May
RAG with linked template tools
May 22nd, 2025
You can now call linked tools in your prompt templates. This allows you to combine your Tools, Prompts and Agents to build more complex workflows. For example, by calling a linked Python code Tool within a Prompt’s template, you can build a powerful RAG system.
To start building with this feature, link a tool to your Prompt or Agent in the Editor.
This can be a Python code Tool, or even another Prompt or Agent.
Then, reference the tool in your prompt template using the {{tool_name()}}
syntax.
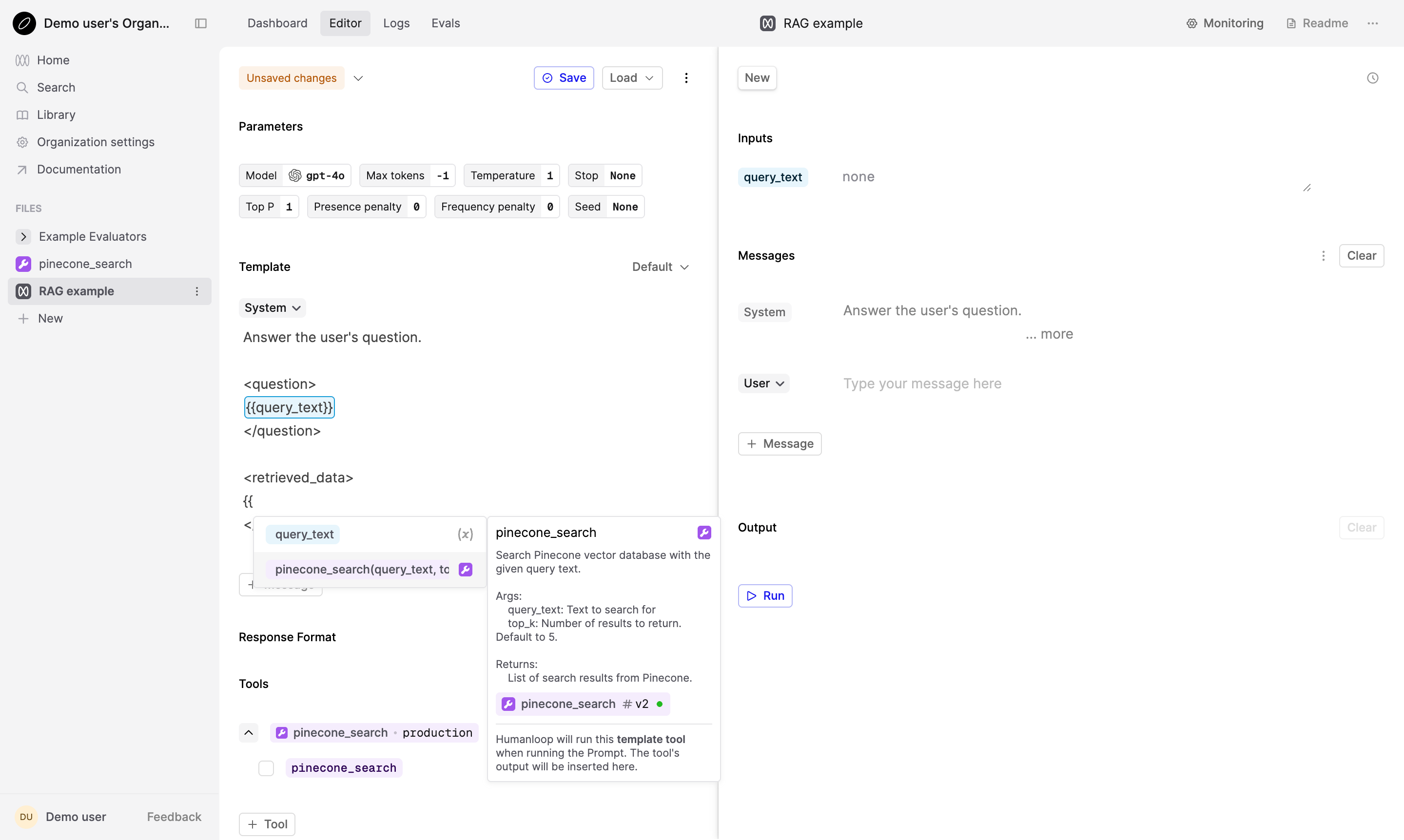
Referencing a pinecone_search
tool in a prompt template
When you run this Prompt or Agent, Humanloop will automatically run the tool and insert its output into the template at runtime. The tool’s output is displayed in the Log drawer, allowing you to view the tool’s output and what was passed to the Prompt or Agent.
