Humanloop + Hugging Face 🤗 = the fastest way to build custom NLP
Get early access 👈
👉Start from over 500 pre-trained models and adapt them to your use-case
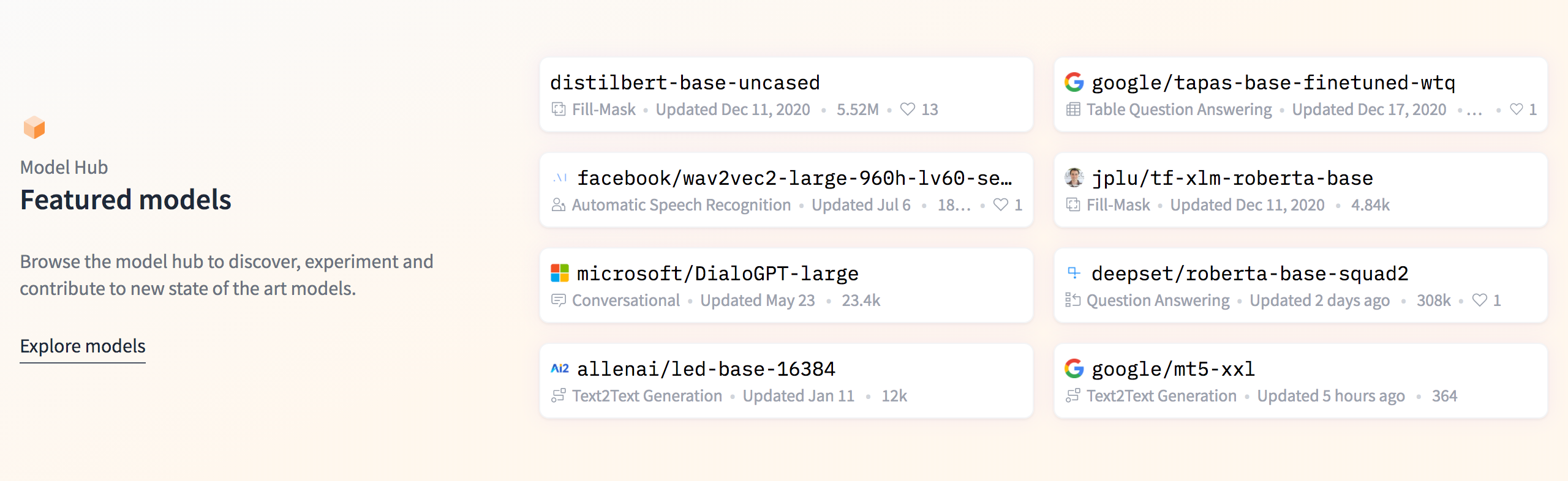
The Hugging Face transformers library makes it easier than ever to access state-of-the-art NLP models. The NLP community has pre-trained and uploaded hundreds of models to their hub. By combining Hugging Face models with the Humanloop platform, it's now possible to start from any of these models and specialize them to your data and your task through annotation.
Want to train a model for content moderation? Start from this hate-speech model and specialize it your dataset. Want to do sentiment analysis? In German? There's a model for that. Or start from scratch with your own data on a state-of-the-art architecture.
High quality labeled data is the biggest contributor to your model's performance
Data labeling is a neglected topic. While the machine learning community has produced fantastic libraries for modeling, hyperparameter search and monitoring, we regularly speak to many teams that are still using the same processes for data labeling — specifically Excel or rudimentary solutions quickly designed in house — that they were a few years ago. Academia barely discusses data labeling.
