Set up semantic search (RAG)
In this guide we will set up a Humanloop Pinecone tool and use it to enrich a prompt with the relevant context from a data source of documents. This tool combines Pinecone’s semantic search with OpenAI’s embedding models.
Prerequisites
- A Humanloop account - you can create one by going to our sign up page.
- A Pinecone account - you can create one by going to their sign up page.
- Python installed - you can download and install Python by following the steps on the Python download page.
If you have an existing Pinecone index that was created using one of OpenAI’s embedding models, you can skip to section: Setup Humanloop
Set up Pinecone
Install the Pinecone SDK
If you already have the Pinecone SDK installed, skip to the next section.
Go to the Pinecone console API Keys tab and create an API key - copy the key value
and the environment
.
Create a Pinecone index
Now we’ll initialise a Pinecone index, which is where we’ll store our vector embeddings. We will be using OpenAI’s ada model to create vectors to save to Pinecone, which has an output dimension of 1536 that we need to specify upfront when creating the index:
Preprocess the data
Now that you have a Pinecone index, we need some data to put in it. In this section we’ll pre-process some data ready for embedding and storing to the index in the next section.
We’ll use the awesome Hugging Face datasets to source a demo dataset (following the Pinecone quick-start guide). In practice you will customise this step to your own use case.
Populate Pinecone
Now that you have a Pinecone index and a dataset of text chunks, we can populate the index with embeddings before moving on to Humanloop. We’ll use one of OpenAI’s embedding models to create the vectors for storage.
Install and initialise Open AI SDK
If you already have your OpenAI key and the SDK installed, skip to the next section.
Populate the index
If you already have a Pinecone index set up, skip to the next section.
Set up Humanloop
Configure Pinecone
You’re now ready to configure a Pinecone tool in Humanloop:
Configure Pinecone and OpenAI
These should be the same values you used when setting up your Pinecone index in the previous sections. All these values are editable later.
- For Pinecone: populate values for
Name
(use quora_search),pinecone_key
,pinecone_environment
,pinecone_index
(note: we named our indexhumanloop-demo
). The name will be used to create the signature for the tool that you will use in your prompt templates in the next section. - For OpenAI: populate the
openai_key
andopenai_model
(note: we used thetext-embedding-ada-002
model above)
An active tool for quora_search will now appear on the tools tab and you’re ready to use it within a prompt template.