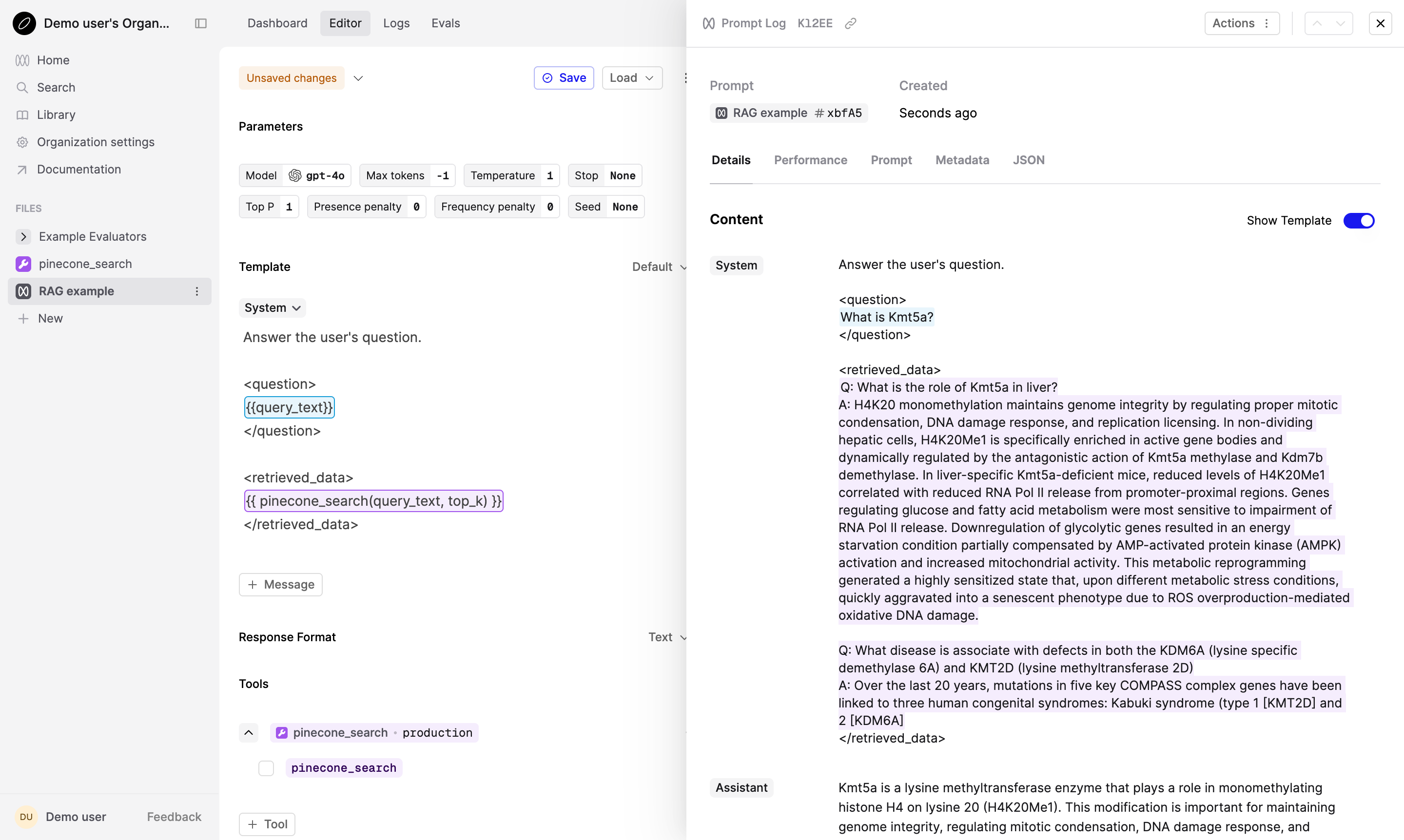
Support for Claude 4 models
May 22nd, 2025
Anthropic just launched Claude 4, and we’ve added support for Claude 4 Sonnet and Opus. Try them out in the Editor now!