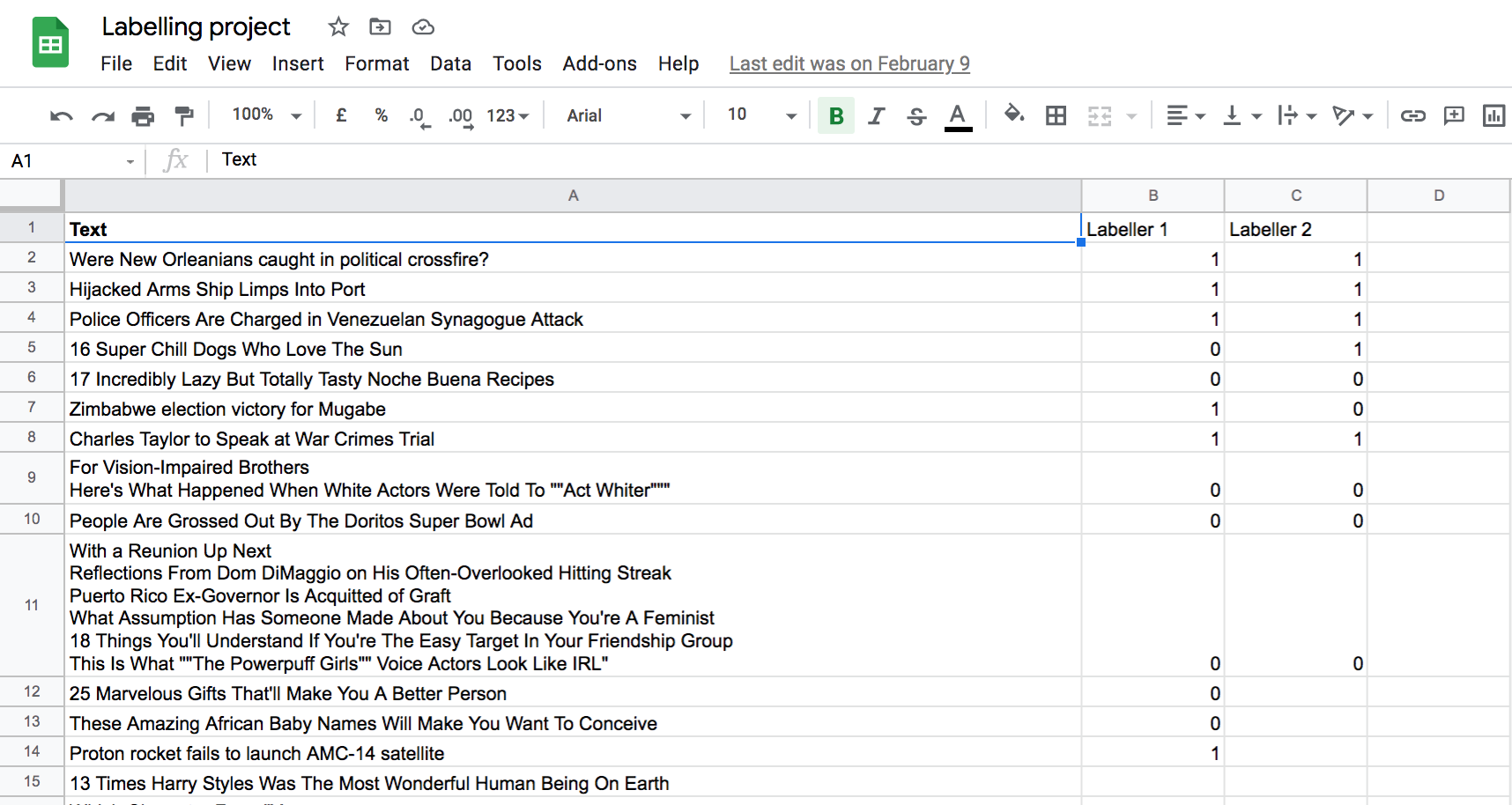
In practice though, the quality of your dataset likely has a bigger impact on model performance than most choices about model selection. As soon as you start labeling you're quickly faced with questions like:
- Which data is most important to label?
- How big a test set should you have?
- How do you make sure you get good label coverage on all your classes?
At Humanloop, we're applying the same level of research intensity to data labeling and selection that other teams have applied just to modeling. We provide labeling tools to quickly build your own custom datasets and use active learning to help you find only the most valuable data to label.
By automatically constructing test sets and providing quality assurance tools for teams of annotators, we let you improve your models by improving your data.
Go from unlabeled data to a live API fast
As you label your data, we'll train your model, construct a test set and give you estimates of the performance you'll likely get in production. When you're happy with your model, it's already hosted in an optimized runtime that you can start using immediately.
We believe that the combination of state-of-the-art models integrated directly into labeling workflows will make this the fastest way to go from idea to trained and deployed model.
If you would like to train your own NLP model sign up now for early access now.
