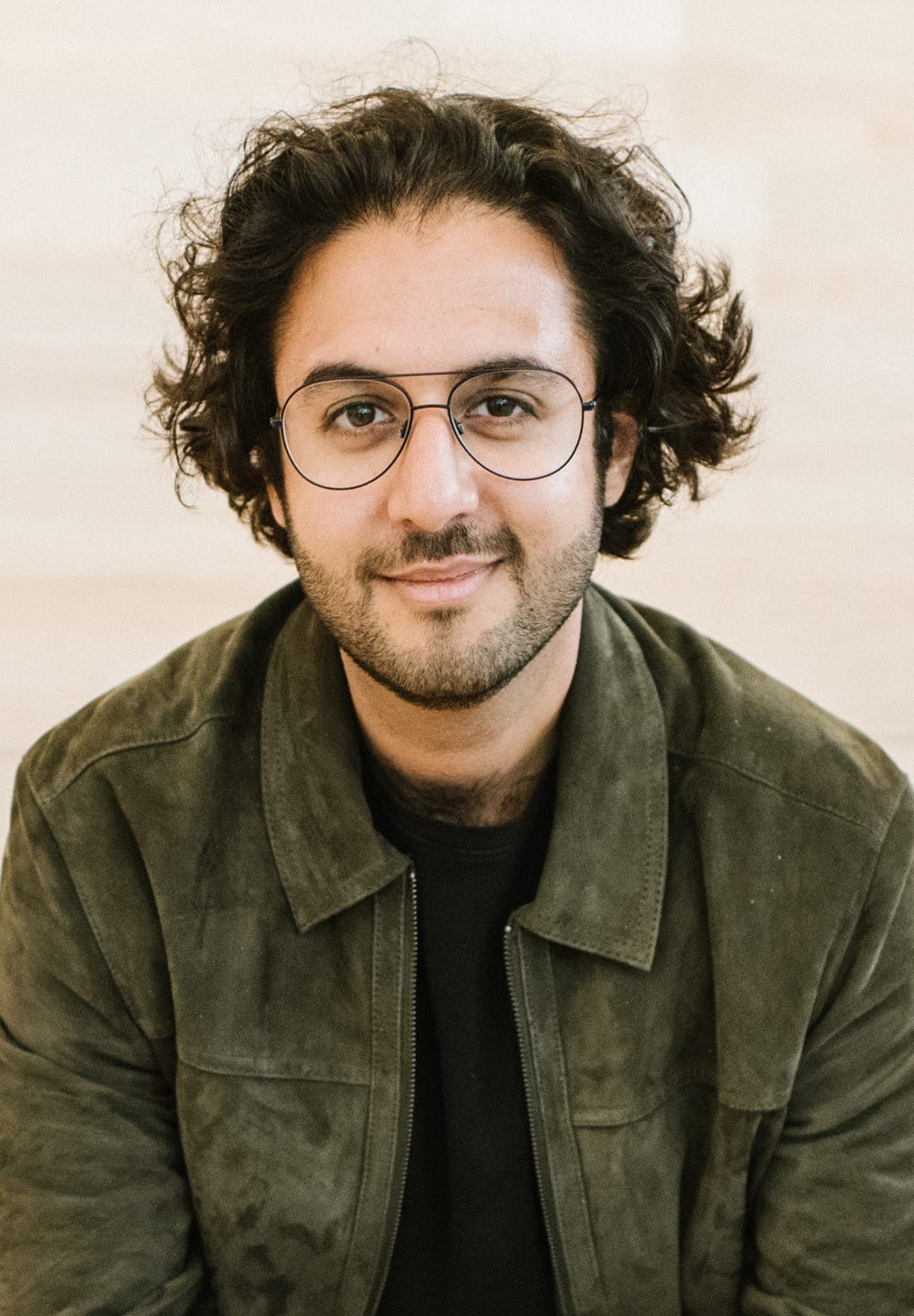