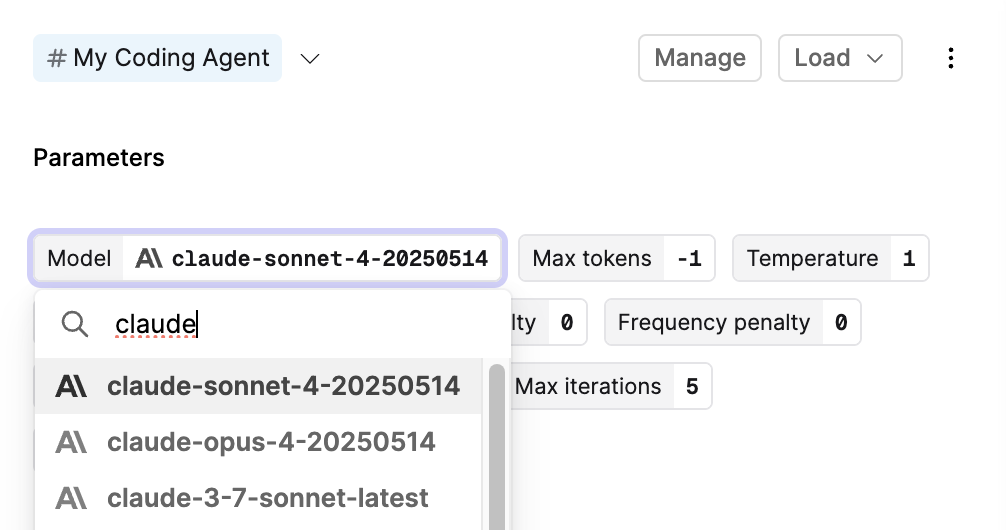
Cross-Region inference support for AWS Bedrock
May 20th, 2025
We’ve added support for cross-Region inference for AWS Bedrock models.
Cross-Region inference automatically selects the optimal AWS Region within your geographic area to process inference requests. This ensures higher availability and improved performance by dynamically routing traffic to the most responsive region.
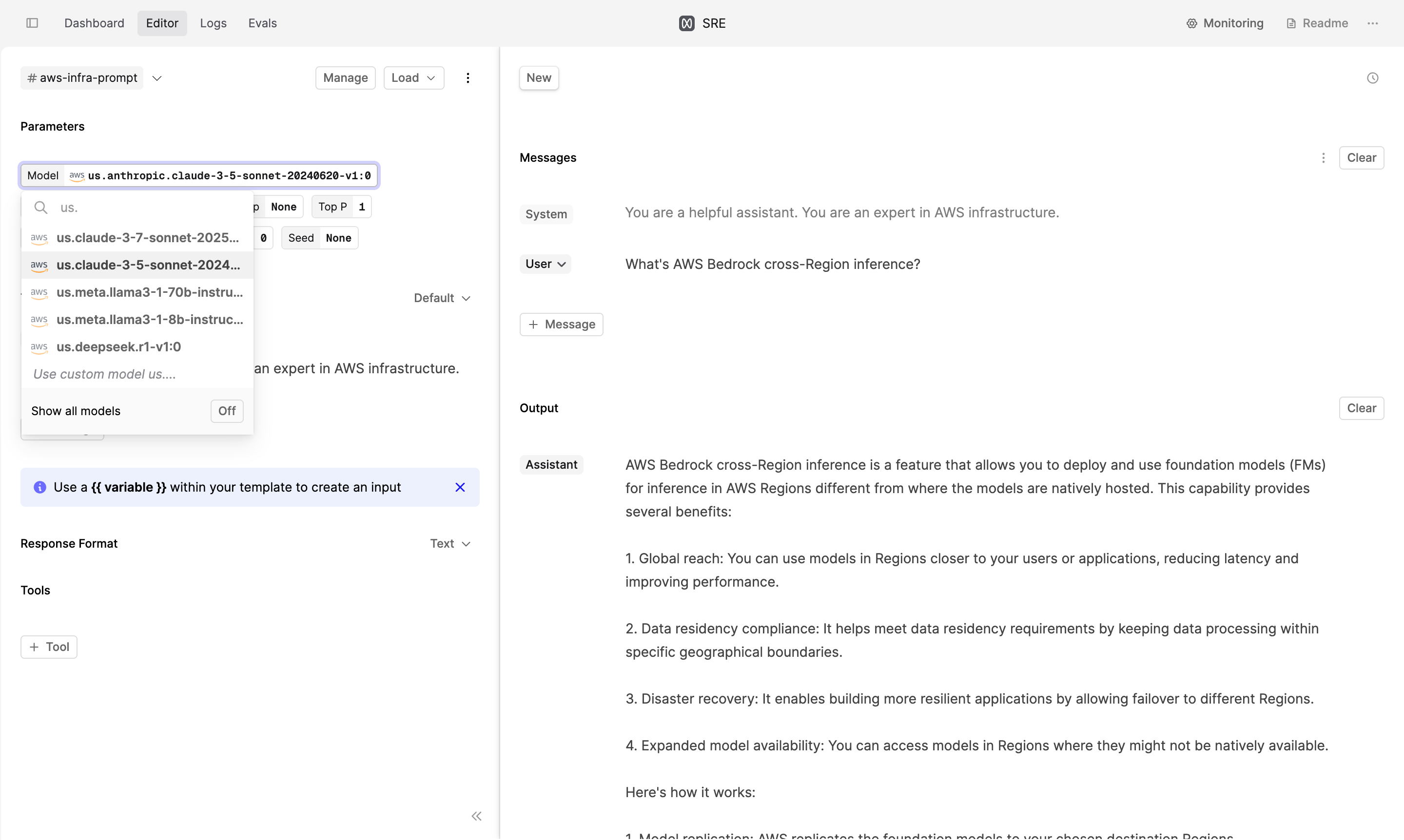
How to use:
- In the Prompt Editor: Choose a model prefixed with us. or eu. (e.g.,
us.anthropic.claude-3-5-sonnet-20240620-v1:0
) from the model selection dropdown. - In the SDK: Set the
provider
field tobedrock
, and use a model name prefixed withus.
oreu.
(e.g.,us.anthropic.claude-3-5-sonnet-20240620-v1:0
) in themodel
field.
The us.
prefix is used for models deployed in the US geographic area, while eu.
is for models deployed in the EU geographic area. Note that not all models support cross-Region inference.
See the the full list of supported models in AWS documentation.
To find out more about cross-Region inference, see the AWS documentation.
Store Prompts in code
May 19th, 2025
We’ve added support for storing Prompt and Agent Files in your codebase, allowing you to maintain them alongside your application code.
Local environment after running the humanloop pull
command
This release introduces a workflow to sync Prompts and Agents between Humanloop and your codebase:
Pull Files to your local environment using the CLI command humanloop pull
,
available in our Python and TypeScript SDKs (TypeScript support coming soon).
Reference Files from your local filesystem by initializing the SDK with use_local_files=True
,
then using the familiar path
parameter to point to local Files instead of remote ones.
This feature bridges the gap between Humanloop’s collaborative environment and developer workflows, enabling teams to use familiar Git-based processes while maintaining the benefits of centralized Prompt and Agent management.
To get started, check out our guide on storing Prompts in code.
More Intuitive Observability
May 16th, 2025
Previously, Evaluators (monitoring or those in Evaluation Runs) would be applied to a Log once at creation time.
Flow Logs were an exception to this rule: they are created upfront and used to trace other Logs created by your AI system. So you’d have to mark the Flow Log as complete
, letting Humanloop know no more Logs would be added to its trace.
However, some usecases don’t fit this workflow. For example, in an issue tracking system, a user may re-open an issue and continue the conversation, making it difficult to determine when the conversation is complete.
We’ve done away with the evaluate-once approach: Now, every time you modify a Log, Evaluators will be applied on it again. In the case of Flow Logs, every time you add a new Log to its trace, we re-apply the Evaluators and re-compute metrics such as latency.