About the author
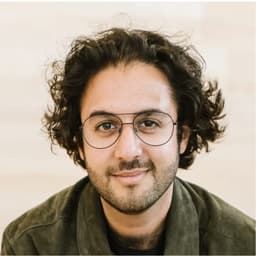
- 𝕏@RazRazcle
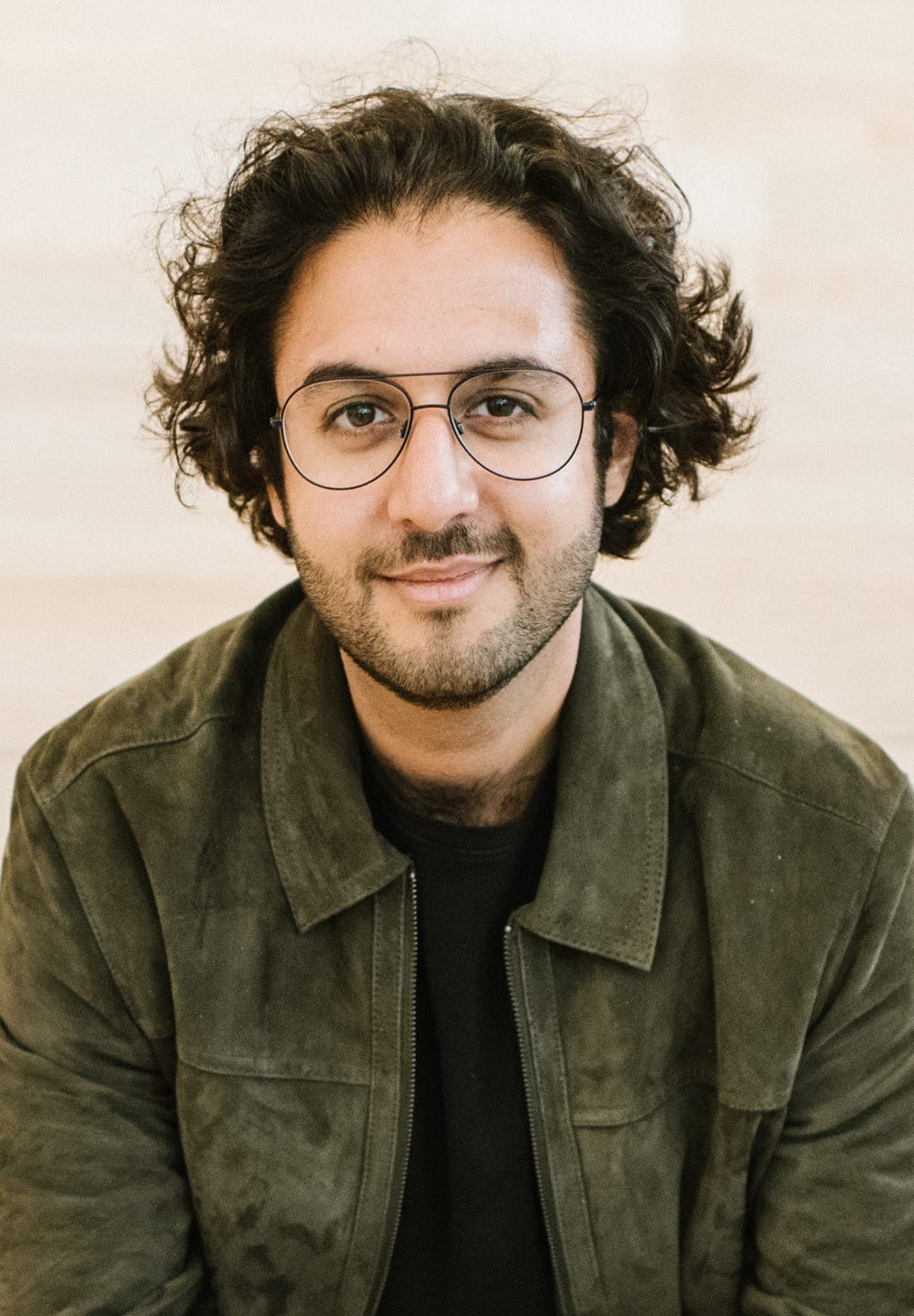