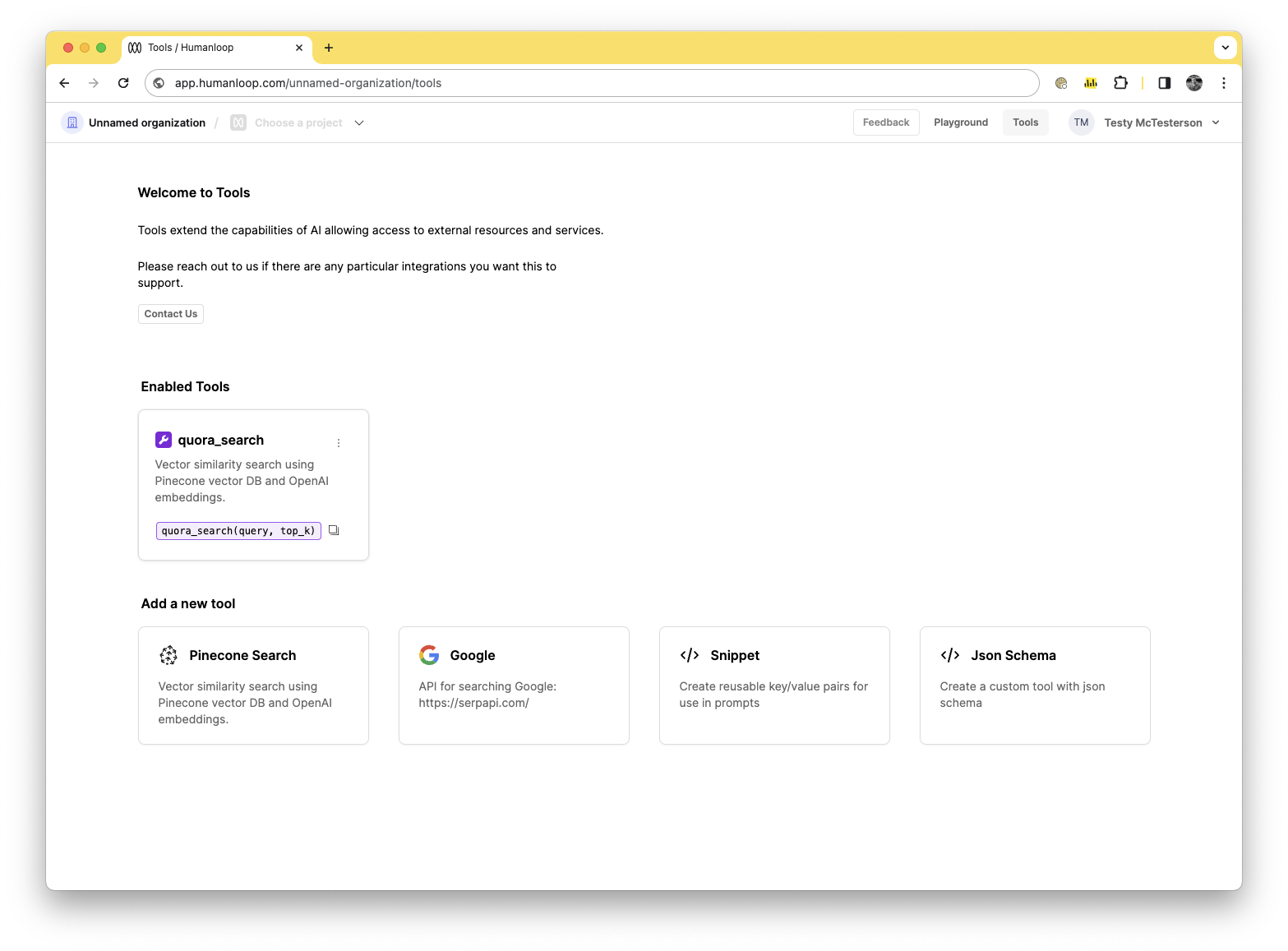
Enhance your Prompt template
Now that we have a Pinecone tool configured we can use this to pull relevant context into your prompts.
This is an effective way to enrich your LLM applications with knowledge from your own internal documents and also help fix hallucinations.
On the right hand side under Completions, enter the following three examples of topics: Google, Physics and Exercise.
Press the Run all button bottom right (or use the keyboard shortcut Command + Enter
).
On the right hand side the results from calling the Pinecone tool for the specific topic will be shown highlighted in purple and the final summary provided by the LLM that uses these results will be highlighted in green.
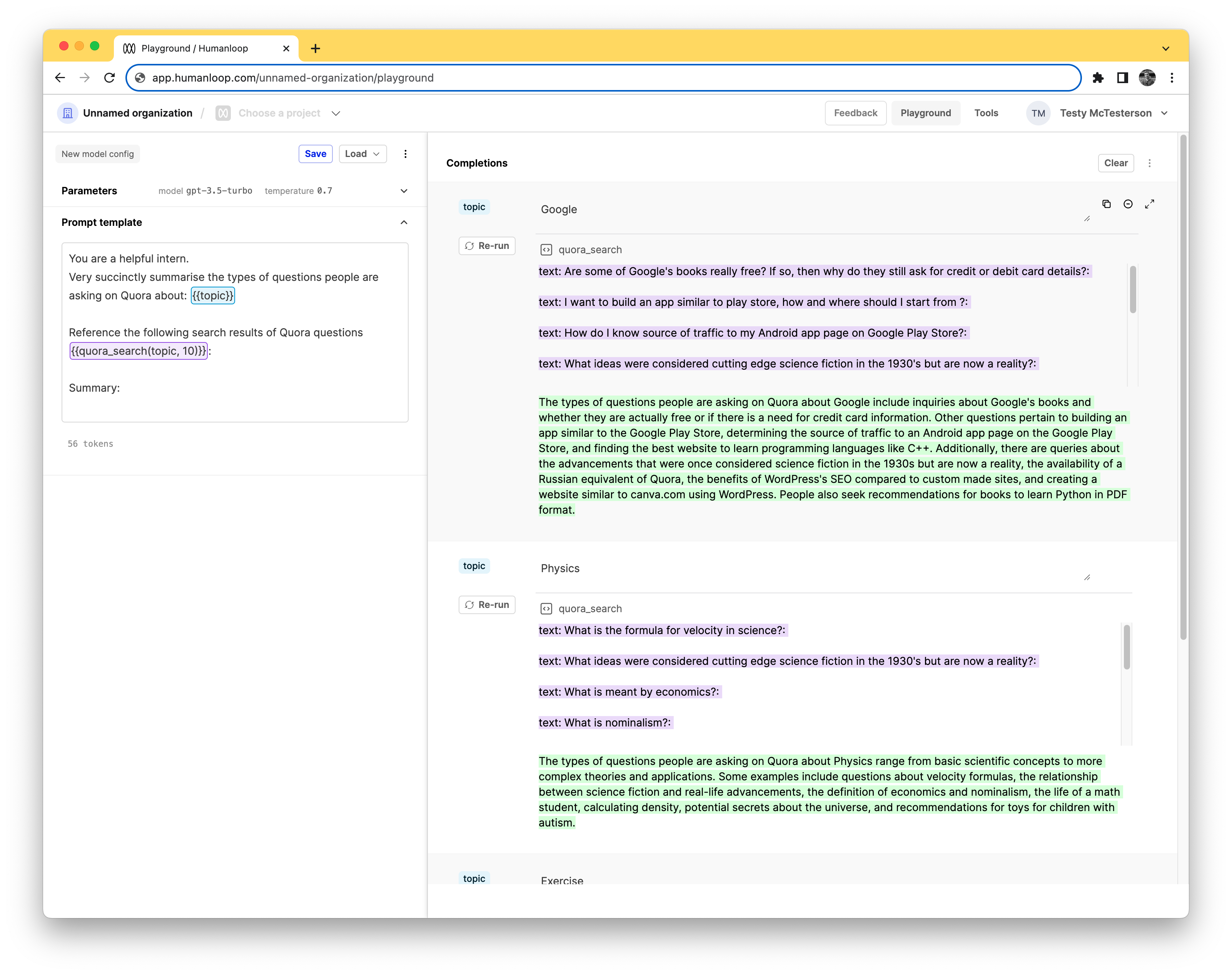
Using tools in the prompt template
Each active tool in your organisation will have a unique signature that you can use to specify the tool within a prompt template.
You can find the signature in the pink box on each tool card on the Tools page.
You can also use double curly brackets - {{
- within the prompt template in the Prompt Editor to see a dropdown of available tools.
In the case of Pinecone tools, the signature takes two positional arguments: query
(the query text passed to Pinecone) and top_k
(the number of similar chunks to retrieve from Pinecone for the query).
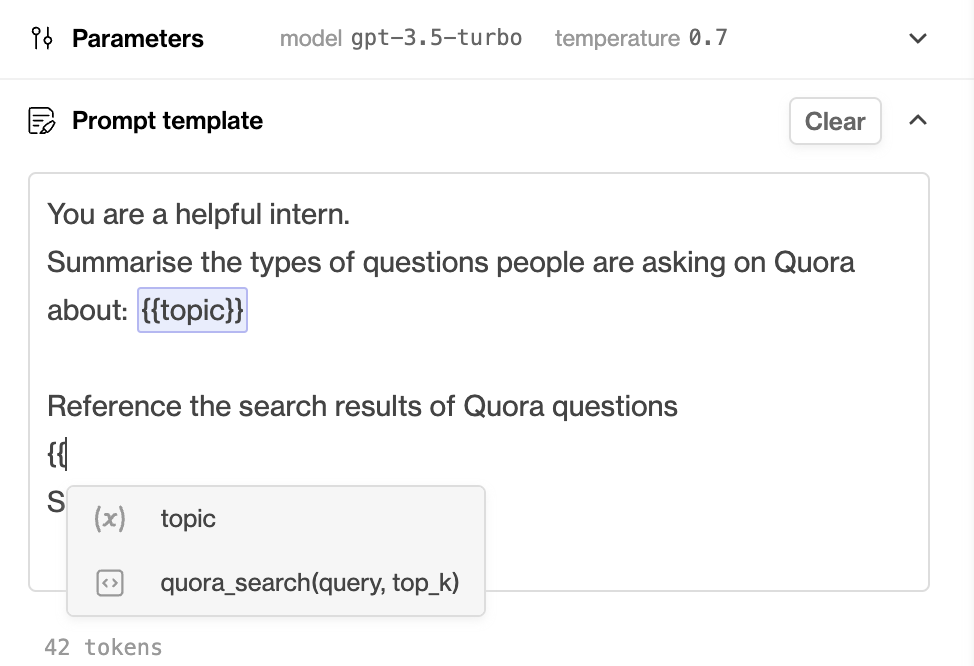