Marking Flow Logs as complete
is no longer required for evaluation, but completing one will still prevent the trace from being modified.
We believe the new approach makes for a more intuitive experience: observability should always reflect the latest state of the observed system.
Link Files to Agents by environment
May 10th, 2025
You can now link tools to Agents by environment instead of by version. This allows an Agent to automatically use the latest deployed version of another Agent, Prompt, or Tool, ensuring the Agent’s tools remain up-to-date with your workspace changes.
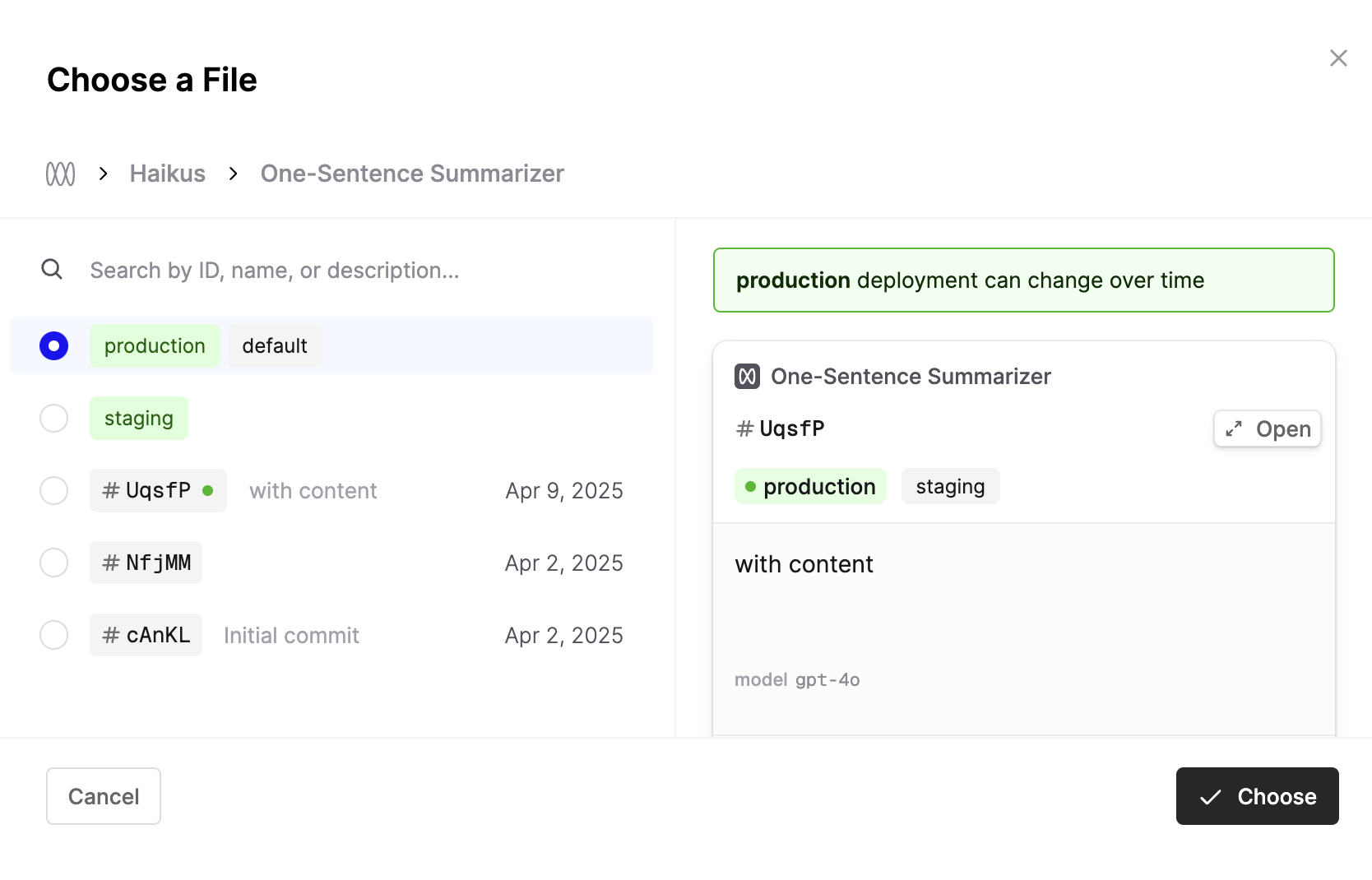
Trace Logs in the Review Tab
May 9th, 2025
You can now select trace Logs within the Review tab when evaluating a Flow or Agent.
