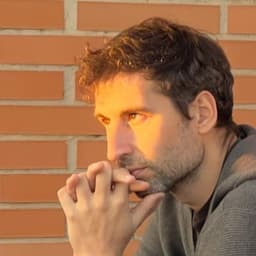
Daniele Alfarone
Sr. Director of Engineering at Dixa
3x
faster product release cadence
100%
visibility into AI product performance
10 hrs
saved across product, engineering, and ML per week
faster product release cadence
visibility into AI product performance
saved across product, engineering, and ML per week
Transitioning from traditional ML to LLM foundation models
Building AI products with ChatGPT
When ChatGPT launched in 2022, Dixa immediately recognized it would be a game changer. They were already a leading customer support AI platform serving a large client base and LLMs could dramatically accelerate their AI product roadmap.
Prior to the ChatGPT launch, Dixa made a $43 million investment into bolstering their toolkit, enabling them to utilize data on a new scale for automation, measurement and advanced analytics.
These upgrades poised Dixa for rapid product development and TAM expansion. However, despite their technology investments, Dixa was still stuck in the research and prototyping phase of their AI product roadmap and hitting deployment roadblocks due to resource constraints.

Avoiding AI production bottlenecks
Much of the workload was falling on Dixa’s product teams to develop AI features using more traditional machine learning methods that required large volumes of training data and many iterations of fine-tuning. When OpenAI opened up their first model APIs in November 2022, the Dixa team realized they could build AI capabilities much faster and at a fraction of the cost and investment compared to traditional ML approaches.

There was no question new proprietary LLMs like OpenAI could help the Dixa team accelerate AI feature production, but they knew development was only half the battle. They needed to ensure Dixa products maintained the same accuracy and reliability the company was known for.
Maintaining Trust and AI Governance
Dixa faced two major concerns when building AI features:
-
GDPR Compliance - Since Dixa has a large customer base in the EU, they needed assurance that all data ingested and generated must be secure, protect publicly identifiable information, and be compliant with the latest GDPR regulations.
-
High-expectations from customers - Dixa powers hundreds of millions of high quality conversations for brands across the world, that are known for their excellent customer experience.When layering in more AI automation and text-generation, the product team knew that accuracy and customization needed to to be top-tier in order to maintain the high customer service standards its client base was known for.
Dixa immediately began searching for LLM Evals platforms to help them scale their AI efforts. They started experimenting with the ChatGPT playground to test various features and use cases, such as summarization, translation and answer recommendations they could incorporate into existing products. This rapid prototyping demonstrated the potential of GPT-based features and the need to expand their capabilities.
Considering build vs. buy for LLM Evals
Dixa briefly considered a build vs. buy approach, but scoping out the resource requirements revealed this would be too much overhead for their 40+ person engineering team.
Dixa concluded that having a single source of truth to evaluate, track, and benchmark LLM prompt performance was the critical missing element to get ChatGPT models live within their product environment.
Leveraging the Humanloop LLM Evals Platform to Scale GenAI Adoption and Accelerate Production
Creating a single source of truth for prompt management
Dixa ultimately chose Humanloop as their single source of truth for all LLMops, prompt management, and evaluations to ensure scalability and quick implementation of AI features.

Evaluating LLM Outputs
Evaluating prompt outputs was the biggest resource constraint on the Dixa AI team because it required collaboration across multiple stakeholder groups such as machine learning, product, and engineering. Humanloop won against competitive options due to its user-friendly interface that made cross-team collaboration, and therefore LLM adoption easier.
Product Managers and domain experts favored Humanloop because they could easily change prompts and complete human evaluations without touching the codebase.
Engineering stakeholders valued Humanloop because prototyping and rapid deployment was easier thanks to Humanloops extensive API endpoints.

Since many of Dixa’s AI features were new, they were also experimenting with pricing levers, so they needed observability to ensure they were pricing their new AI products within scope of their backend compute costs.
Establishing LLM observability best practices
Dixa leveraged Humanloop observability features to help them monitor, manage, and optimize their AI features, ensuring reliable performance and continuous improvement.

Dixa combined Humanloop observability metrics with internal engineering metrics to create a custom Grafana dashboard that helped them monitor the following:
-
Compute Cost Monitoring: Track and analyze compute costs associated with AI model usage to manage and optimize expenses.
-
Application Error Monitoring & Alerting: Detect and troubleshoot errors in API calls and other application issues, ensuring reliable performance and quick troubleshooting.
-
Performance Metric Thresholds: Configure alerts based on specific metrics, such as high resource consumption or degraded performance, to proactively address potential issues.
Rapidly prototyping AI apps into production
In addition to enhancing observability, Humanloop helped Dixa's product team rapidly integrate LLMs into AI feature development. By bringing all LLMs and prompts onto the Humanloop platform, Dixa's AI product teams could compare best practices for prompting, evaluations, and accuracy standards.
To drive adoption, Daniele organized weekly demo days where teams showcased new experiments, refined prompts for optimal output, and shared learnings. He maintained a leaderboard to gamify the prototyping process, which accelerated team learning and engagement.
These initiatives, combined with Humanloop’s intuitive UI, helped the team adopt and scale an iterative prompt management cadence that helped them ship products faster and distribute workloads across multiple teams.

Benchmarking LLM Performance
As Dixa shipped more AI products, LLM evaluations and comparisons became more important. With Humanloop. Dixa could easily compare the performance of different LLMs against predefined benchmarks, ensuring the best models were in use for specific tasks. The Dixa AI team did thorough regression testing to ensure new models do not introduce performance regressions and maintained high standards of quality.

Accelerating AI Product Development With a Modern Tech Stack
Improving customer experiences with AI
Customer support AI is an extremely crowded marketplace with many competitors, which means speed-to-market is a critical way Dixa differentiates itself.
Since Dixa began working with Humanloop, it has shipped 9 AI features to market, increasing product release velocity by 3X.

Now in production: RAG-powered chatbots & AI copilots
Dixa’s strategic pivot to GenAI product development has helped its customers improve customer service experiences across chat, social, email and voice channels.
In particular, Dixa’s launch of its RAG-powered chatbot Mim and its AI Copilot product suite has seen significant adoption, contributing to Dixa’s YoY revenue.
When it comes to measuring ROI, Daniele and his team quantifies success the following ways:
- Predictable AI Costs & Performance - Dixa leverages Humanloop to track model costs and performance in order to accurately price new AI solutions for its customer base.
- Prompt Accuracy - Dixa ensures AI product features meet or exceed a 95% accuracy rate.
- Engineering Team Efficiency - On average, Dixa product and engineering teams report saving 10 hours of time week over week thanks to the efficiencies gained in prompt management and evaluations.
In the near future, Daniele and the Dixa AI product team look forward to leveraging Humanloop to continue expanding its AI product suite to meet the demands of the fast-paced CX market.
To learn more about Humanloop, check out our docs and request a demo today.
100m+
Customer conversations processed
Models used
GPT 4o
GPT 3.5-turbo
Anthropic Claude
LLM Use Cases
Writing Assistant
RAG
Text summarization
Automatic categorization
Answer recommendation
Chatbots
Workflow automation
Quality Assurance
Topic extraction