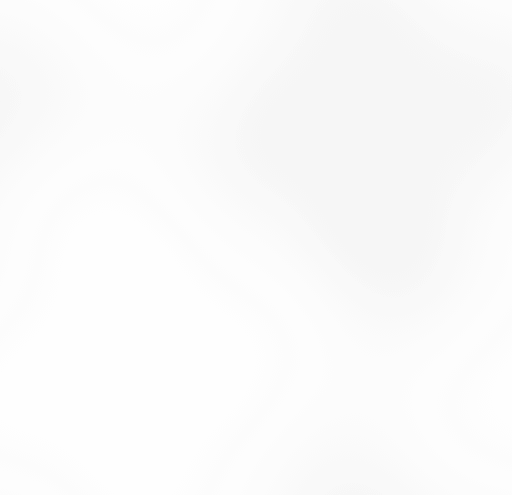
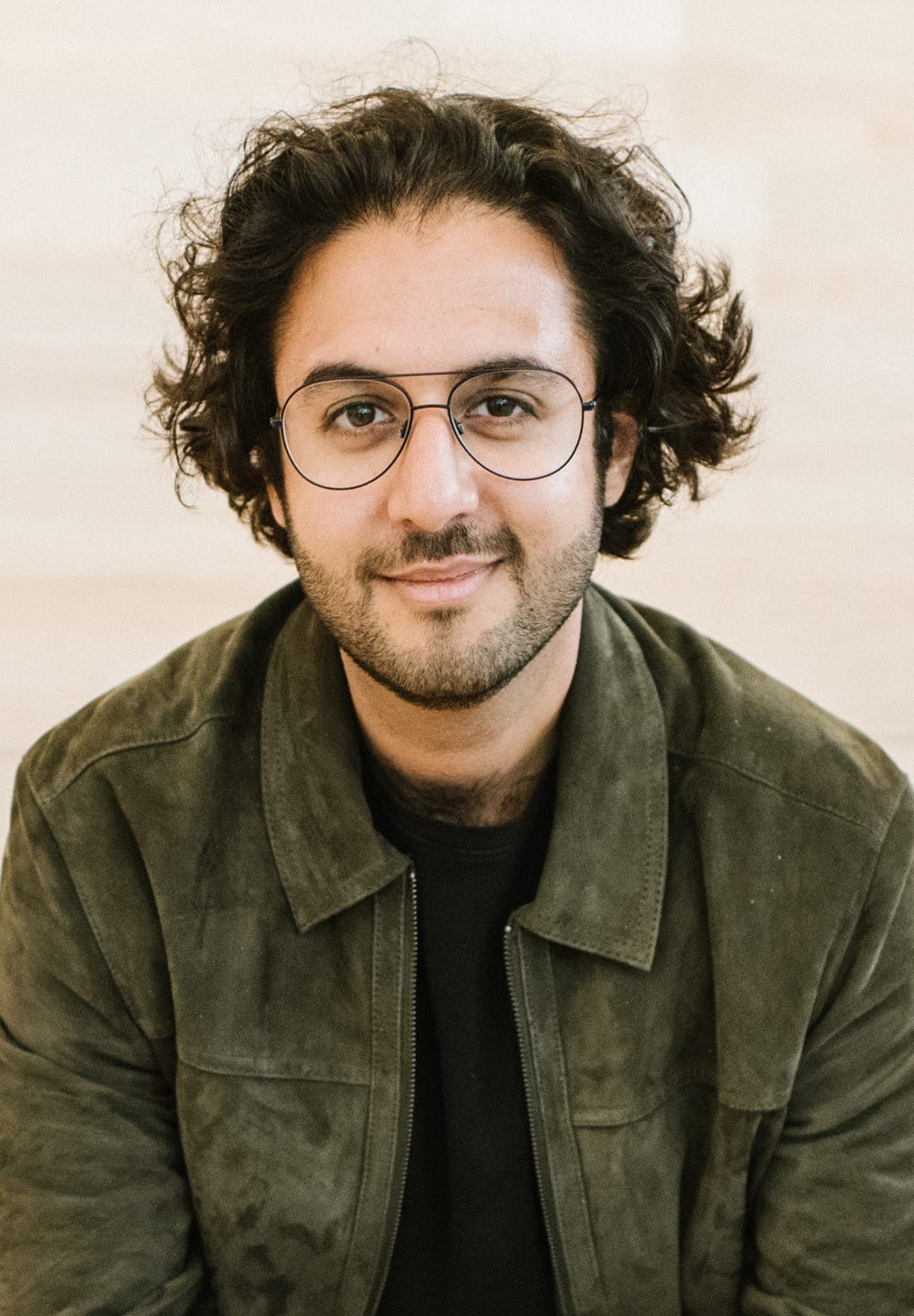