This helps you dive deeper into each Log within the Trace, making it easier to understand how each step contributes to the final output.
To try it out, run an Agent or Flow Evaluation and open the Review tab.
Nested Agents
May 8th, 2025
Agents can now call other Agents as tools. This allows you to build more complex multi-step agentic workflows. To try it out, simply link the desired Agent file and version as a tool in the main Agent.
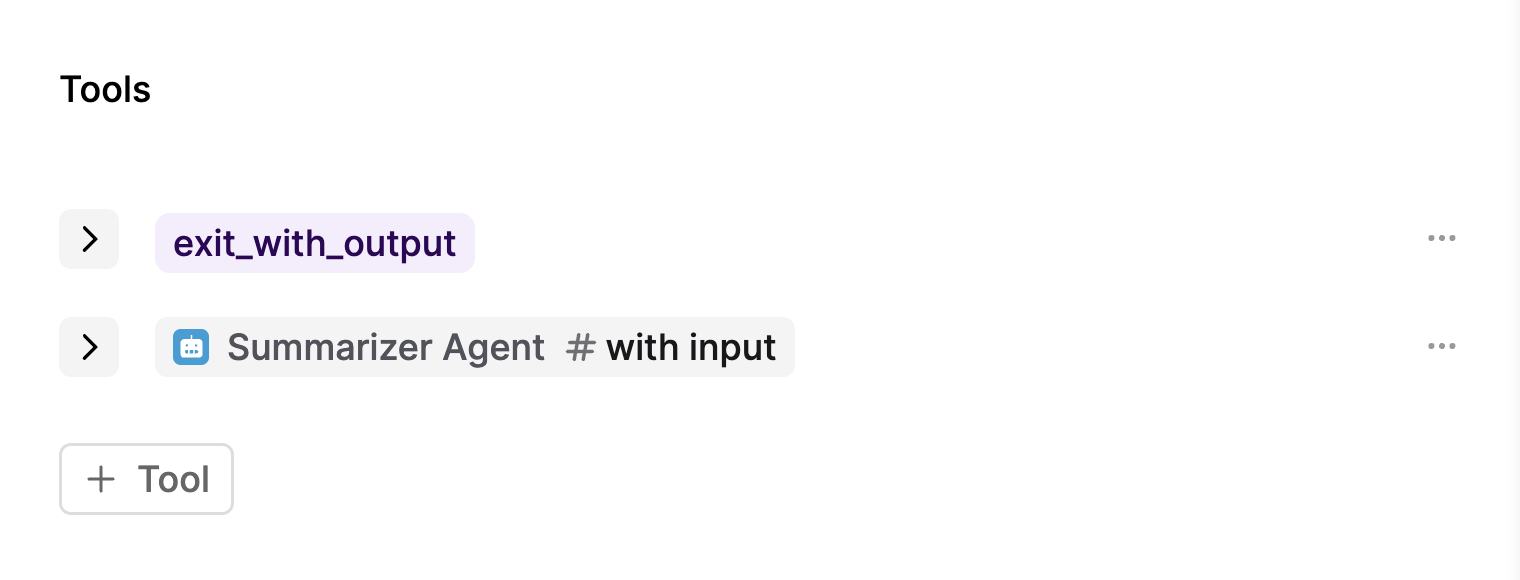
Logs generated by the nested Agent will be captured in the main Agent’s trace.