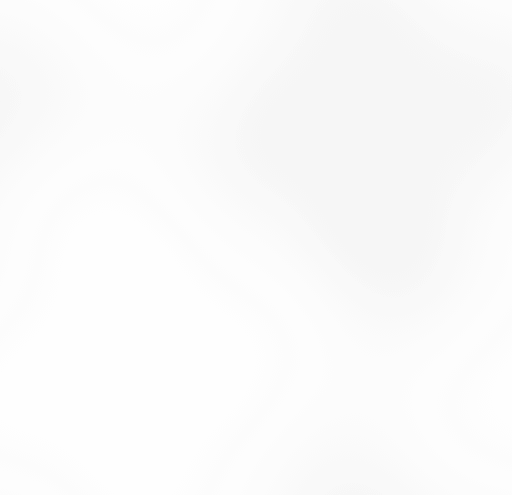
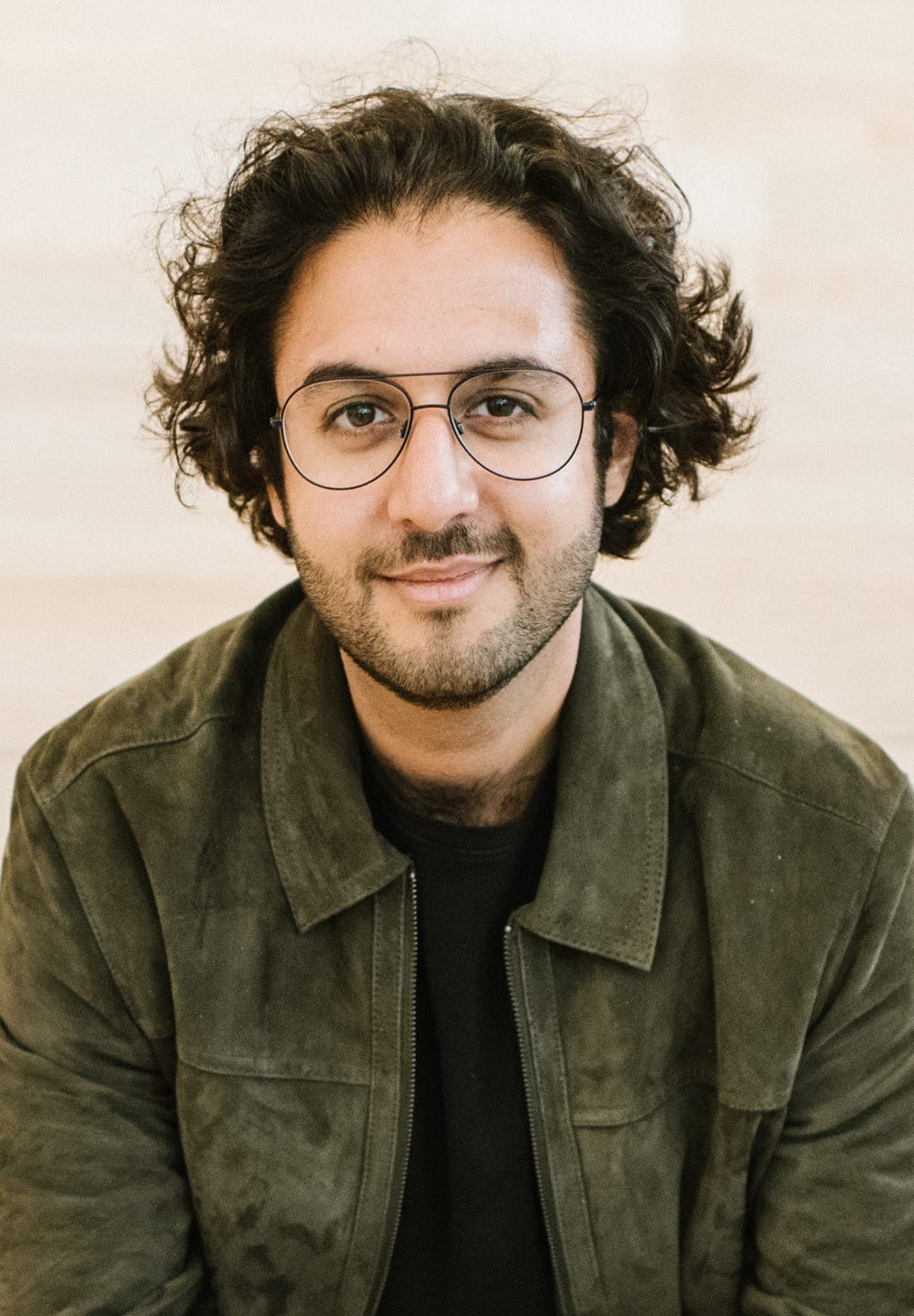