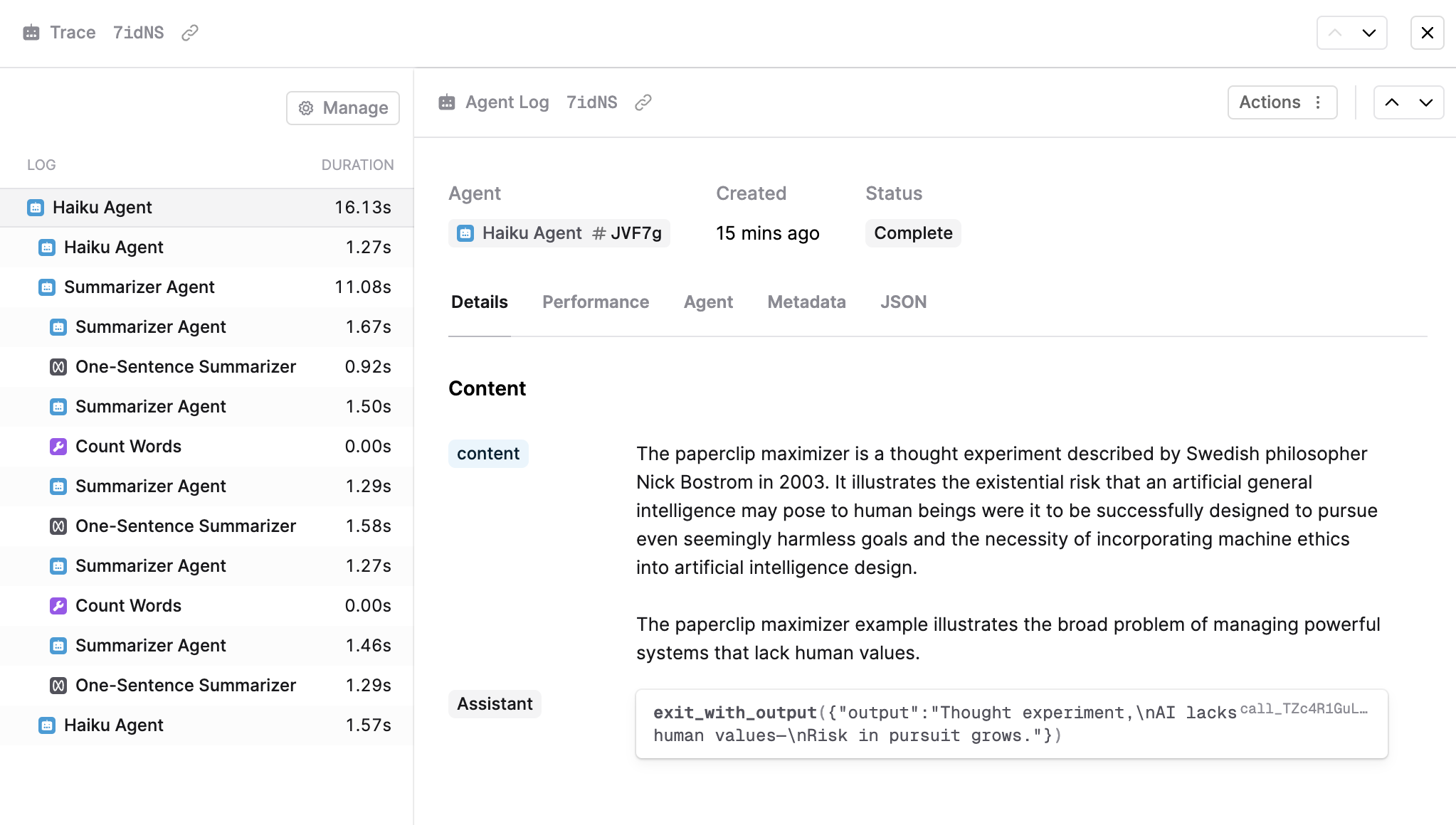
For an in-depth introduction on Agents, see our Key Concepts documentation. To get started with Agents, follow our Agents quickstart guide.
Bedrock support for DeepSeek
May 2nd, 2025
We’ve added support for the DeepSeek R1 model through our AWS Bedrock integration.
DeepSeek R1 model is now available as a cost-effective, high-performance alternative to proprietary reasoning models like Claude 3.7 or OpenAI’s 03-mini. To try it out, select the DeepSeek R1 model from the model selection dropdown in the Prompt Editor.